Symmetric Models for Visual Force Policy Learning
CoRR(2023)
摘要
While it is generally acknowledged that force feedback is beneficial to robotic control, applications of policy learning to robotic manipulation typically only leverage visual feedback. Recently, symmetric neural models have been used to significantly improve the sample efficiency and performance of policy learning across a variety of robotic manipulation domains. This paper explores an application of symmetric policy learning to visual-force problems. We present Symmetric Visual Force Learning (SVFL), a novel method for robotic control which leverages visual and force feedback. We demonstrate that SVFL can significantly outperform state of the art baselines for visual force learning and report several interesting empirical findings related to the utility of learning force feedback control policies in both general manipulation tasks and scenarios with low visual acuity.
更多查看译文
关键词
learning,models
AI 理解论文
溯源树
样例
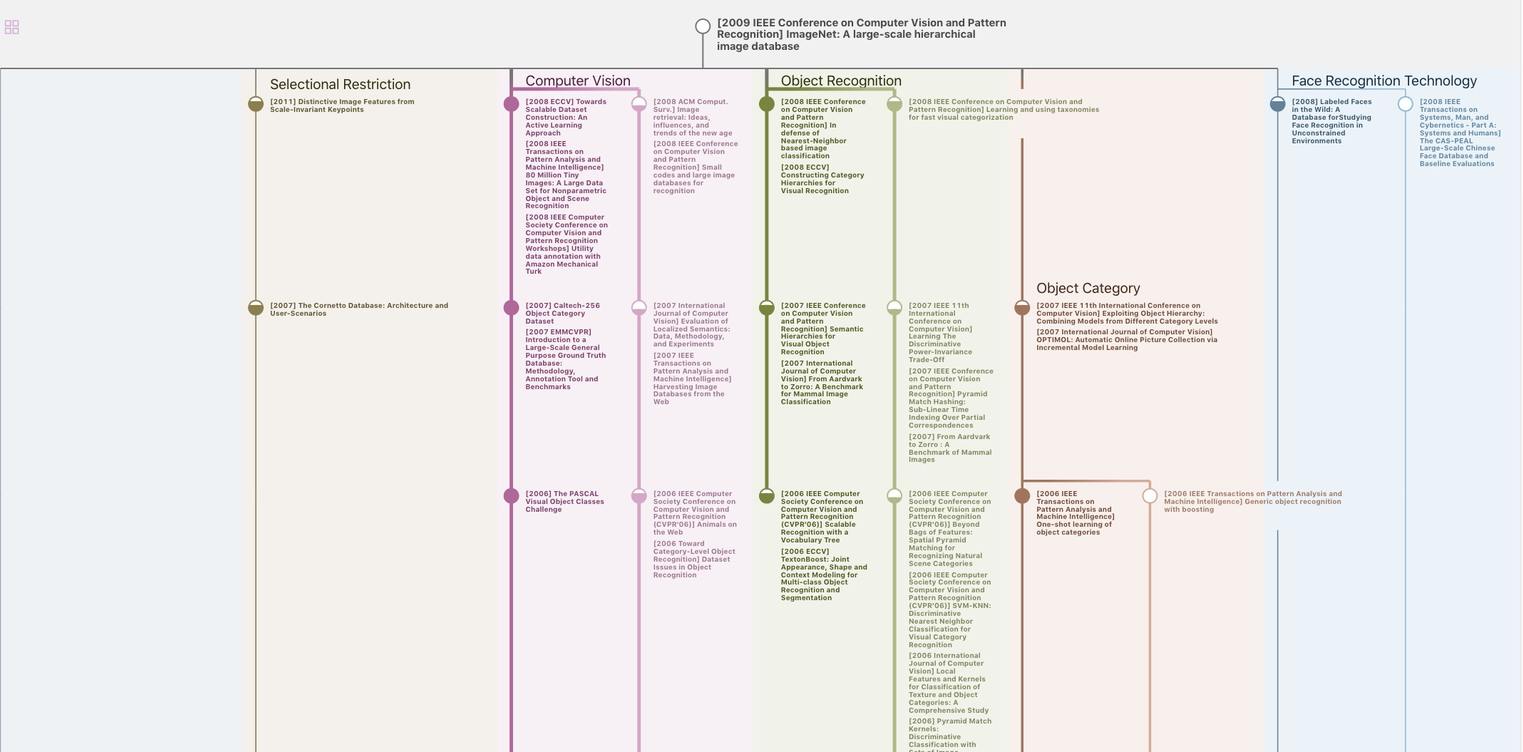
生成溯源树,研究论文发展脉络
Chat Paper
正在生成论文摘要