Rate-Optimal Policy Optimization for Linear Markov Decision Processes
CoRR(2023)
摘要
We study regret minimization in online episodic linear Markov Decision Processes, and obtain rate-optimal $\widetilde O (\sqrt K)$ regret where $K$ denotes the number of episodes. Our work is the first to establish the optimal (w.r.t.~$K$) rate of convergence in the stochastic setting with bandit feedback using a policy optimization based approach, and the first to establish the optimal (w.r.t.~$K$) rate in the adversarial setup with full information feedback, for which no algorithm with an optimal rate guarantee is currently known.
更多查看译文
关键词
linear markov decision processes,optimization,policy,rate-optimal
AI 理解论文
溯源树
样例
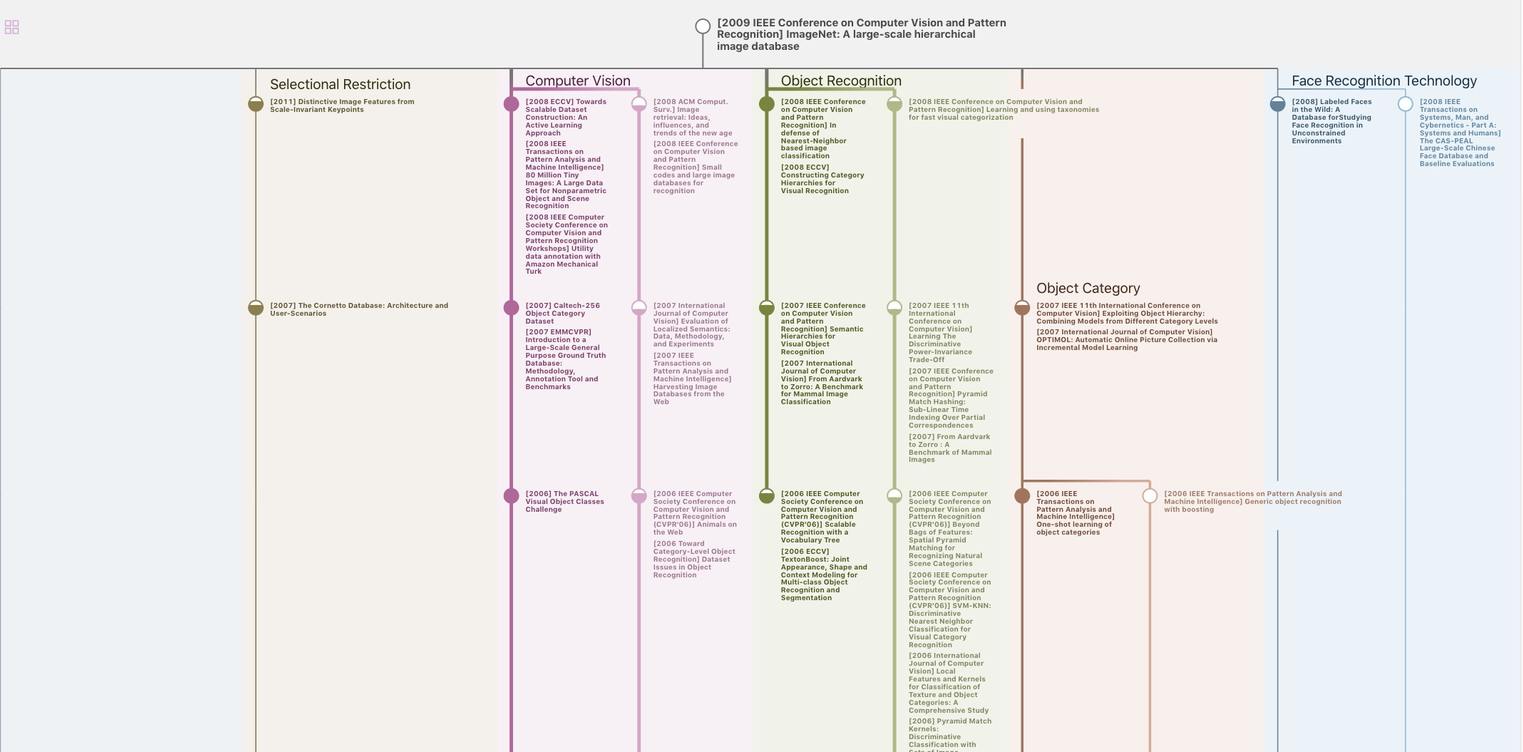
生成溯源树,研究论文发展脉络
Chat Paper
正在生成论文摘要