Vulnerability of Machine Learning Approaches Applied in IoT-based Smart Grid: A Review
CoRR(2023)
摘要
The machine learning (ML) sees an increasing prevalence of being used in the internet-of-things enabled smart grid. However, the trustworthiness of ML is a severe issue that must be addressed to accommodate the trend of ML-based smart grid applications (MLsgAPPs). The adversarial distortion injected into the power signal will greatly affect the system's normal control and operation. Therefore, it is imperative to conduct vulnerability assessment for MLsgAPPs applied in the context of safety-critical power systems. In this paper, we provide a comprehensive review of the recent progress in designing attack and defense methods for MLsgAPPs. Unlike the traditional survey about ML security, this is the first review work about the security of MLsgAPPs that focuses on the characteristics of power systems. The survey is organized from the aspects of adversarial assumptions, targeted applications, evaluation metrics, defending approaches, physics-related constraints, and applied datasets. We also highlight future directions on this topic to encourage more researchers to conduct further research on adversarial attacks and defending approaches for MLsgAPPs.
更多查看译文
关键词
Smart Grid,Vulnerability Assessment,Adversarial Machine Learning,Power System Specifics,Physical constraints
AI 理解论文
溯源树
样例
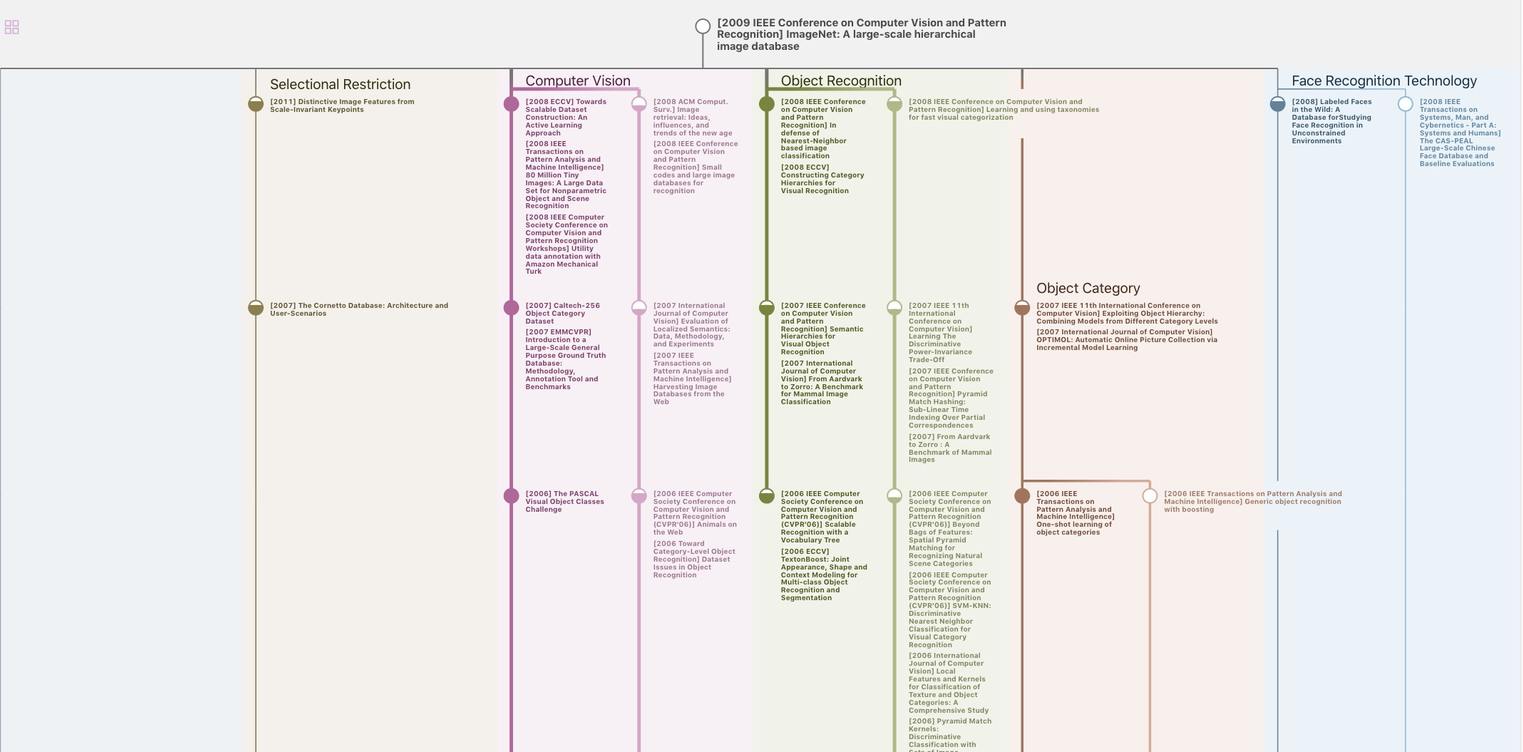
生成溯源树,研究论文发展脉络
Chat Paper
正在生成论文摘要