S-TREK: Sequential Translation and Rotation Equivariant Keypoints for local feature extraction
CoRR(2023)
摘要
In this work we introduce S-TREK, a novel local feature extractor that combines a deep keypoint detector, which is both translation and rotation equivariant by design, with a lightweight deep descriptor extractor. We train the S-TREK keypoint detector within a framework inspired by reinforcement learning, where we leverage a sequential procedure to maximize a reward directly related to keypoint repeatability. Our descriptor network is trained following a "detect, then describe" approach, where the descriptor loss is evaluated only at those locations where keypoints have been selected by the already trained detector. Extensive experiments on multiple benchmarks confirm the effectiveness of our proposed method, with S-TREK often outperforming other state-of-the-art methods in terms of repeatability and quality of the recovered poses, especially when dealing with in-plane rotations.
更多查看译文
关键词
rotation equivariant keypoints,local feature extraction,feature extraction,sequential translation,s-trek
AI 理解论文
溯源树
样例
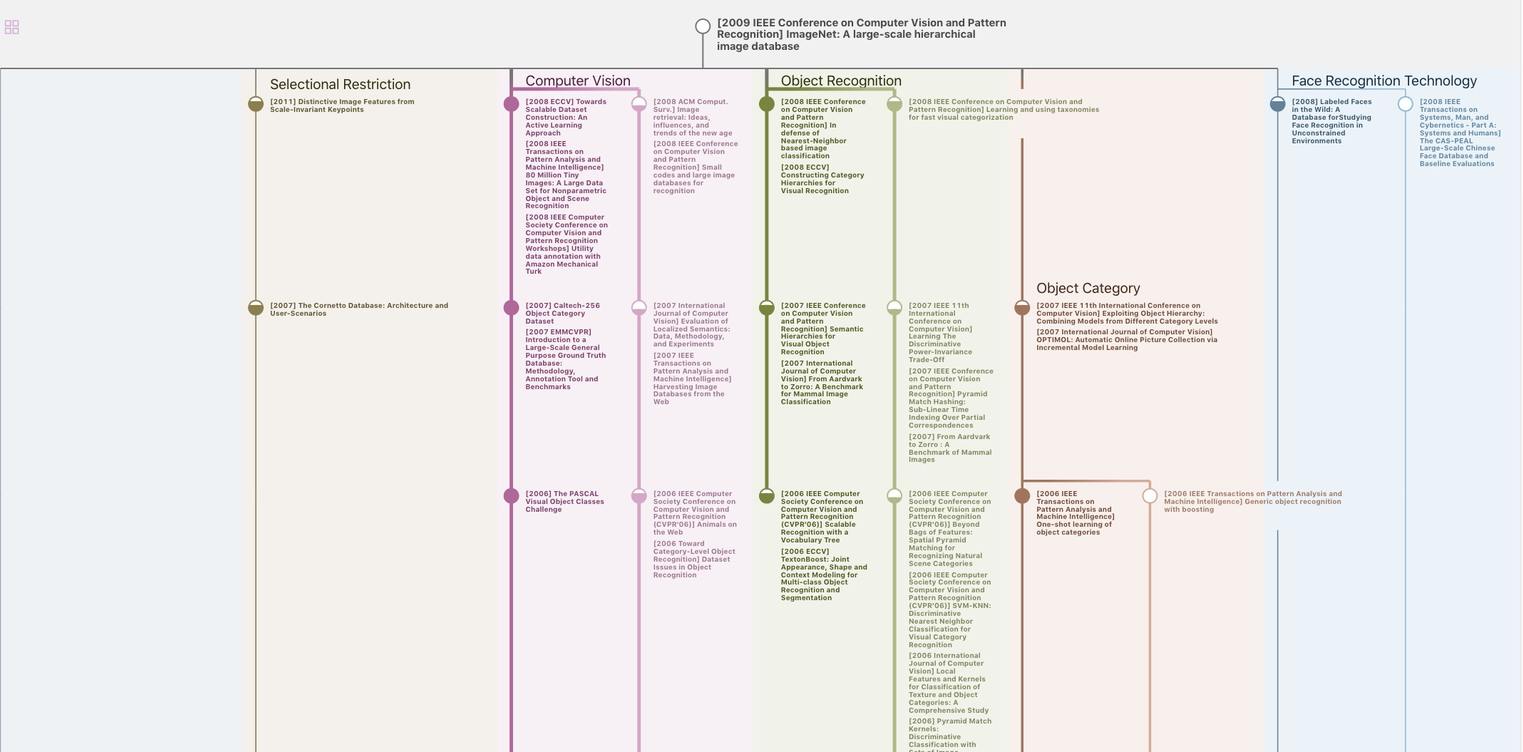
生成溯源树,研究论文发展脉络
Chat Paper
正在生成论文摘要