XVir: A Transformer-Based Architecture for Identifying Viral Reads from Cancer Samples
CoRR(2023)
摘要
It is estimated that approximately 15% of cancers worldwide can be linked to viral infections. The viruses that can cause or increase the risk of cancer include human papillomavirus, hepatitis B and C viruses, Epstein-Barr virus, and human immunodeficiency virus, to name a few. The computational analysis of the massive amounts of tumor DNA data, whose collection is enabled by the recent advancements in sequencing technologies, have allowed studies of the potential association between cancers and viral pathogens. However, the high diversity of oncoviral families makes reliable detection of viral DNA difficult and thus, renders such analysis challenging. In this paper, we introduce XVir, a data pipeline that relies on a transformer-based deep learning architecture to reliably identify viral DNA present in human tumors. In particular, XVir is trained on genomic sequencing reads from viral and human genomes and may be used with tumor sequence information to find evidence of viral DNA in human cancers. Results on semi-experimental data demonstrate that XVir is capable of achieving high detection accuracy, generally outperforming state-of-the-art competing methods while being more compact and less computationally demanding.
更多查看译文
关键词
viral reads,transformer-based
AI 理解论文
溯源树
样例
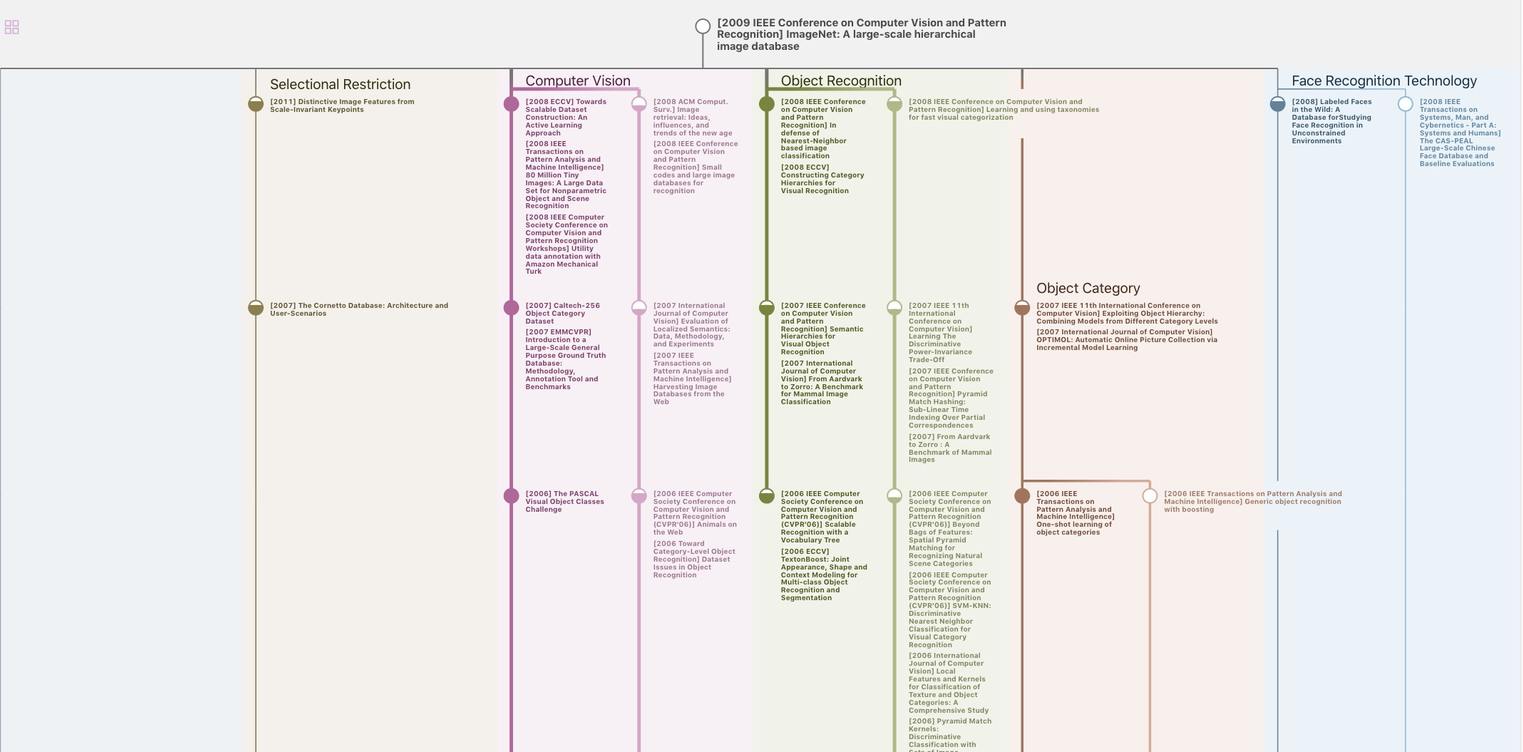
生成溯源树,研究论文发展脉络
Chat Paper
正在生成论文摘要