Decentralized Data-Privacy Preserving Deep-Learning Approaches for Enhancing Inter-Database Generalization in Automatic Sleep Staging
IEEE JOURNAL OF BIOMEDICAL AND HEALTH INFORMATICS(2023)
摘要
Automatic sleep staging has been an active field of development. Despite multiple efforts, the area remains a focus of research interest. Indeed, while promising results have reported in past literature, uptake of automatic sleep scoring in the clinical setting remains low. One of the current issues regards the difficulty to generalization performance results beyond the local testing scenario, i.e. across data from different clinics. Issues derived from data-privacy restrictions, that generally apply in the medical domain, pose additional difficulties in the successful development of these methods. We propose the use of several decentralized deep-learning approaches, namely ensemble models and federated learning, for robust inter-database performance generalization and data-privacy preservation in automatic sleep staging scenario. Specifically, we explore four ensemble combination strategies (max-voting, output averaging, size-proportional weighting, and Nelder-Mead) and present a new federated learning algorithm, socalled sub-sampled federated stochastic gradient descent (ssFedSGD). To evaluate generalization capabilities of such approaches, experimental procedures are carried out using a leaving-one-database-out direct-transfer scenario on six independent and heterogeneous public sleep staging databases. The resulting performance is compared with respect to two baseline approaches involving single-database and centralized multiple-database derived models. Our results show that proposed decentralized learning methods outperform baseline local approaches, and provide similar generalization results to centralized database-combined approaches. We conclude that these methods are more preferable choices, as they come with additional advantages concerning improved scalability, flexible design, and data-privacy preservation.
更多查看译文
关键词
Data-privacy,deep-learning,domain adaption,ensemble models,federated learning,inter-database generalization,sleep staging
AI 理解论文
溯源树
样例
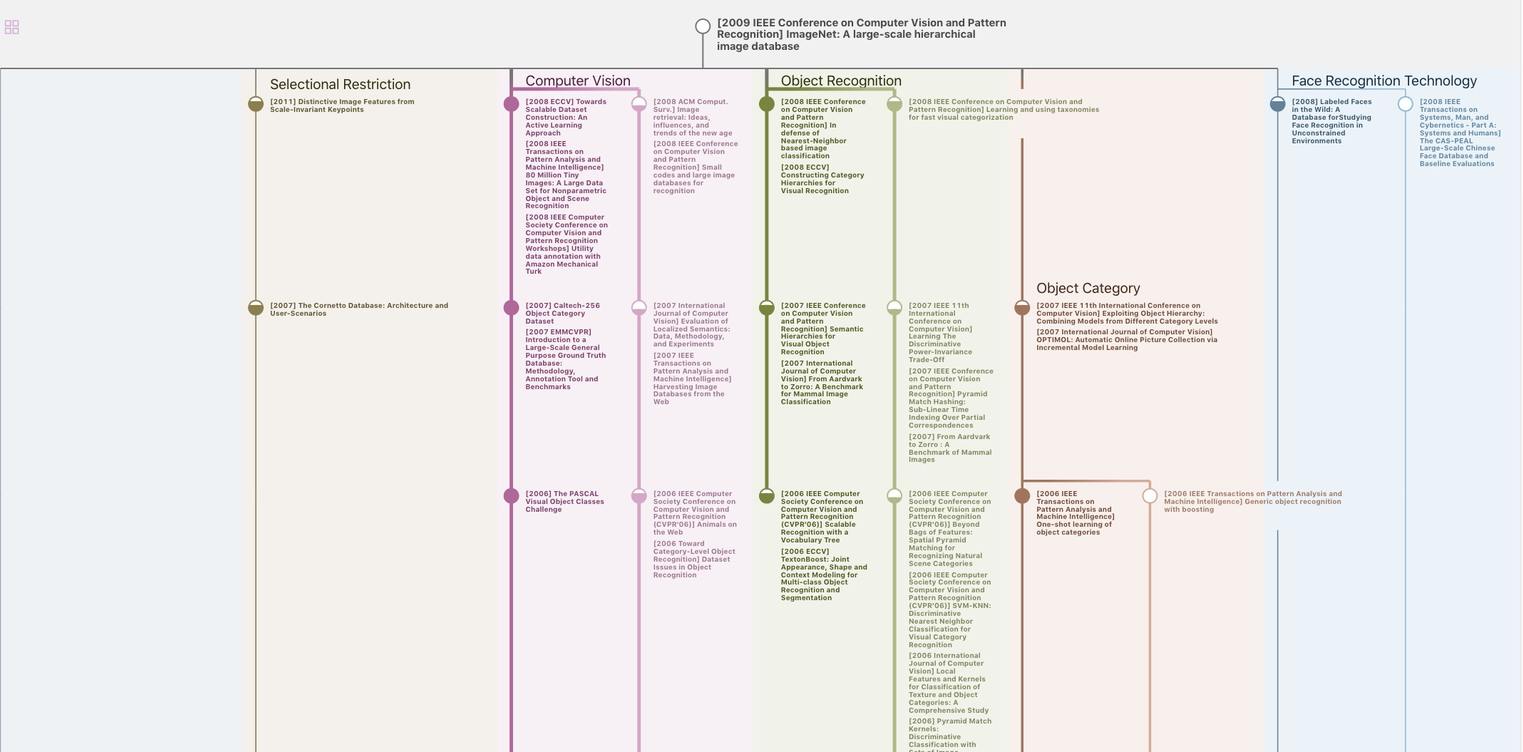
生成溯源树,研究论文发展脉络
Chat Paper
正在生成论文摘要