Effects of machine learning-based clinical decision support systems on decision-making, care delivery, and patient outcomes: a scoping review.
Journal of the American Medical Informatics Association : JAMIA(2023)
摘要
OBJECTIVE:This study aims to summarize the research literature evaluating machine learning (ML)-based clinical decision support (CDS) systems in healthcare settings.
MATERIALS AND METHODS:We conducted a review in accordance with the PRISMA-ScR (Preferred Reporting Items for Systematic Reviews and Meta Analyses extension for Scoping Review). Four databases, including PubMed, Medline, Embase, and Scopus were searched for studies published from January 2016 to April 2021 evaluating the use of ML-based CDS in clinical settings. We extracted the study design, care setting, clinical task, CDS task, and ML method. The level of CDS autonomy was examined using a previously published 3-level classification based on the division of clinical tasks between the clinician and CDS; effects on decision-making, care delivery, and patient outcomes were summarized.
RESULTS:Thirty-two studies evaluating the use of ML-based CDS in clinical settings were identified. All were undertaken in developed countries and largely in secondary and tertiary care settings. The most common clinical tasks supported by ML-based CDS were image recognition and interpretation (n = 12) and risk assessment (n = 9). The majority of studies examined assistive CDS (n = 23) which required clinicians to confirm or approve CDS recommendations for risk assessment in sepsis and for interpreting cancerous lesions in colonoscopy. Effects on decision-making, care delivery, and patient outcomes were mixed.
CONCLUSION:ML-based CDS are being evaluated in many clinical areas. There remain many opportunities to apply and evaluate effects of ML-based CDS on decision-making, care delivery, and patient outcomes, particularly in resource-constrained settings.
更多查看译文
AI 理解论文
溯源树
样例
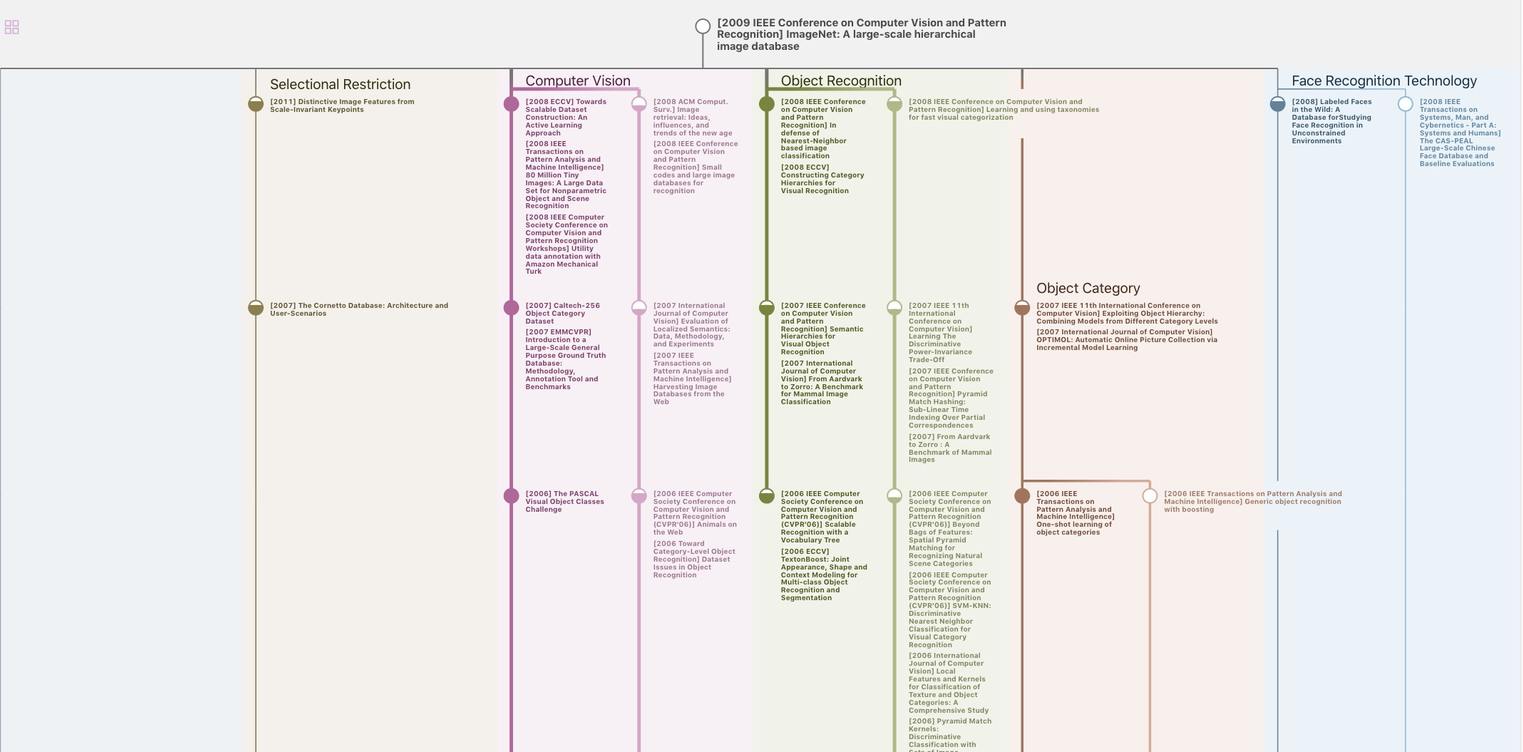
生成溯源树,研究论文发展脉络
Chat Paper
正在生成论文摘要