OB-ConvLSTM: A sequential remote sensing crop classification model with OBIA and ConvLSTM models
2023 11th International Conference on Agro-Geoinformatics (Agro-Geoinformatics)(2023)
摘要
Remote sensing technology has become increasingly important in recent years due to its ability to collect high-resolution images of agricultural fields. One of the most popular methods for crop classification in agricultural fields is object-based image analysis (OBIA). At the same time, the convolutional long short-term memory (ConvLSTM) network has shown great potential in processing spatiotemporal data.In this study, we proposed a new model called OB-ConvLSTM (Object-based ConvLSTM) that combines OBIA and ConvLSTM for spatiotemporal crop classification tasks. This model extracts crop spectral information from the spatial dimension of remote sensing images, extracts crop growth information from multiple remote sensing images in the temporal dimension, and synthesizes the spatial and temporal dimension information to improve crop classification accuracy. Compared with traditional crop classification models based on single temporal remote sensing, the model proposed in this study is superior to existing models in classification accuracy and model robustness. The proposed OB-ConvLSTM model has been applied to crop classification tasks in major crop-producing regions, achieving over 93% of the crop species recognition accuracy, with mIoU reaching 83%.The main contribution of this study is to design a temporal remote sensing image semantic segmentation model structure suitable for field crop classification, combining the OBIA method with ConvLSTM and improving the model’s performance by optimizing model components such as activation functions and optimizers. Specifically, there are several innovations in the following aspects: First, to facilitate model input, this study uses the SLIC algorithm to segment remote sensing images into uniformly sized superpixel objects and aligns the superpixel objects in the temporal dimension; Subsequently, we used the ConvLSTM model totrain and classify superpixel objects with temporal information, and adopted the Mish activation function further to improve the semantic segmentation accuracy of remote sensing images. The dataset used in the experiment is temporal remote sensing images of corresponding ground crop types, including soybean and corn crops.Our experimental results demonstrate that the proposed method achieved an overall classification accuracy of 93%, with a mean intersection-over-union (mIoU) of 83%. This model achieves higher accuracy than existing methods in field crop classification tasks. Future research can further expand the application of this model in crop yield prediction, crop management, and agricultural decision-making.
更多查看译文
关键词
sequential remote sensing,object-based image analysis,crop classification,long short-term memory
AI 理解论文
溯源树
样例
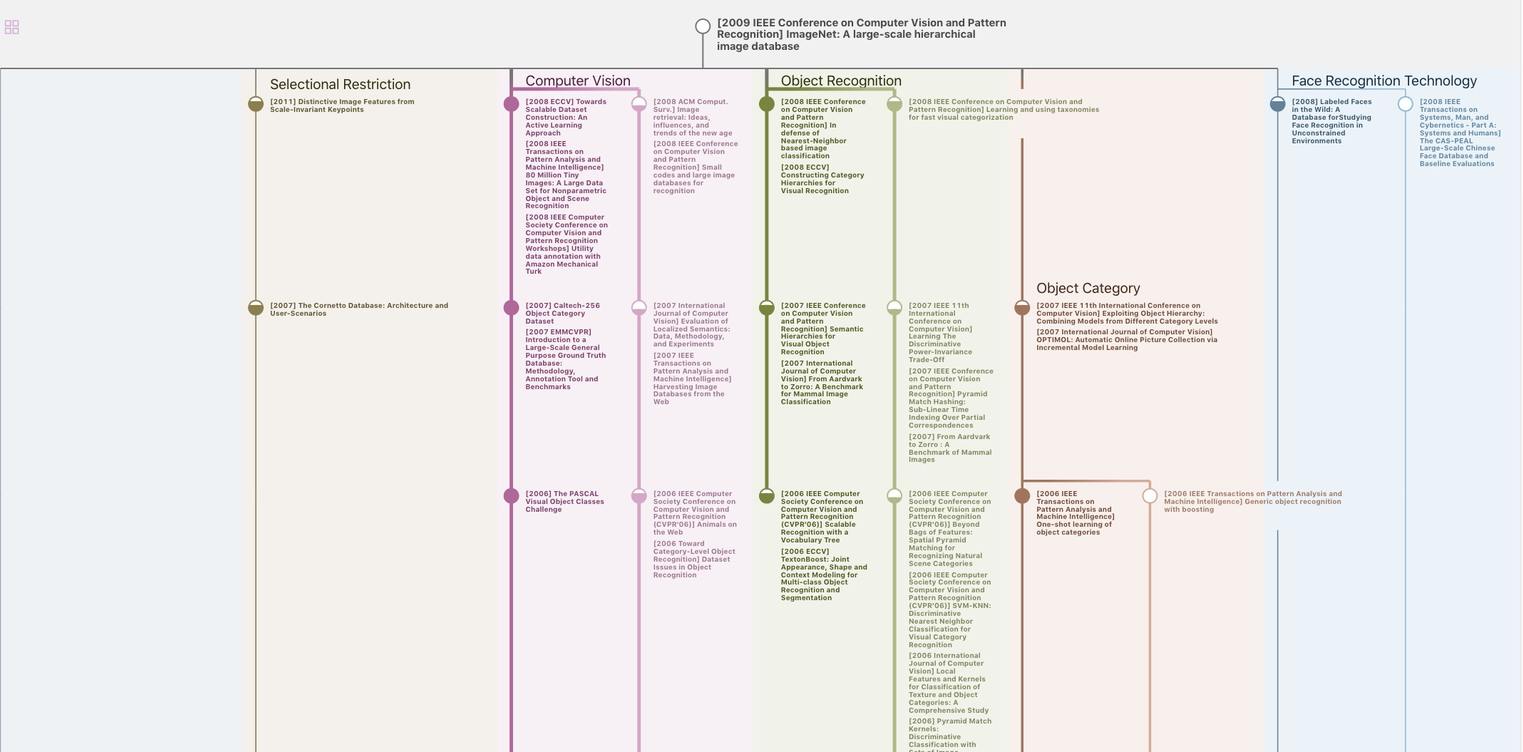
生成溯源树,研究论文发展脉络
Chat Paper
正在生成论文摘要