$\ell _{2,p}$ -Norm and Mahalanobis Distance-Based Robust Fuzzy C-Means
IEEE Transactions on Fuzzy Systems(2023)
摘要
Fuzzy C-means (FCM) is a kind of classic cluster method, which has been widely used in various fields, such as image segmentation and data mining. Euclidean distance is a frequently used distance metric in FCM, but it is only suitable for data with spherical structure. As an extension of Euclidean distance, Mahalanobis distance has been used in Gustafson–Kessel FCM and its variants to tackle ellipsoidal data. For the convenience of optimizing, most existing Mahalanobis distance-based FCM algorithms only focus on squared Mahalanobis distance. However, squared Mahalanobis distance may not be the best distance metric for FCM because it is easy to enlarge the influence of outliers. In this article, we propose a novel
$\ell _{2,p}$
-norm and Mahalanobis distance-based FCM model, abbreviated as LM-FCM, which can help FCM improve the ability of tackling ellipsoidal clusters and outliers. Then, in order to reduce computational complexity, we propose a more simplified model, abbreviated as SLM-FCM. Furthermore, we develop an iteratively reweighted optimization algorithm to optimize the proposed models and provide a rigorous monotonous convergence proof. Finally, compared with the existing state-of-the-art FCM algorithms, we conduct extensive experiments on both synthetic and real-world datasets to manifest the superior clustering performance and robustness of the proposed algorithms.
更多查看译文
关键词
distance-based,c-means
AI 理解论文
溯源树
样例
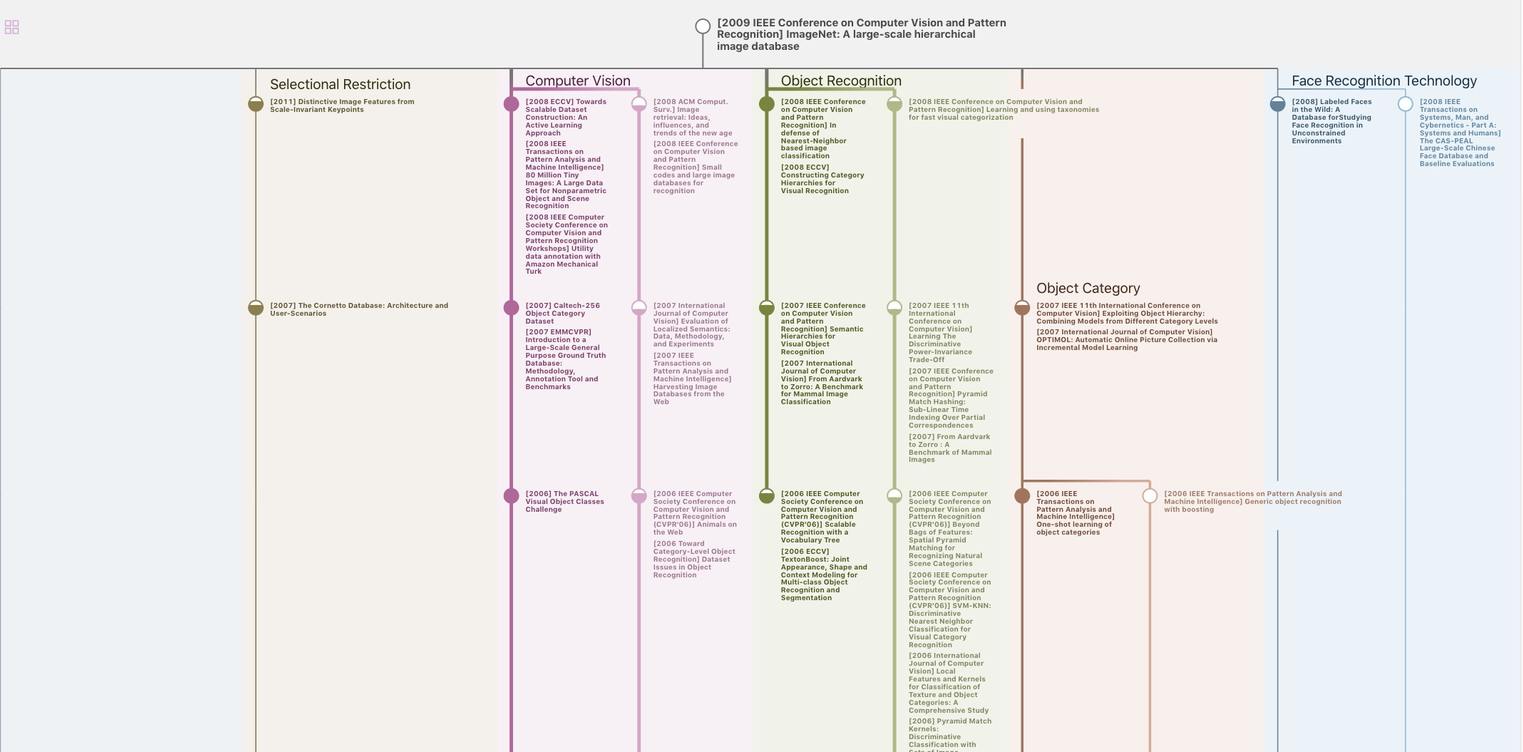
生成溯源树,研究论文发展脉络
Chat Paper
正在生成论文摘要