Cross-Modal Diversity-Based Active Learning for Multi-Modal Emotion Estimation.
IJCNN(2023)
摘要
Emotion recognition is an important part of affective computing. Utilizing information from multiple modalities would facilitate more accurate emotion recognition. The performance of data-driven machine learning models usually relies on a large amount of labeled training data. However, labeling emotional data is expensive, because each sample usually requires multiple evaluators to annotate. To alleviate the annotation cost, this paper proposes a cross-modal diversity measure that considers the correlation between different modalities and integrates it with the representativeness for sample selection in unsupervised active learning (AL) for regression. To our knowledge, this challenging multi-modal unsupervised AL scenario has not been explored before: previous research only considered either unsupervised uni-modal AL or supervised multi-modal AL. Experiments on RECOLA and IEMOCAP datasets demonstrated the effectiveness of our proposed AL approach.
更多查看译文
关键词
Active learning,unsupervised learning,multi-modal learning,emotion recognition
AI 理解论文
溯源树
样例
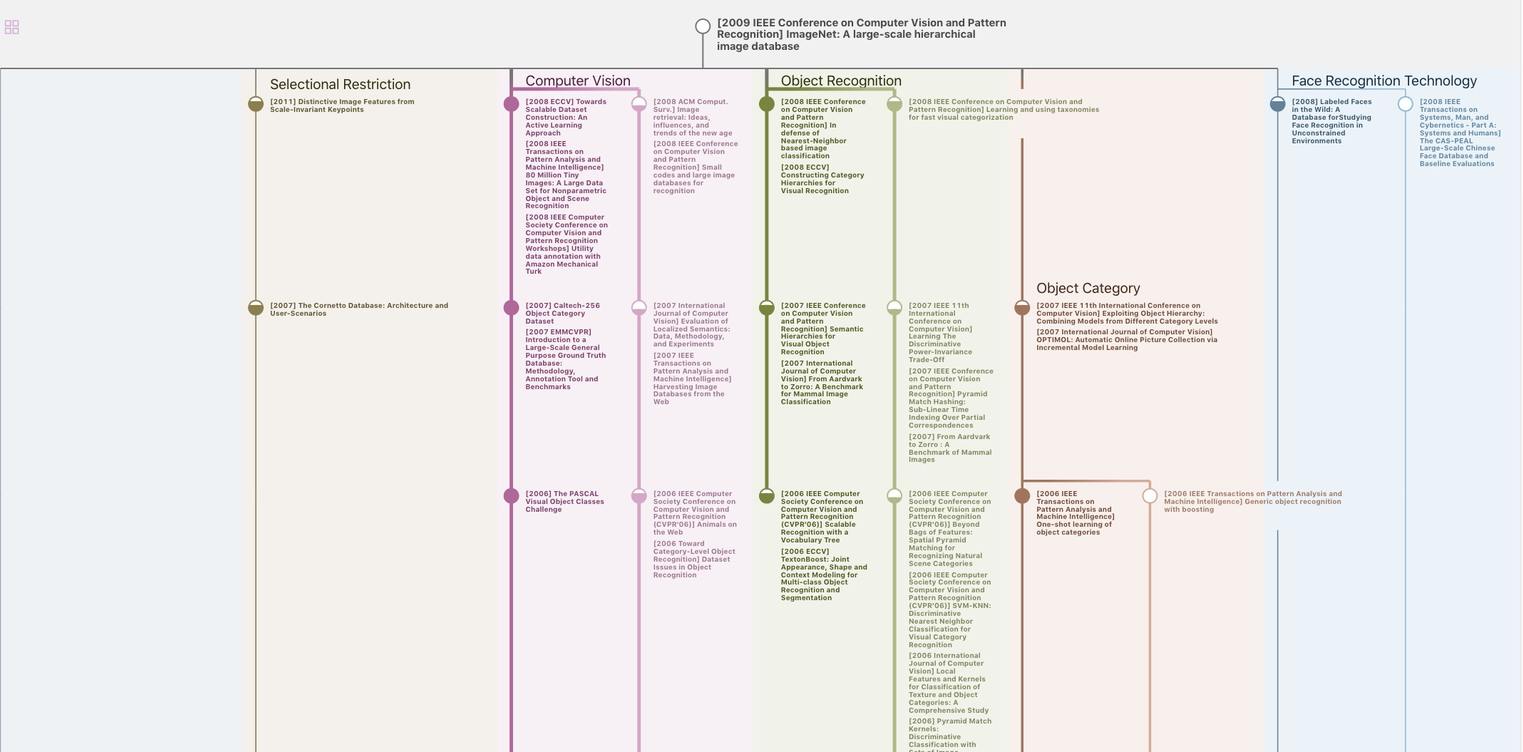
生成溯源树,研究论文发展脉络
Chat Paper
正在生成论文摘要