Automated segmentation of the mouse body language to study stimulus-evoked emotional behaviors.
eNeuro(2023)
摘要
Understanding the neural basis of emotions is a critical step to uncover the biological substrates of neuropsychiatric disorders. To study this aspect in freely behaving mice, neuroscientists have relied on the observation of ethologically relevant bodily cues to infer the affective content of the subject, both in neutral conditions or in response to a stimulus. The best example of that is the widespread assessment of freezing in experiments testing both conditioned and unconditioned fear responses. While robust and powerful, these approaches come at a cost: they are usually confined within selected time windows, accounting for only a limited portion of the complexity of emotional fluctuation. Moreover, they often rely on visual inspection and subjective judgement, resulting in inconsistency across experiments and questionable result interpretations. To overcome these limitations, novel tools are arising, fostering a new avenue in the study of the mouse naturalistic behavior. In this work we developed a computational tool (stimulus-evoked behavioral tracking in 3D for rodents; SEB3R) to automate and standardize an ethologically-driven observation of freely moving mice. Using a combination of machine learning-based behavioral tracking and unsupervised cluster analysis, we identified statistically meaningful postures that could be used for empirical inference on a sub-second scale. We validated the efficacy of this tool in a stimulus-driven test, the whisker nuisance task, where mice are challenged with a prolonged and invasive whisker stimulation, showing that identified postures can be reliably used as a proxy for stimulus-driven fearful and explorative behaviors.We combined novel technical advancements of system neuroscience with a traditional ethology-based behavioral observation to design a simple computational tool for behavior detection in freely-moving mice. Using machine learning-based behavioral tracking and unsupervised cluster analysis, we identified statistically meaningful postures on a sub-second scale. We validated this tool in the whisker nuisance task, where mice are challenged with a prolonged and invasive whisker stimulation, showing that identified postures can be used as a proxy for stimulus-driven fearful and explorative behaviors. With this tool we aim to automate, accelerate and standardize data collection across research laboratories, improving the quality and reproducibility of behavioral studies in mice.
更多查看译文
关键词
behavioral segmentation, DeepLabCut, mouse behavior, mouse body language, mouse emotion, whisker nuisance
AI 理解论文
溯源树
样例
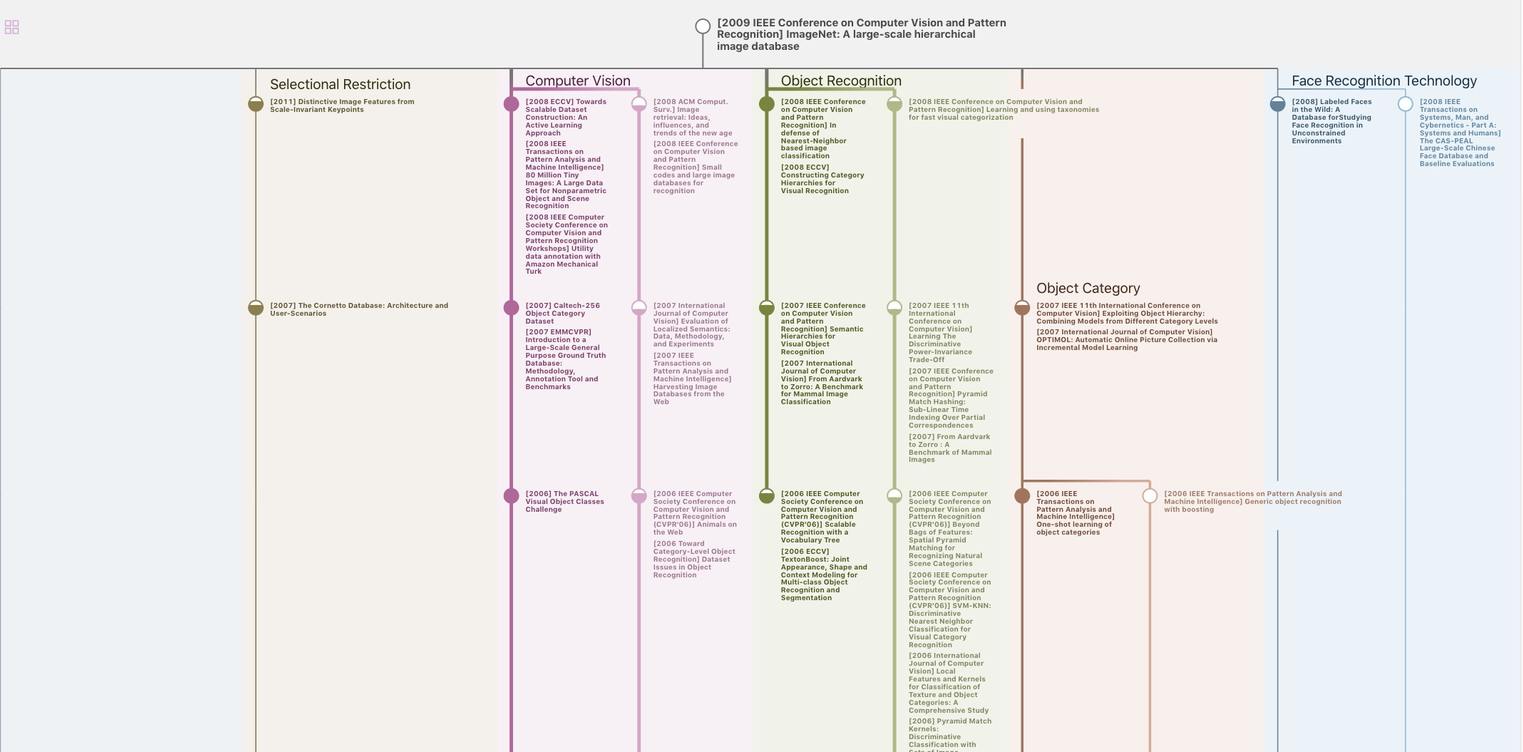
生成溯源树,研究论文发展脉络
Chat Paper
正在生成论文摘要