Heterogeneous Graph Interaction based Event Extraction with Attentional Position Embeddings.
IJCNN(2023)
摘要
Document-level event extraction has become one of the important research directions in natural language processing, which aims to extract the complete event arguments from the whole document. However, existing models cannot fully utilize contextual semantic relations and sequential information in complex document-level scenarios, failing to mine the relational facts between multiple sentences and arguments. To address the above problems, we propose a heterogeneous graph interaction event extraction model fusing positional embedding and attention matrix called GPAIT. Firstly, contextual semantic relationships are enhanced between entities by constructing entity attention relationship matrix; Secondly, the attention matrix is combined to rectify the representation of heterogeneous graphs; Finally, positional embedding is fused into graphs to build a graph convolutional neural network that can better capture semantic sequential relationships. Experiments on ChFinAnn and COVID-19 News show that GPAIT outperforms other document-level event extraction methods in terms of F1 by 1.2% and 3.6% respectively.
更多查看译文
关键词
attention matrix,attentional position embeddings,complete event arguments,complex document-level scenarios,contextual semantic relations,contextual semantic relationships,document-level event extraction methods,entity attention relationship matrix,graph convolutional neural network,heterogeneous graph interaction event extraction model,heterogeneous graphs,important research directions,multiple sentences,natural language processing,positional embedding,relational facts,semantic sequential relationships,sequential information
AI 理解论文
溯源树
样例
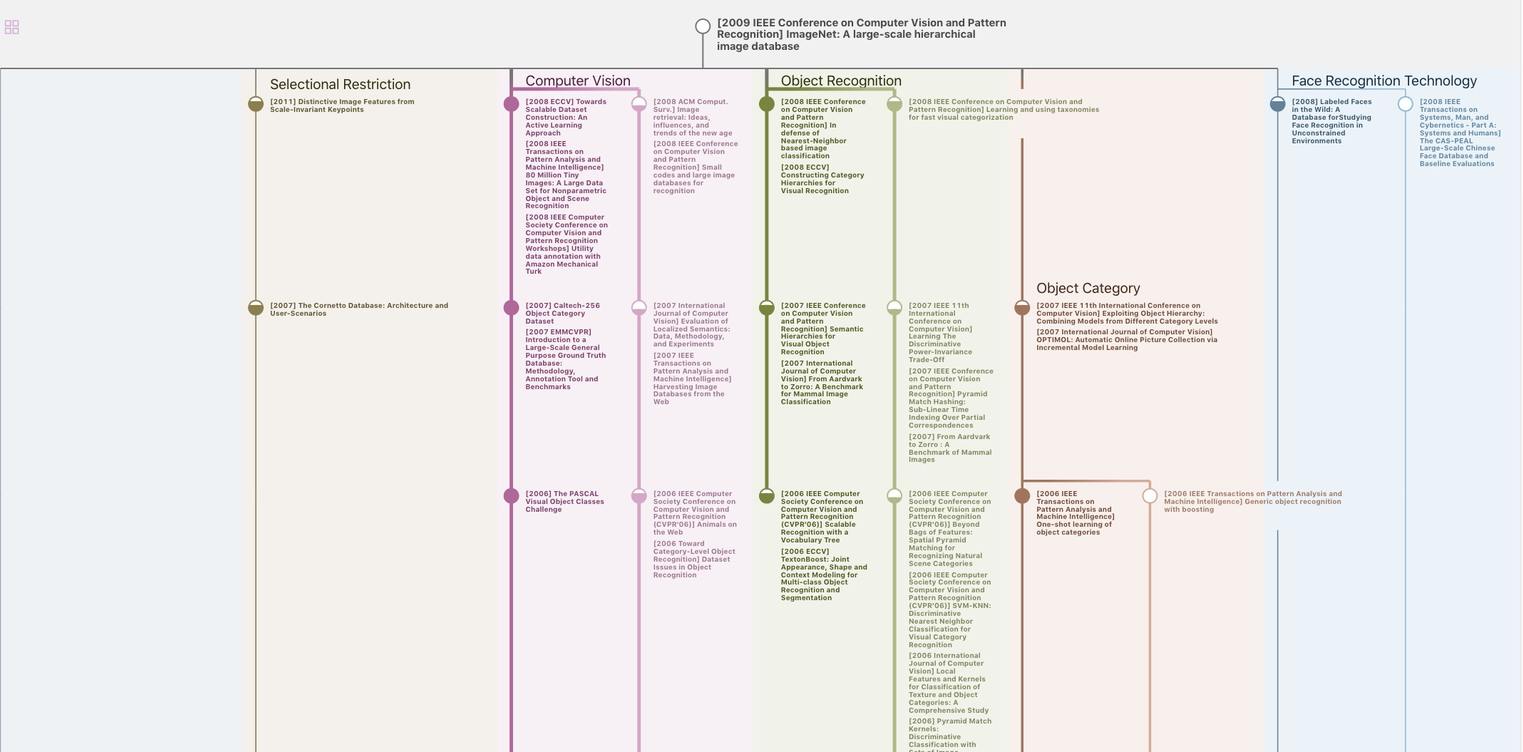
生成溯源树,研究论文发展脉络
Chat Paper
正在生成论文摘要