Representing the Disciplinary Structure of Physics: A Comparative Evaluation of Graph and Text Embedding Methods
CoRR(2023)
摘要
Recent advances in machine learning offer new ways to represent and study scholarly works and the space of knowledge. Graph and text embeddings provide a convenient vector representation of scholarly works based on citations and text. Yet, it is unclear whether their representations are consistent or provide different views of the structure of science. Here, we compare graph and text embedding by testing their ability to capture the hierarchical structure of the Physics and Astronomy Classification Scheme (PACS) of papers published by the American Physical Society (APS). We also provide a qualitative comparison of the overall structure of the graph and text embeddings for reference. We find that neural network-based methods outperform traditional methods and graph embedding methods such as node2vec are better than other methods at capturing the PACS structure. Our results call for further investigations into how different contexts of scientific papers are captured by different methods, and how we can combine and leverage such information in an interpretable manner.
更多查看译文
关键词
disciplinary structure,graph,physics
AI 理解论文
溯源树
样例
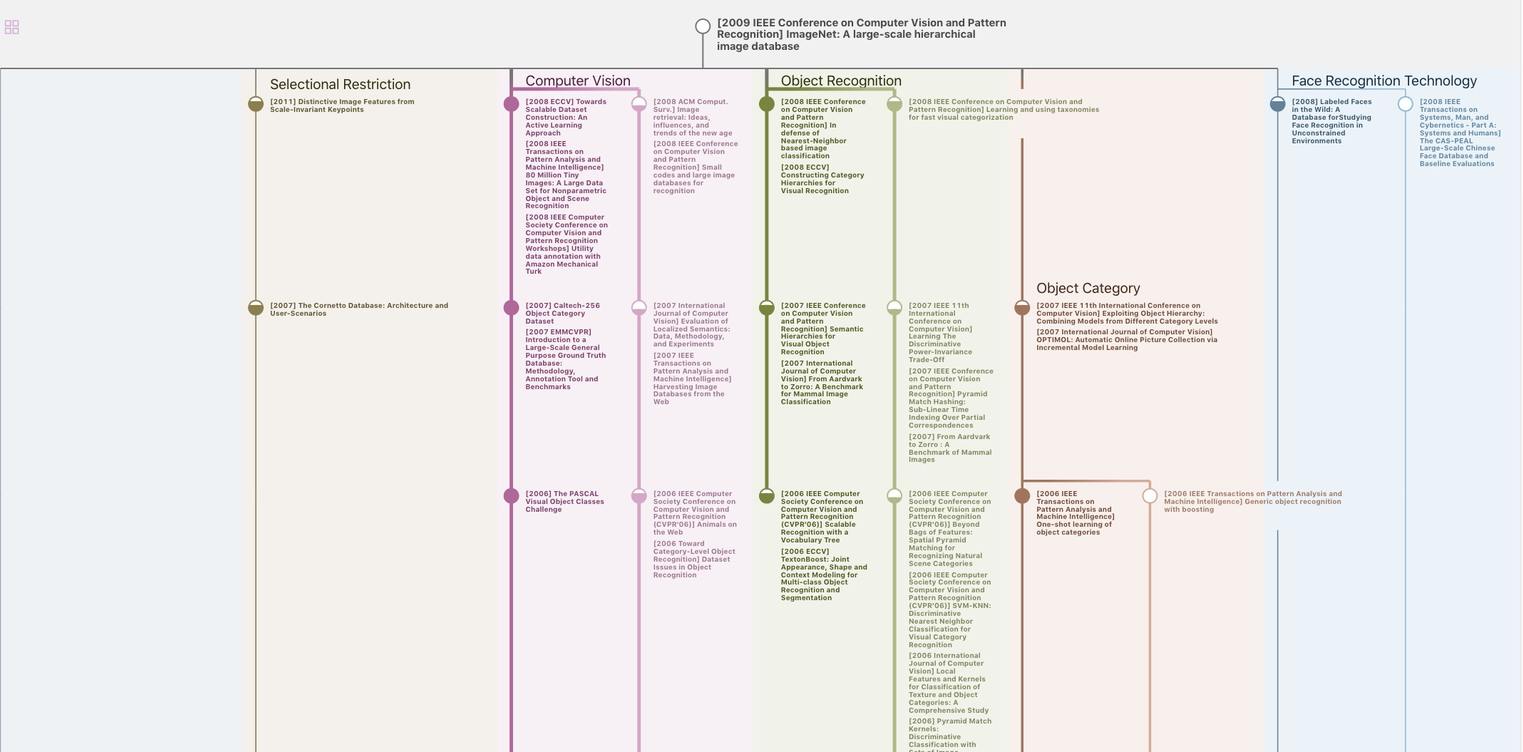
生成溯源树,研究论文发展脉络
Chat Paper
正在生成论文摘要