Minimum Width for Deep, Narrow MLP: A Diffeomorphism and the Whitney Embedding Theorem Approach
CoRR(2023)
摘要
Recently, there has been significant attention on determining the minimum width for the universal approximation property of deep, narrow MLPs. Among these challenges, approximating a continuous function under the uniform norm is important and challenging, with the gap between its lower and upper bound being hard to narrow. In this regard, we propose a novel upper bound for the minimum width, given by $\operatorname{max}(2d_x+1, d_y) + \alpha(\sigma)$, to achieve uniform approximation in deep narrow MLPs, where $0\leq \alpha(\sigma)\leq 2$ represents the constant depending on the activation function. We demonstrate this bound through two key proofs. First, we establish that deep, narrow MLPs with little additional width can approximate diffeomorphisms. Secondly, we utilize the Whitney embedding theorem to show that any continuous function can be approximated by embeddings, further decomposed into linear transformations and diffeomorphisms.
更多查看译文
AI 理解论文
溯源树
样例
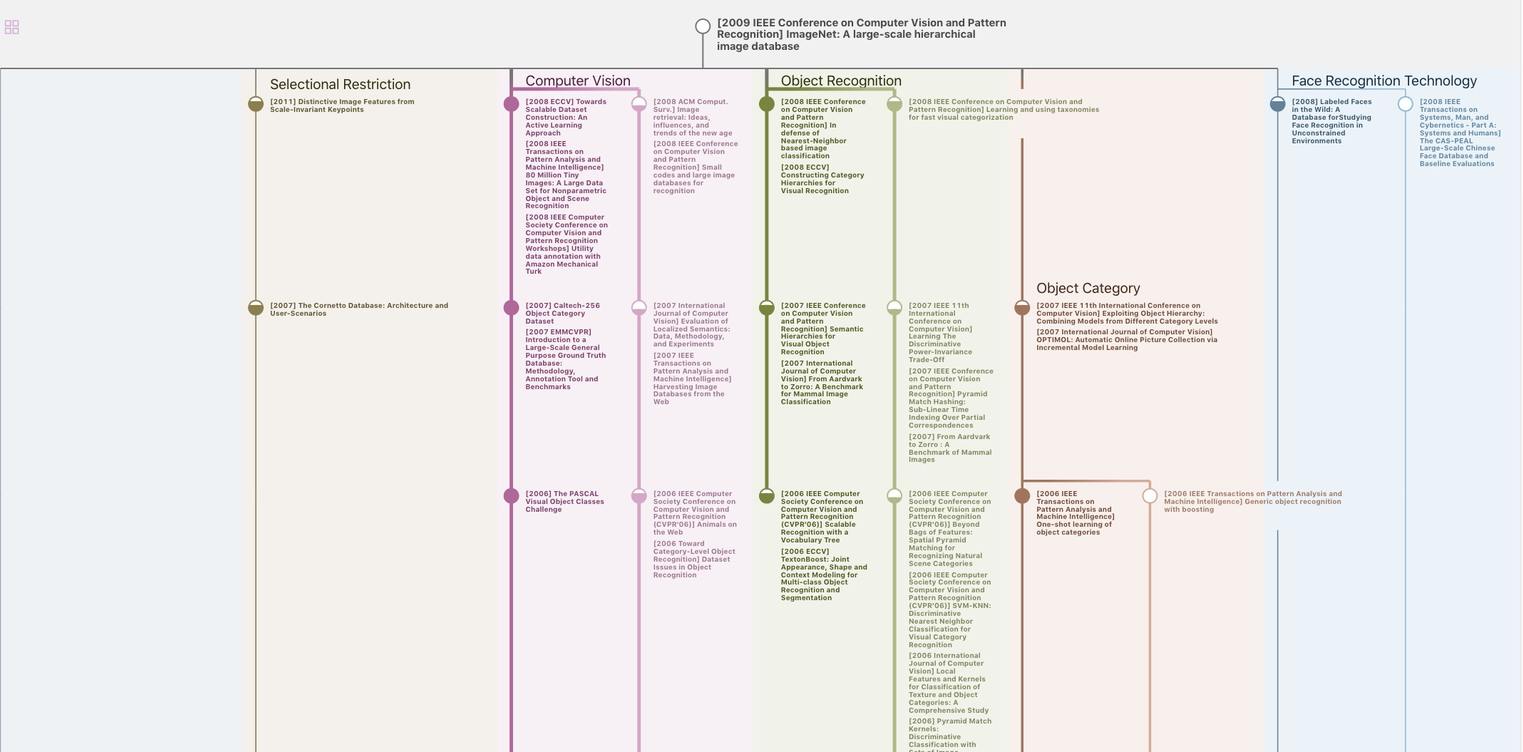
生成溯源树,研究论文发展脉络
Chat Paper
正在生成论文摘要