Latent Dirichlet Allocation For Automatic Document Categorization
MACHINE LEARNING AND KNOWLEDGE DISCOVERY IN DATABASES, PT II(2009)
摘要
In this paper we introduce and evaluate a technique for applying latent Dirichlet, allocation to supervised semantic categorization of documents. In our setup, for every category an own collection of topics is assigned, and for a labeled training document only topics from its category are sampled. Thus, compared to the classical LDA that processes the entire corpus in one, we essentially build separate LDA models for each category with the category-specific topics, and then these topic collections are put together to form a unified LDA model. For an unseen document the inferred topic distribution gives an estimation how much the document fits into the category.We use this method for Web document classification. Our key results are 46% decrease in 1-AUC value in classification accuracy over tf.idf with SVM and 43% over the plain LDA baseline with SVM. Using a careful vocabulary selection method and a heuristic which handles the effect; that similar topics may arise in distinct categories the improvement; is 83% over tf.idf with SVM and 82% over LDA with SVM in 1-AUC.
更多查看译文
关键词
classical LDA,plain LDA baseline,separate LDA model,unified LDA model,Web document classification,distinct category,training document,unseen document,1-AUC value,careful vocabulary selection method,Automatic Document Categorization,Latent Dirichlet Allocation
AI 理解论文
溯源树
样例
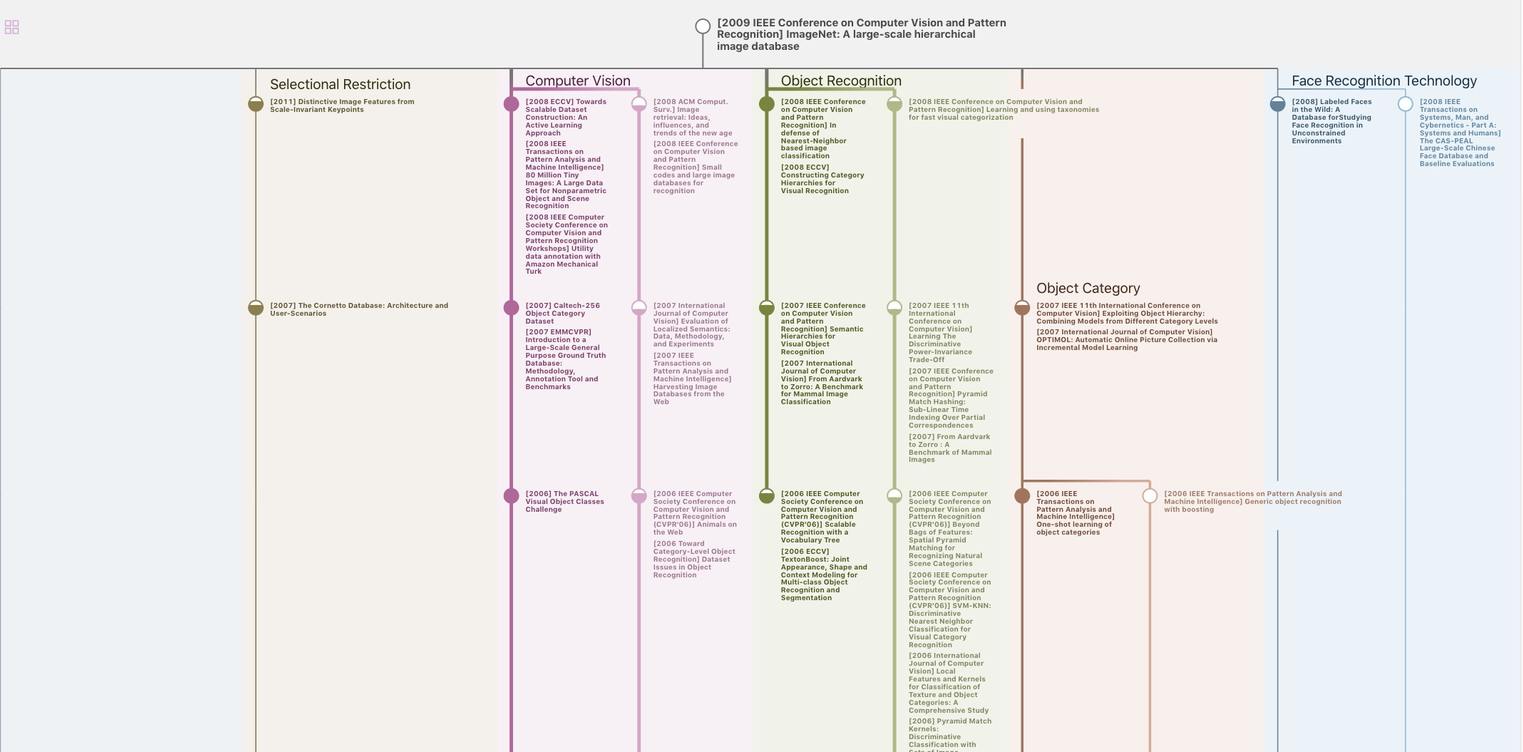
生成溯源树,研究论文发展脉络
Chat Paper
正在生成论文摘要