TinyFL: On-Device Training, Communication And Aggregation On A Microcontroller For Federated Learning
2023 21st IEEE Interregional NEWCAS Conference (NEWCAS)(2023)
摘要
In federated learning (FL), in contrast to centralized ML learning processes, ML models are sent rather than the raw data. Therefore, FL is a decentralized and privacy-compliant process currently experiencing significant research interest. As a result, initial investigations were carried out with FL and microcontrollers (MCUs). However, each of these studies used a PC as a server. In this work, we introduce TinyFL, a method using only MCUs to build a low-cost, low-power, and low-storage system. TinyFL uses a hybrid master/slave protocol where the master MCU is responsible for communication and aggregation. Thereby, the communication is performed by inter-integrated circuit ((IC)-C-2). TinyFL demonstrates that communication and aggregation for FL can be performed on only MCUs. Furthermore, we show that the training with TinyFL is 11.57% faster than centralized training using a gesture recognition use case.
更多查看译文
关键词
Federated Learning,Microcontroller,On-Device Aggregation,On-Device Training,Embedded Systems,TinyFL
AI 理解论文
溯源树
样例
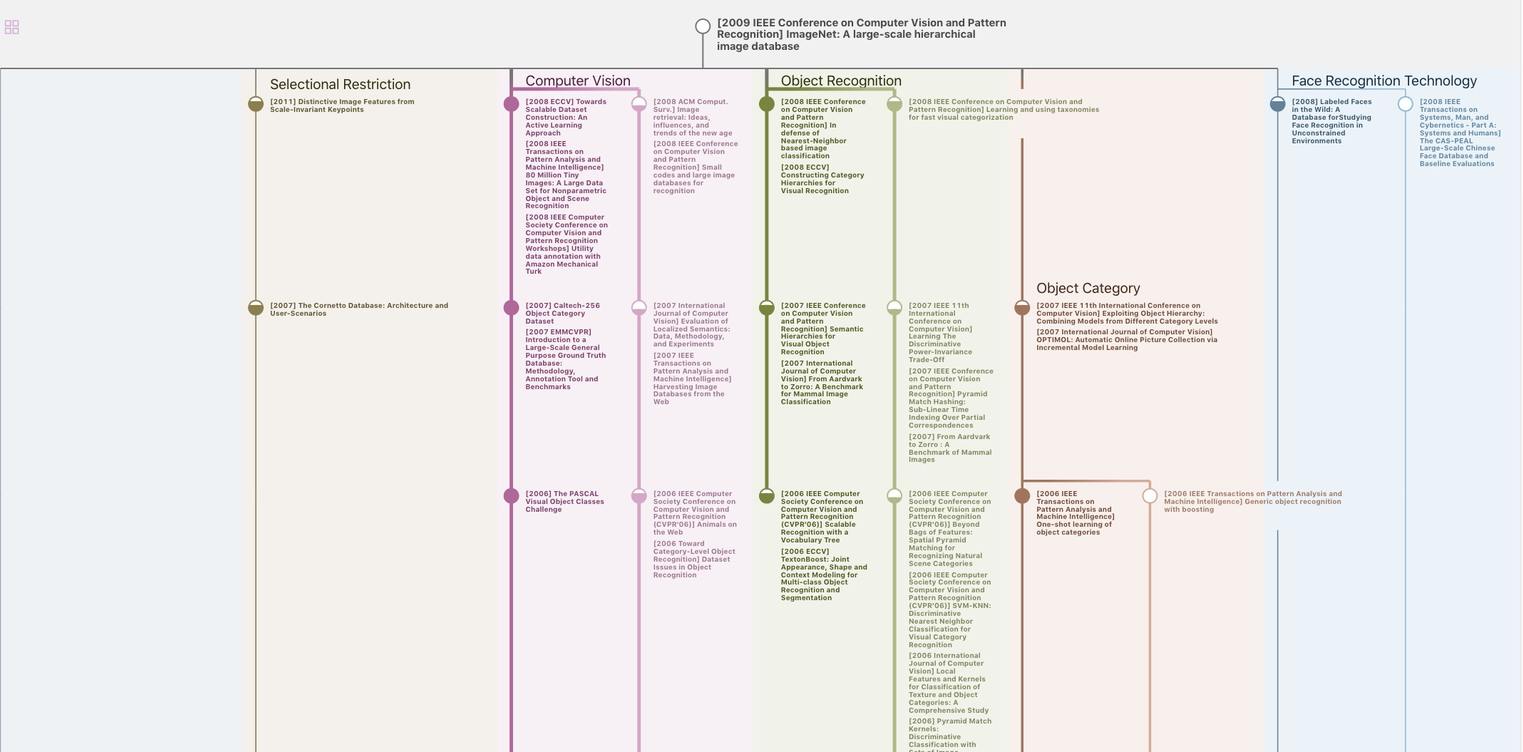
生成溯源树,研究论文发展脉络
Chat Paper
正在生成论文摘要