Design of Ferroelectric FET-Based Capacitive-Coupling Computing-In-Memory For Binary Neural Networks
2023 China Semiconductor Technology International Conference (CSTIC)(2023)
摘要
Ferroelectric FETs (FeFETs) have been applied to computing-in-memories (CIMs) for binary neural networks (BNNs) because of the advantages of ultra-low energy cost, while suffering from large output variation or write disturb. This work proposes a novel FeFET-based CIM for BNNs, reducing the output variation by exploiting charge-domain scheme and avoiding write disturb by exploiting additional access transistors. Simulation results show that compared with conventional FeFET-based current-domain design, the proposed design can improve accuracy by 20% on MNIST by reducing the output variation. Compared with previous SRAM-based and RRAM-based CIM for BNNs, the proposed design can reduce energy cost by 54% and 98%, showing its great potential for edge AI applications.
更多查看译文
AI 理解论文
溯源树
样例
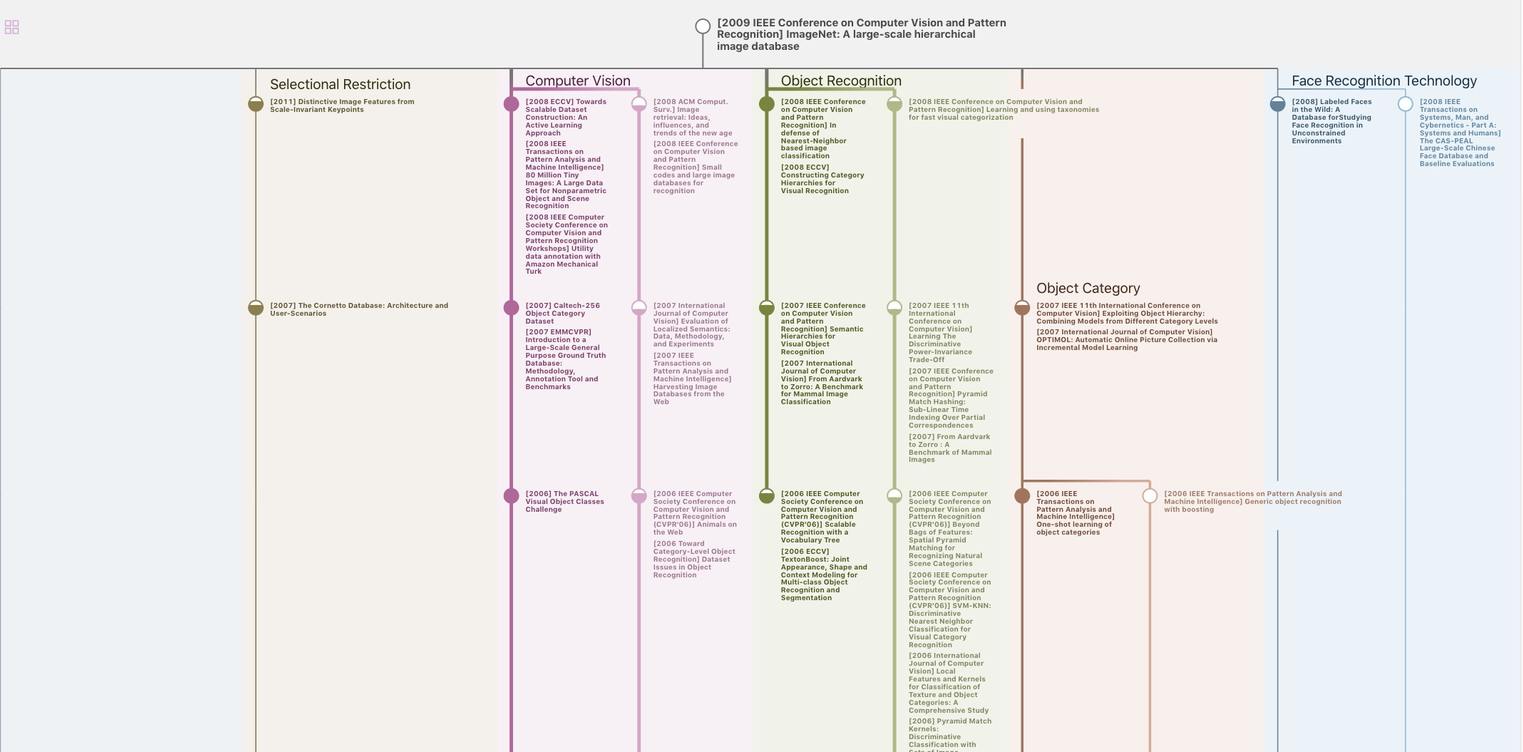
生成溯源树,研究论文发展脉络
Chat Paper
正在生成论文摘要