Semantic Image Synthesis via Class-Adaptive Cross-Attention
CoRR(2023)
摘要
In semantic image synthesis, the state of the art is dominated by methods that use spatially-adaptive normalization layers, which allow for excellent visual generation quality and editing versatility. Granted their efficacy, recent research efforts have focused toward finer-grained local style control and multi-modal generation. By construction though, such layers tend to overlook global image statistics leading to unconvincing local style editing and causing global inconsistencies such as color or illumination distribution shifts. Also, the semantic layout is required for mapping styles in the generator, putting a strict alignment constraint over the features. In response, we designed a novel architecture where cross-attention layers are used in place of de-normalization ones for conditioning the image generation. Our model inherits the advantages of both solutions, retaining state-of-the-art reconstruction quality, as well as improved global and local style transfer. Code and models available at https://github.com/TFonta/CA2SIS.
更多查看译文
关键词
semantic image synthesis,class-adaptive,cross-attention
AI 理解论文
溯源树
样例
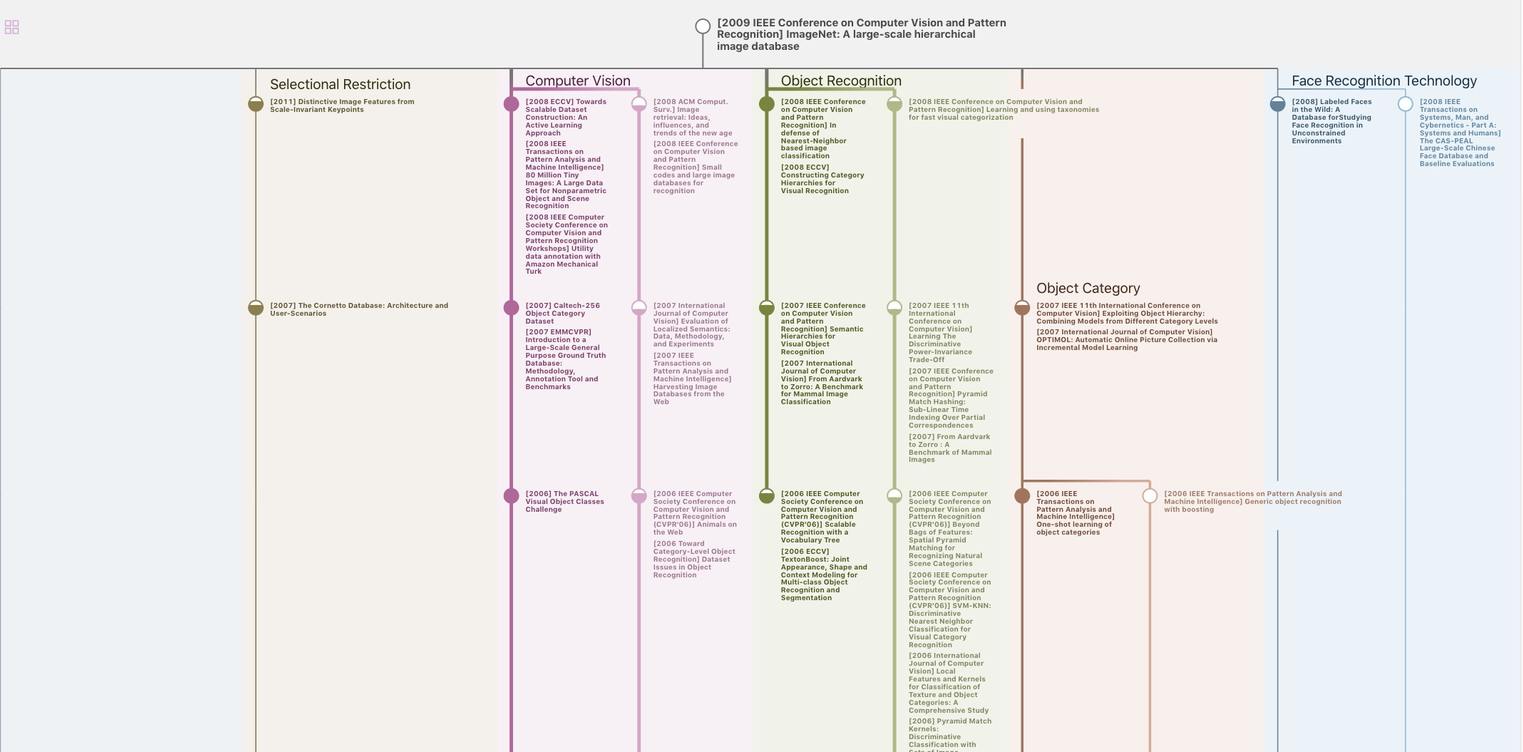
生成溯源树,研究论文发展脉络
Chat Paper
正在生成论文摘要