Input Margins Can Predict Generalization Too
AAAI 2024(2024)
摘要
Understanding generalization in deep neural networks is an active area of research. A promising avenue of exploration has been that of margin measurements: the shortest distance to the decision boundary for a given sample or its representation internal to the network. While margins have been shown to be correlated with the generalization ability of a model when measured at its hidden representations (hidden margins), no such link between large margins and generalization has been established for input margins. We show that while input margins are not generally predictive of generalization, they can be if the search space is appropriately constrained.
We develop such a measure based on input margins, which we refer to as 'constrained margins'. The predictive power of this new measure is demonstrated on the 'Predicting Generalization in Deep Learning' (PGDL) dataset and contrasted with hidden representation margins. We find that constrained margins achieve highly competitive scores and outperform other margin measurements in general. This provides a novel insight on the relationship between generalization and classification margins, and highlights the importance of considering the data manifold for investigations of generalization in DNNs.
更多查看译文
关键词
ML: Representation Learning,ML: Adversarial Learning & Robustness,ML: Deep Learning Theory
AI 理解论文
溯源树
样例
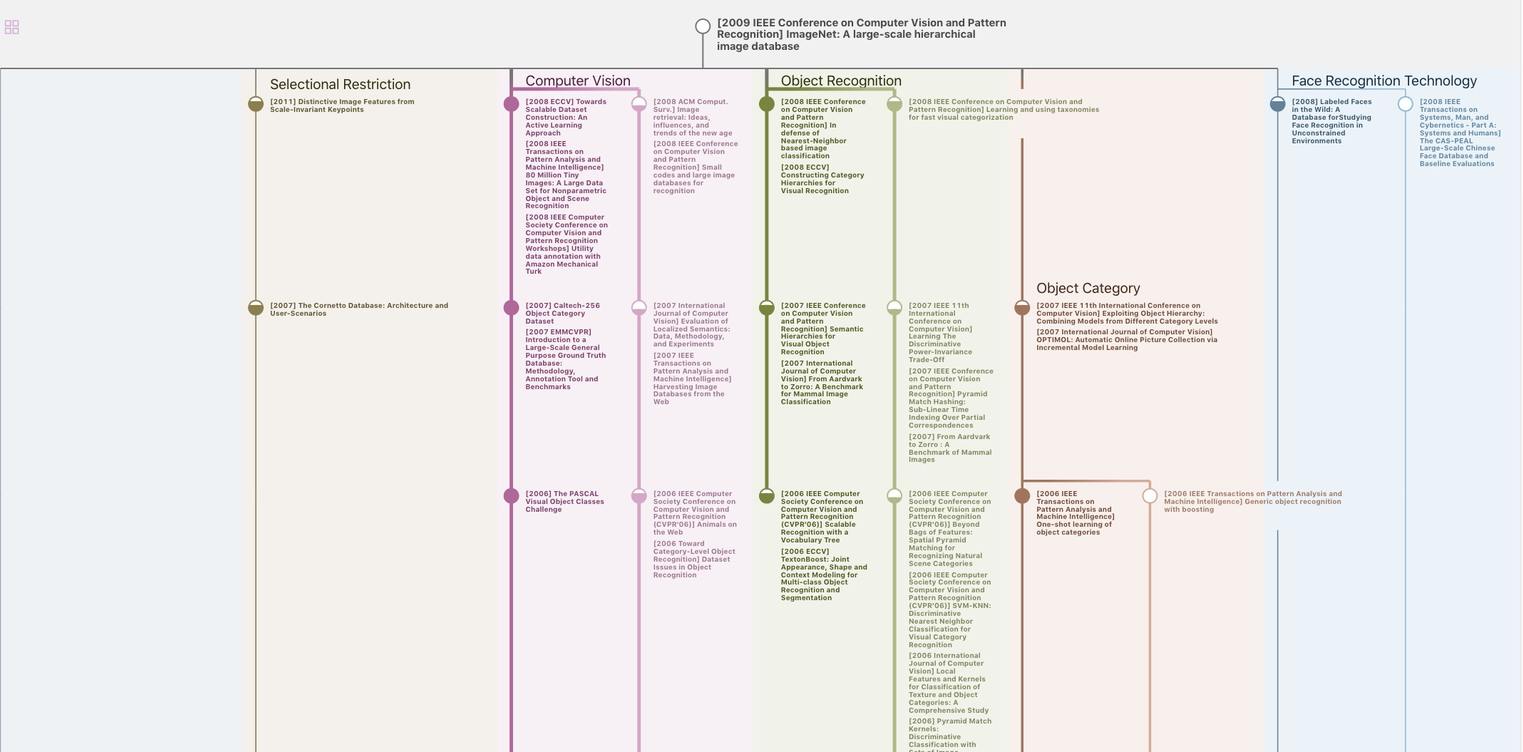
生成溯源树,研究论文发展脉络
Chat Paper
正在生成论文摘要