Bridging Distribution Learning and Image Clustering in High-dimensional Space
2023 IEEE Virtual Conference on Communications (VCC)(2023)
摘要
Distribution learning focuses on learning the probability density function from a set of data samples. In contrast, clustering aims to group similar objects together in an unsupervised manner. Usually, these two tasks are considered unrelated. However, the relationship between the two may be indirectly correlated, with Gaussian Mixture Models (GMM) acting as a bridge. In this paper, we focus on exploring the correlation between distribution learning and clustering, with the motivation to fill the gap between these two fields, utilizing an autoencoder (AE) to encode images into a high-dimensional latent space. Then, Monte-Carlo Marginalization (MCMarg) and Kullback-Leibler (KL) divergence loss are used to fit the Gaussian components of the GMM and learn the data distribution. Finally, image clustering is achieved through each Gaussian component of GMM. Yet, the "curse of dimensionality" poses severe challenges for most clustering algorithms. Compared with the classic Expectation-Maximization (EM) Algorithm, experimental results show that MCMarg and KL divergence can greatly alleviate the difficulty. Based on the experimental results, we believe distribution learning can exploit the potential of GMM in image clustering within high-dimensional space.
更多查看译文
关键词
Clustering Algorithm
AI 理解论文
溯源树
样例
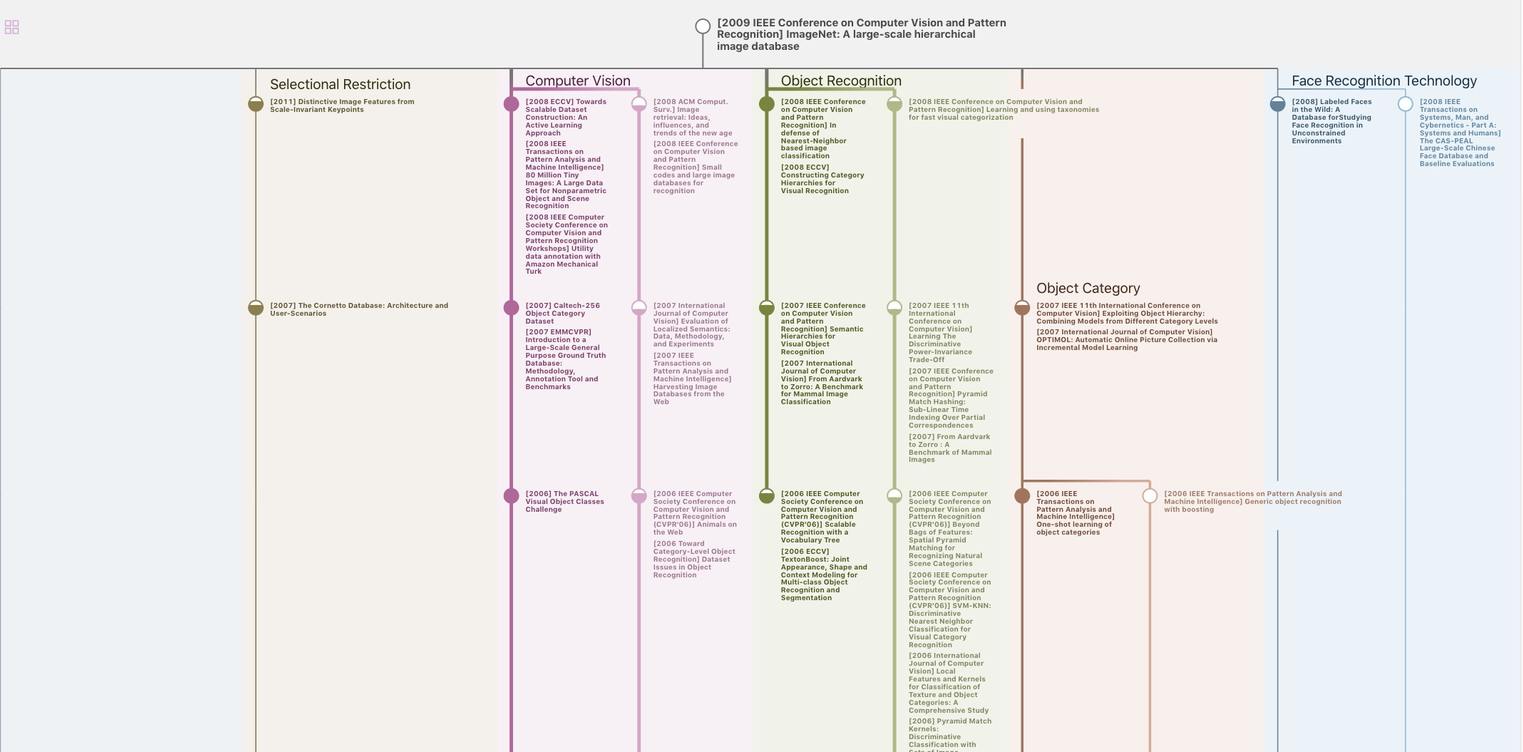
生成溯源树,研究论文发展脉络
Chat Paper
正在生成论文摘要