Combination of Rapid Intrinsic Activity Measurements and Machine Learning as a Screening Approach for Multicomponent Electrocatalysts.
ACS applied materials & interfaces(2023)
摘要
Machine learning (ML) coupled with quantum chemistry calculations predicts catalyst properties with high accuracy; however, ML approaches in the design of multicomponent catalysts primarily rely on simulation data because obtaining sufficient experimental data in a short time is difficult. Herein, we developed a rapid screening strategy involving nanodroplet-mediated electrodeposition using a carbon nanocorn electrode as the support substrate that enables complete data collection for training artificial intelligence networks in one week. The inert support substrate ensures intrinsic activity measurement and characterization of the irreversible reconstruction of multinary alloy particles during the oxygen evolution reaction. Our approach works as a closed loop: catalyst synthesis-in situ measurement and characterization-database construction-ML analysis-catalyst design. Using artificial neural networks, the ML analysis revealed that the entropy values of multicomponent catalysts are proportional to their catalytic activity. The catalytic activities of high-entropy systems with different components varied little, and the overall catalytic activity was greater than that of the medium-low-entropy system. These findings will serve as a guideline for the design of catalysts.
更多查看译文
关键词
rapid intrinsic activity measurements
AI 理解论文
溯源树
样例
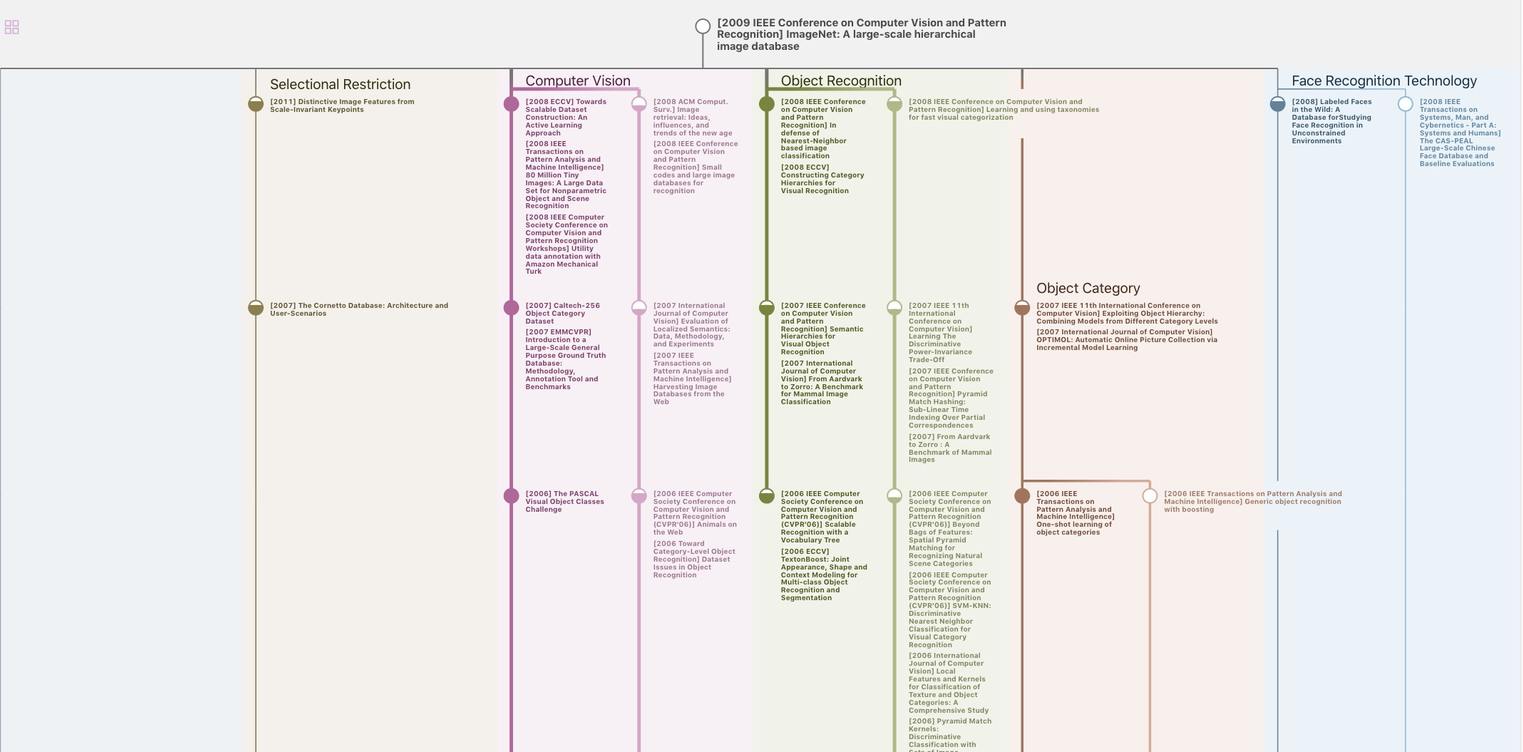
生成溯源树,研究论文发展脉络
Chat Paper
正在生成论文摘要