Design and Implementation of an FFT-Based Neural Network Accelerator Using Rapid Single-Flux-Quantum Technology
2023 21st IEEE Interregional NEWCAS Conference (NEWCAS)(2023)
摘要
Deep neural networks (DNNs) are continually expanding in size and complexity, making it essential to enhance their energy efficiency and performance without sacrificing accuracy. The model size significantly influences the performance, scalability, and energy efficiency of DNNs, which has led to increased interest in model compression and hardware acceleration research. This paper presents a novel convolution processor architecture specifically designed for a block-circulant matrices-based neural network processing approach. The proposed design employs ultra-fast (tens of gigahertz) superconductor-based devices and leverages fast Fourier transform (FFT)-based rapid multiplication, achieving a simultaneous reduction in both computational complexity and storage complexity from O(n(2)) to O(n log n). To validate the concept, we implement a 7-bit, 8-point convolution processing element (CPE) based on the proposed architecture, demonstrating high-speed operation up to 43.1 GHz. The implemented circuit consists of 21,575 Josephson junctions and is fabricated using a 10kA/cm(2) 9-layer niobium superconductor process.
更多查看译文
关键词
8-point convolution processing element,9-layer niobium superconductor process,block-circulant matrices-based neural network processing approach,computational complexity,convolution processor architecture,CPE,deep neural networks,DNN,energy efficiency,fast Fourier transform,FFT-based neural network accelerator,hardware acceleration research,Josephson junctions,rapid single-flux-quantum technology,storage complexity,word length 7 bit
AI 理解论文
溯源树
样例
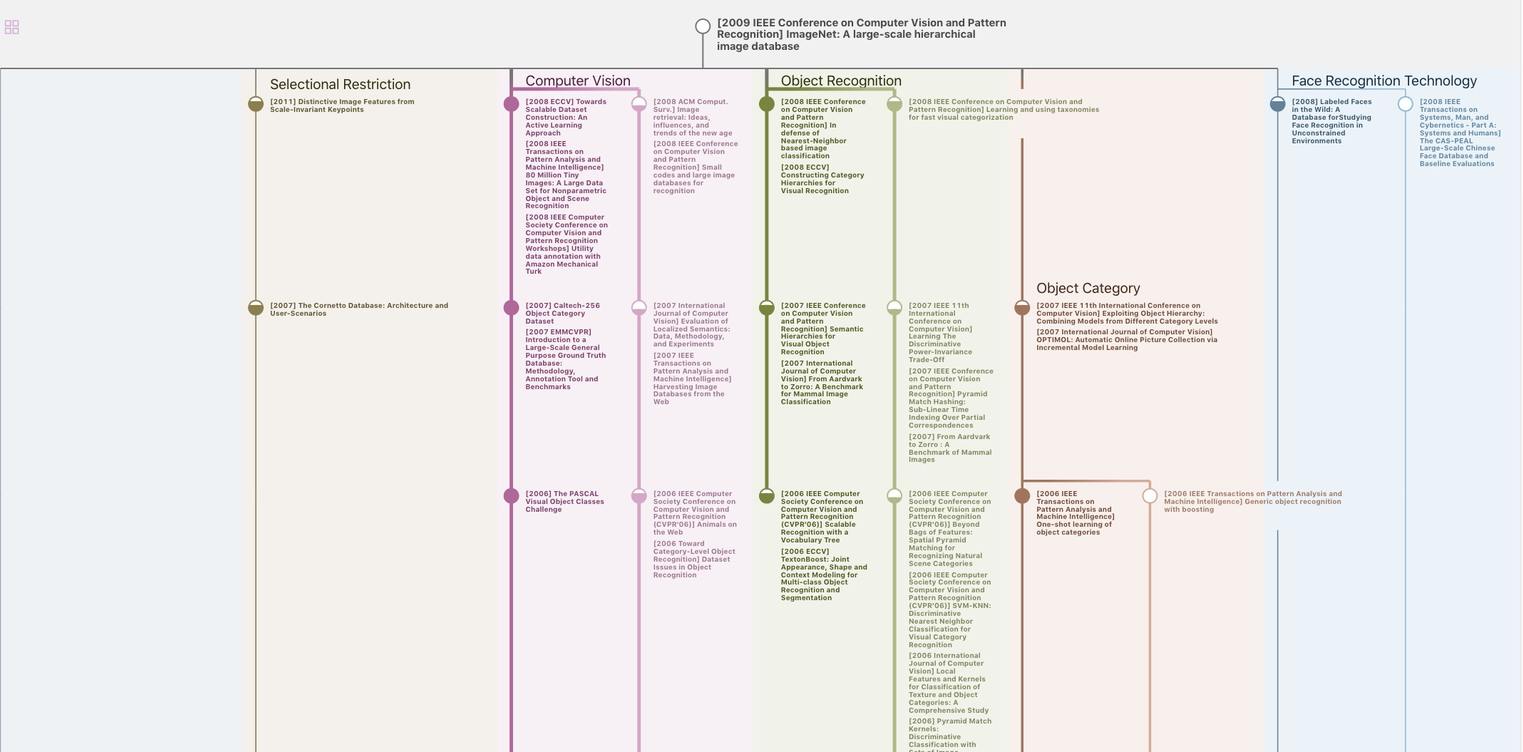
生成溯源树,研究论文发展脉络
Chat Paper
正在生成论文摘要