Classification robustness to common optical aberrations
2023 IEEE/CVF INTERNATIONAL CONFERENCE ON COMPUTER VISION WORKSHOPS, ICCVW(2023)
摘要
Computer vision using deep neural networks (DNNs) has brought about seminal changes in people's lives. Applications range from automotive, face recognition in the security industry, to industrial process monitoring. In some cases, DNNs infer even in safety-critical situations. Therefore, for practical applications, DNNs have to behave in a robust way to disturbances such as noise, pixelation, or blur. Blur directly impacts the performance of DNNs, which are often approximated as a disk-shaped kernel to model defocus. However, optics suggests that there are different kernel shapes depending on wavelength and location caused by optical aberrations. In practice, as the optical quality of a lens decreases, such aberrations increase. This paper proposes OpticsBench, a benchmark for investigating robustness to realistic, practically relevant optical blur effects. Each corruption represents an optical aberration (coma, astigmatism, spherical, trefoil) derived from Zernike Polynomials. Experiments on ImageNet show that for a variety of different pre-trained DNNs, the performance varies strongly compared to disk-shaped kernels, indicating the necessity of considering realistic image degradations. In addition, we show on ImageNet-100 with OpticsAugment that robustness can be increased by using optical kernels as data augmentation. Compared to a conventionally trained ResNeXt50, training with OpticsAugment achieves an average performance gain of 21.7% points on OpticsBench and 6.8% points on 2D common corruptions.
更多查看译文
关键词
Point Spread Function,MTF,DNN,Automotive,Lens
AI 理解论文
溯源树
样例
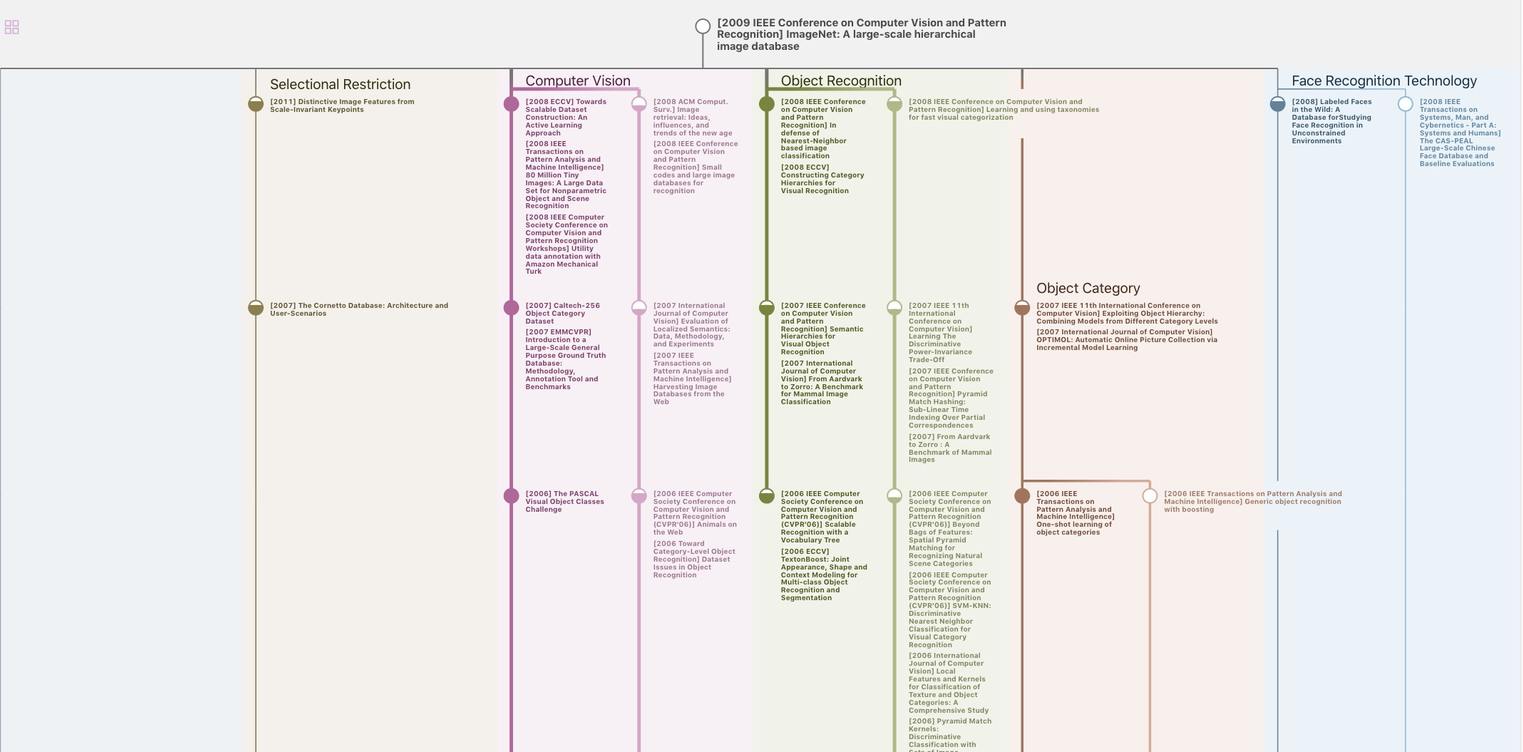
生成溯源树,研究论文发展脉络
Chat Paper
正在生成论文摘要