Class-Constrained t-SNE: Combining Data Features and Class Probabilities.
CoRR(2024)
摘要
Data features and class probabilities are two main perspectives when, e.g., evaluating model results and identifying problematic items. Class probabilities represent the likelihood that each instance belongs to a particular class, which can be produced by probabilistic classifiers or even human labeling with uncertainty. Since both perspectives are multi-dimensional data, dimensionality reduction (DR) techniques are commonly used to extract informative characteristics from them. However, existing methods either focus solely on the data feature perspective or rely on class probability estimates to guide the DR process. In contrast to previous work where separate views are linked to conduct the analysis, we propose a novel approach, class-constrained t-SNE, that combines data features and class probabilities in the same DR result. Specifically, we combine them by balancing two corresponding components in a cost function to optimize the positions of data points and iconic representation of classes - class landmarks. Furthermore, an interactive user-adjustable parameter balances these two components so that users can focus on the weighted perspectives of interest and also empowers a smooth visual transition between varying perspectives to preserve the mental map. We illustrate its application potential in model evaluation and visual-interactive labeling. A comparative analysis is performed to evaluate the DR results.
更多查看译文
关键词
Dimensionality reduction,t-distributed stochastic neighbor embedding,constraint integration
AI 理解论文
溯源树
样例
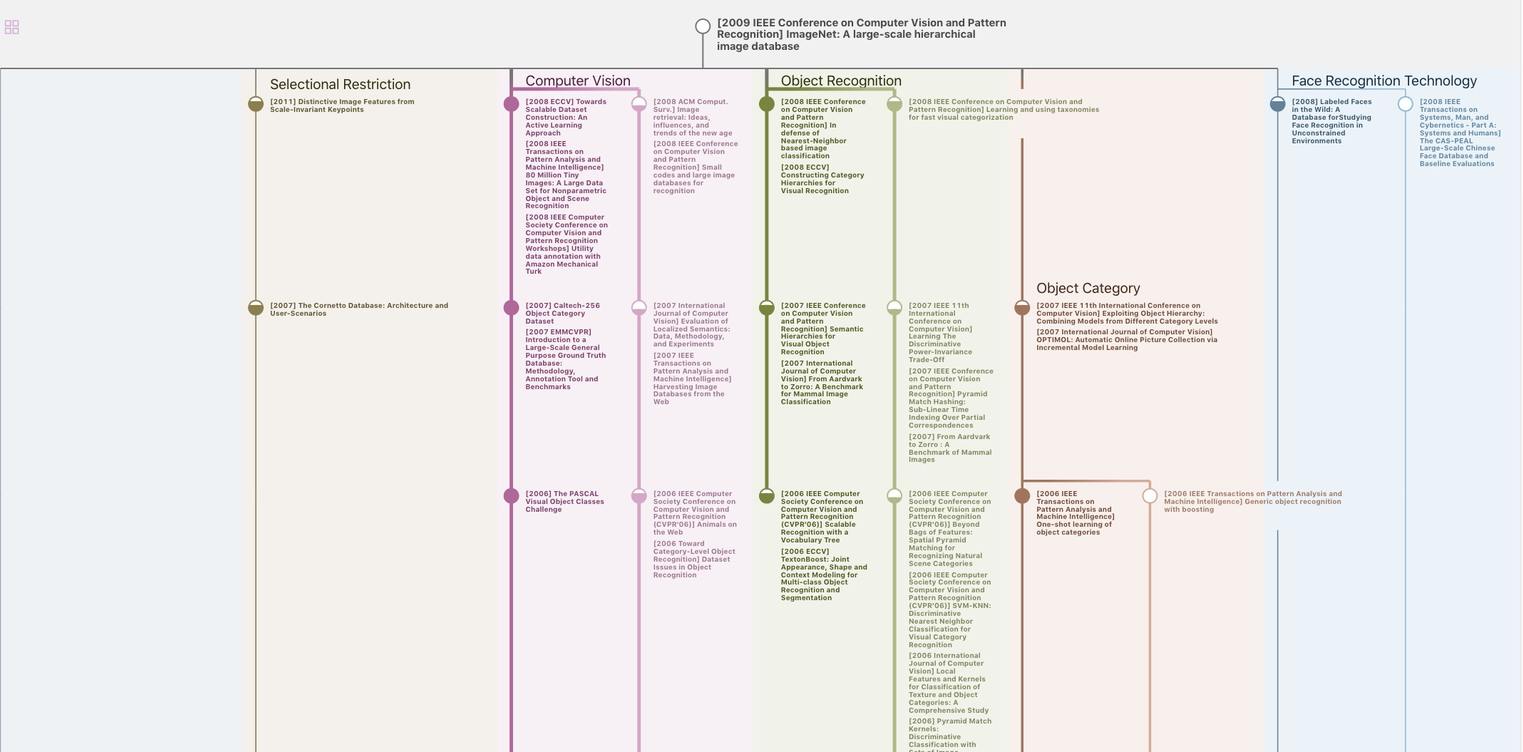
生成溯源树,研究论文发展脉络
Chat Paper
正在生成论文摘要