Quantifying acute kidney injury in an Ischaemia-Reperfusion Injury mouse model using Deep Learning-based semantic segmentation in histology.
Biology open(2023)
摘要
This study focuses on Ischaemia-Reperfusion Injury (IRI) in kidneys, a cause of acute kidney injury (AKI) and end-stage kidney disease (ESKD). Traditional kidney damage assessment methods are semi-quantitative and subjective. This study aims to use a Convolutional Neural Network (CNN) to segment murine kidney structures after IRI, quantify damage via CNN-generated pathological measurements, and compare this to conventional scoring. The CNN was able to accurately segment the different pathological classes, such as Intratubular Casts and Tubular Necrosis, with an F1 score of over 0.75. Some classes, such as Glomeruli and Proximal Tubules, had even higher statistical values with F1 scores over 0.90. The scoring generated based on the segmentation approach statistically correlated with the semiquantitative assessment (Spearman Correlation coefficient=0.94). The heatmap approach localised the intratubular necrosis mainly in the outer stripe of the outer medulla, while the tubular casts were also present in more superficial or deeper portions of the cortex and medullary areas. This study presents a CNN model capable of segmenting multiple classes of interest, including acute IRI-specific pathological changes, in a whole mouse kidney section and can provide insights into the distribution of pathological classes within the whole mouse kidney section.
更多查看译文
关键词
kidney injury,acute kidney injury,ischaemia-reperfusion,learning-based
AI 理解论文
溯源树
样例
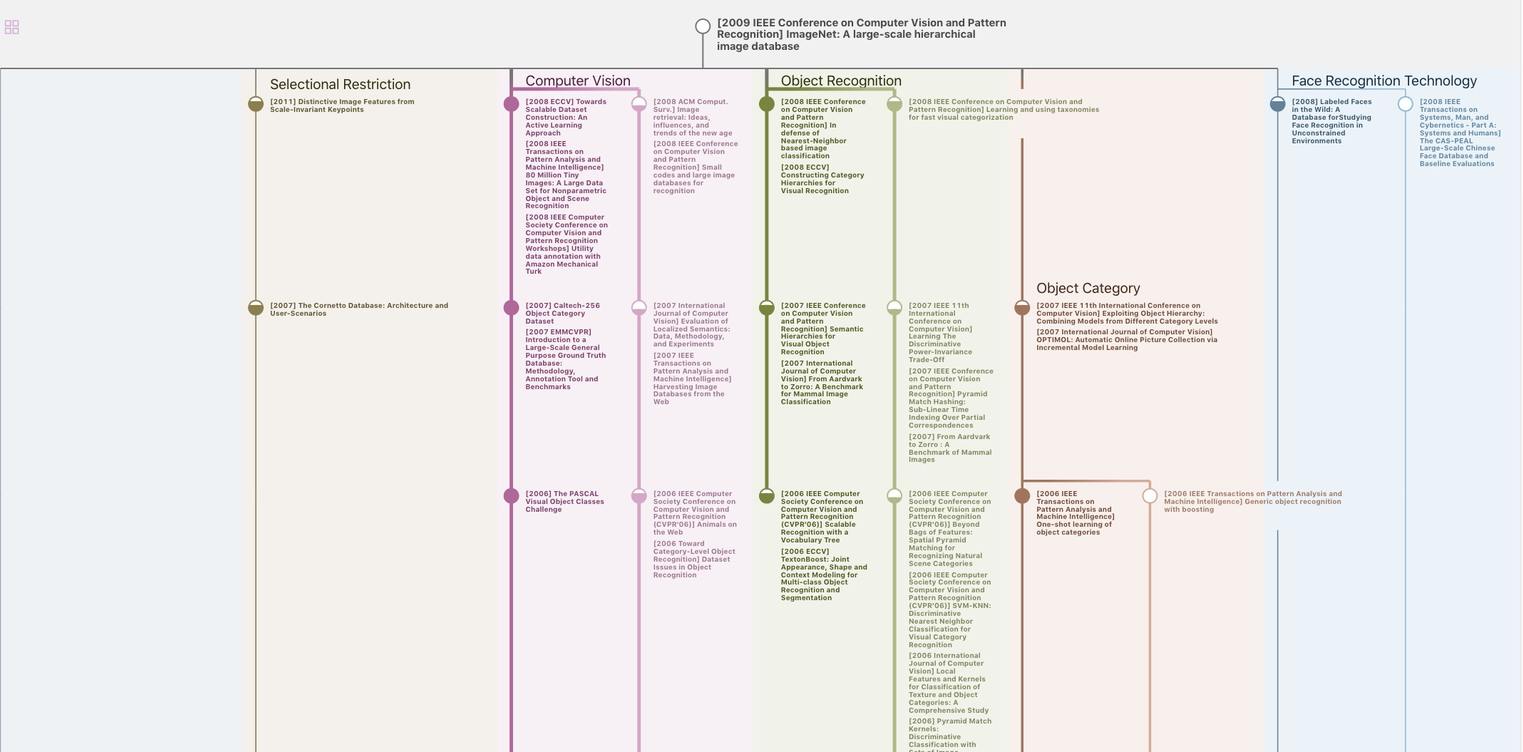
生成溯源树,研究论文发展脉络
Chat Paper
正在生成论文摘要