A flexible Bayesian tool for CoDa mixed models: logistic-normal distribution with Dirichlet covariance
Statistics and Computing(2024)
摘要
Compositional Data Analysis (CoDa) has gained popularity in recent years. This type of data consists of values from disjoint categories that sum up to a constant. Both Dirichlet regression and logistic-normal regression have become popular as CoDa analysis methods. However, fitting this kind of multivariate models presents challenges, especially when structured random effects are included in the model, such as temporal or spatial effects. To overcome these challenges, we propose the logistic-normal Dirichlet Model (LNDM). We seamlessly incorporate this approach into the R-INLA package, facilitating model fitting and model prediction within the framework of Latent Gaussian Models. Moreover, we explore metrics like Deviance Information Criteria, Watanabe Akaike information criterion, and cross-validation measure conditional predictive ordinate for model selection in R-INLA for CoDa. Illustrating LNDM through two simulated examples and with an ecological case study on Arabidopsis thaliana in the Iberian Peninsula, we underscore its potential as an effective tool for managing CoDa and large CoDa databases.
更多查看译文
关键词
CoDa,Dirichlet,INLA,Spatial
AI 理解论文
溯源树
样例
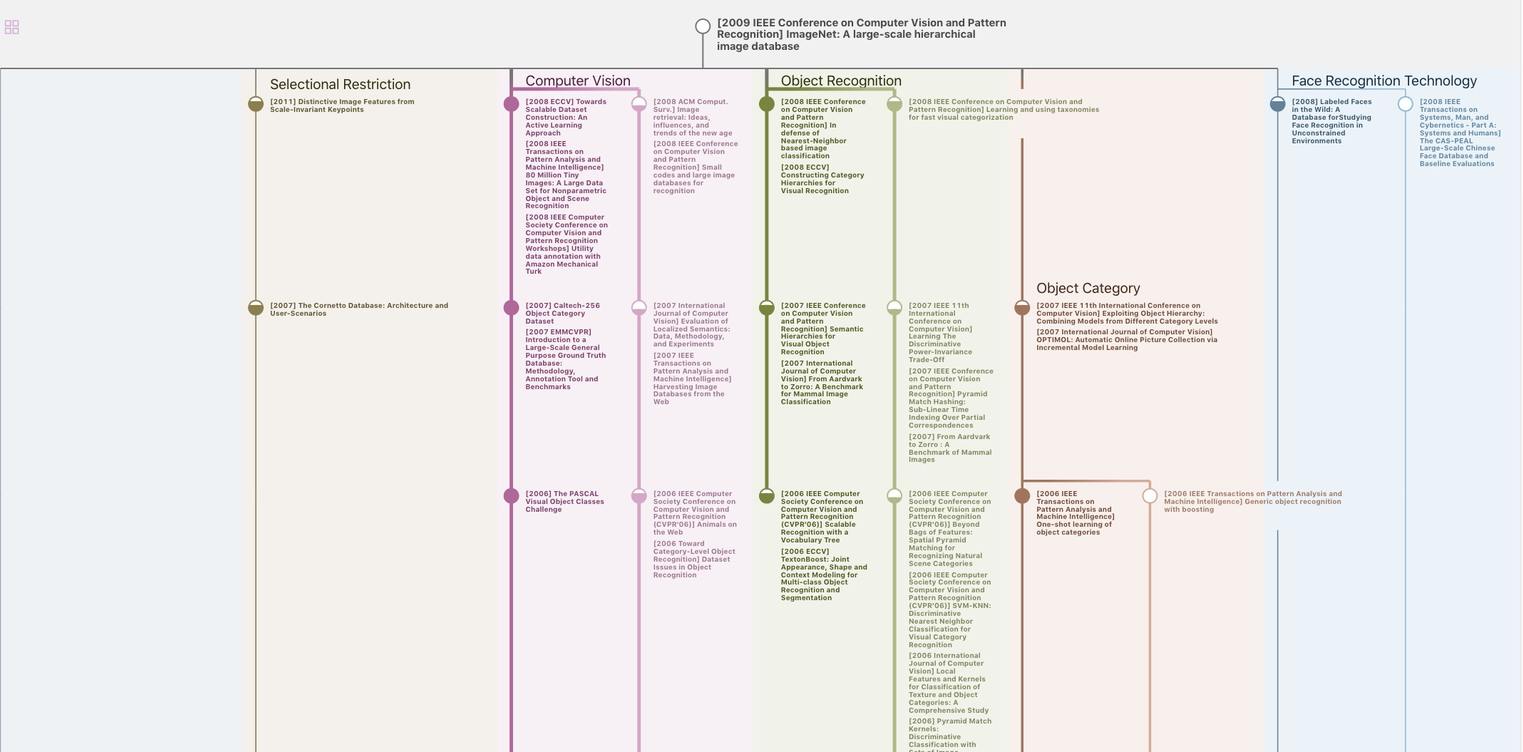
生成溯源树,研究论文发展脉络
Chat Paper
正在生成论文摘要