Novel Personalized Multimedia Recommendation Systems Using Tensor Singular-Value-Decomposition
2023 IEEE International Symposium on Broadband Multimedia Systems and Broadcasting (BMSB)(2023)
摘要
Nowadays, multimedia data are often produced by various sources, e.g., Internet of Things (IoT), social media, customer databases, etc. As tremendous multimedia data are produced every day, users or E-commerce customers cannot infer information from such data by themselves and therefore recommender systems have been developed to help users to select the products or services which better fit users’ preferences or requirements. Nonetheless, there exists few works on the incorporation of side information or multiple attributes about items into the design of a more robust recommender system. In this work, we propose a novel approach based on the third-order tensor singular-value-decomposition (T3-SVD) to design new personalized multimedia recommender systems (PMRSs) for internet users. A PMRS can dynamically adjust its recommendation strategy subject to a particular user’s on-line transaction behavior. To evaluate the effectiveness of our proposed PMRS based on T3-SVD, we compare the performances of our proposed new PMRS and two other existing tensor-based recommendation systems over realworld data in terms of normalized root-mean-square error (NRMSE). As a result, our proposed new PMRS greatly outperforms the other two existing systems.
更多查看译文
关键词
Personalized multimedia recommendation system (PMRS),tensor singular-value-decomposition,normalized root-mean-square error (NRMSE),E-commerce
AI 理解论文
溯源树
样例
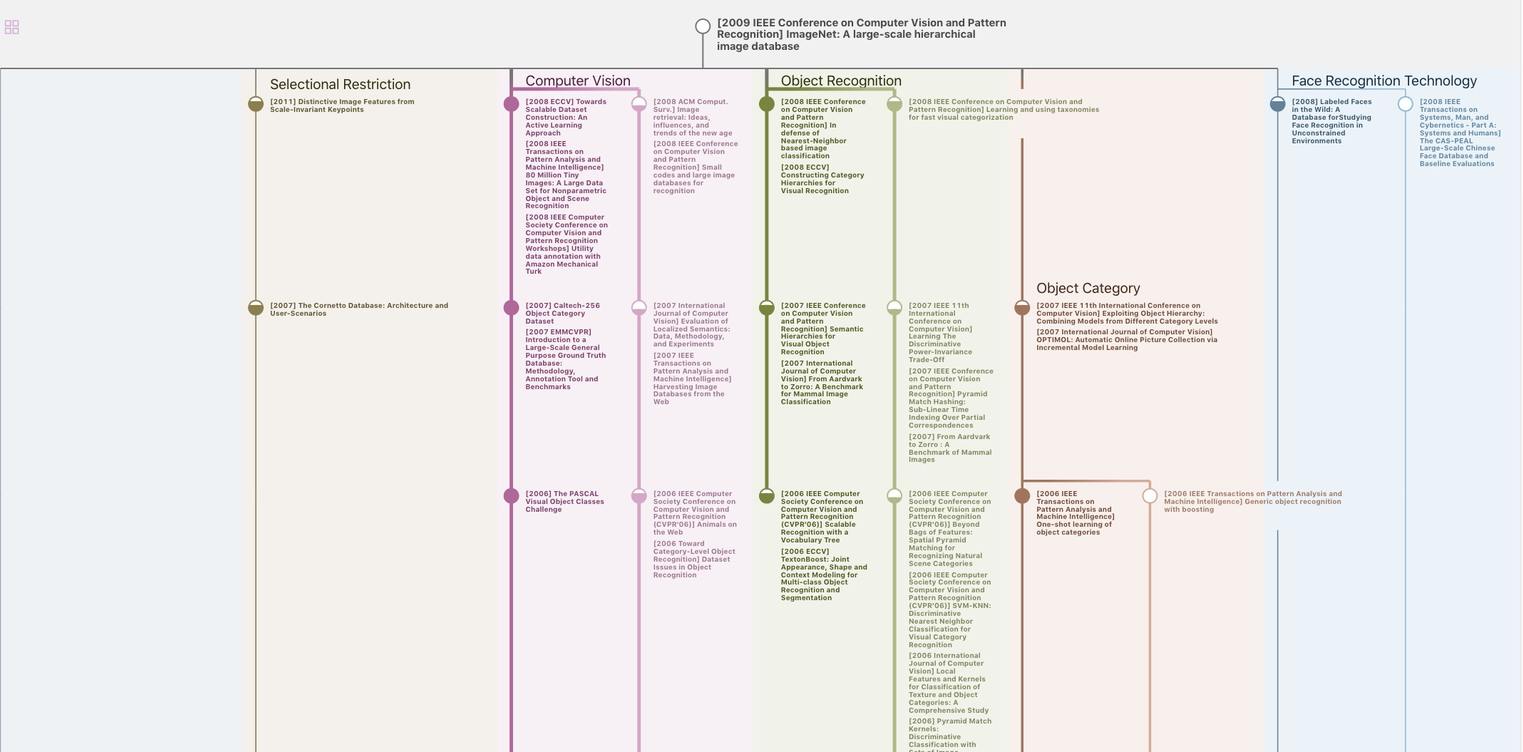
生成溯源树,研究论文发展脉络
Chat Paper
正在生成论文摘要