Uncertainty-driven Affordance Discovery for Efficient Robotics Manipulation
CoRR(2023)
摘要
Robotics affordances, providing information about what actions can be taken in a given situation, can aid robotics manipulation. However, learning about affordances requires expensive large annotated datasets of interactions or demonstrations. In this work, we show active learning can mitigate this problem and propose the use of uncertainty to drive an interactive affordance discovery process. We show that our method enables the efficient discovery of visual affordances for several action primitives, such as grasping, stacking objects, or opening drawers, strongly improving data efficiency and allowing us to learn grasping affordances on a real-world setup with an xArm 6 robot arm in a small number of trials.
更多查看译文
关键词
affordance discovery,efficient robotics
AI 理解论文
溯源树
样例
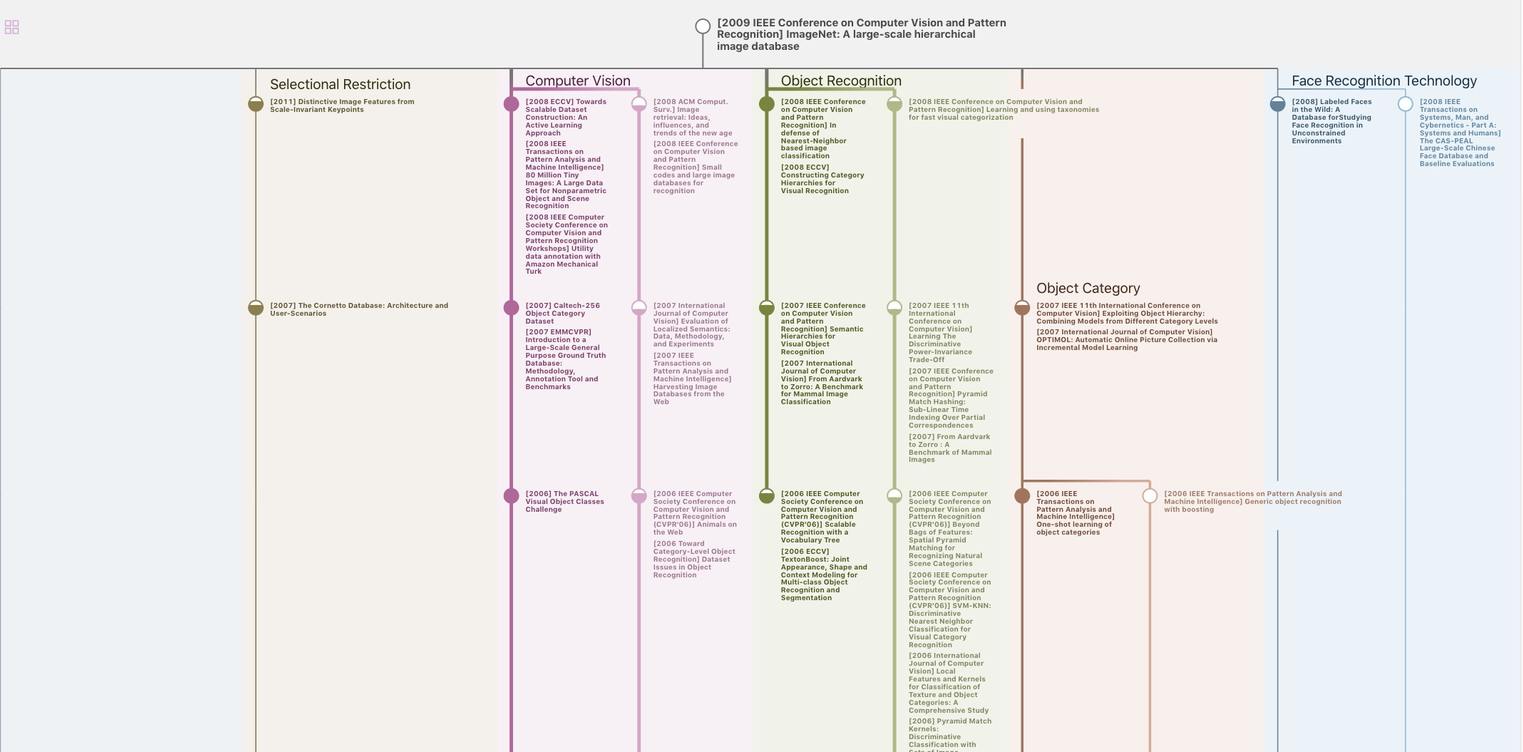
生成溯源树,研究论文发展脉络
Chat Paper
正在生成论文摘要