Deep Learning for Structure-Preserving Universal Stable Koopman-Inspired Embeddings for Nonlinear Canonical Hamiltonian Dynamics
CoRR(2023)
摘要
Discovering a suitable coordinate transformation for nonlinear systems enables the construction of simpler models, facilitating prediction, control, and optimization for complex nonlinear systems. To that end, Koopman operator theory offers a framework for global linearization for nonlinear systems, thereby allowing the usage of linear tools for design studies. In this work, we focus on the identification of global linearized embeddings for canonical nonlinear Hamiltonian systems through a symplectic transformation. While this task is often challenging, we leverage the power of deep learning to discover the desired embeddings. Furthermore, to overcome the shortcomings of Koopman operators for systems with continuous spectra, we apply the lifting principle and learn global cubicized embeddings. Additionally, a key emphasis is paid to enforce the bounded stability for the dynamics of the discovered embeddings. We demonstrate the capabilities of deep learning in acquiring compact symplectic coordinate transformation and the corresponding simple dynamical models, fostering data-driven learning of nonlinear canonical Hamiltonian systems, even those with continuous spectra.
更多查看译文
关键词
embeddings,dynamics,deep learning,structure-preserving,koopman-inspired
AI 理解论文
溯源树
样例
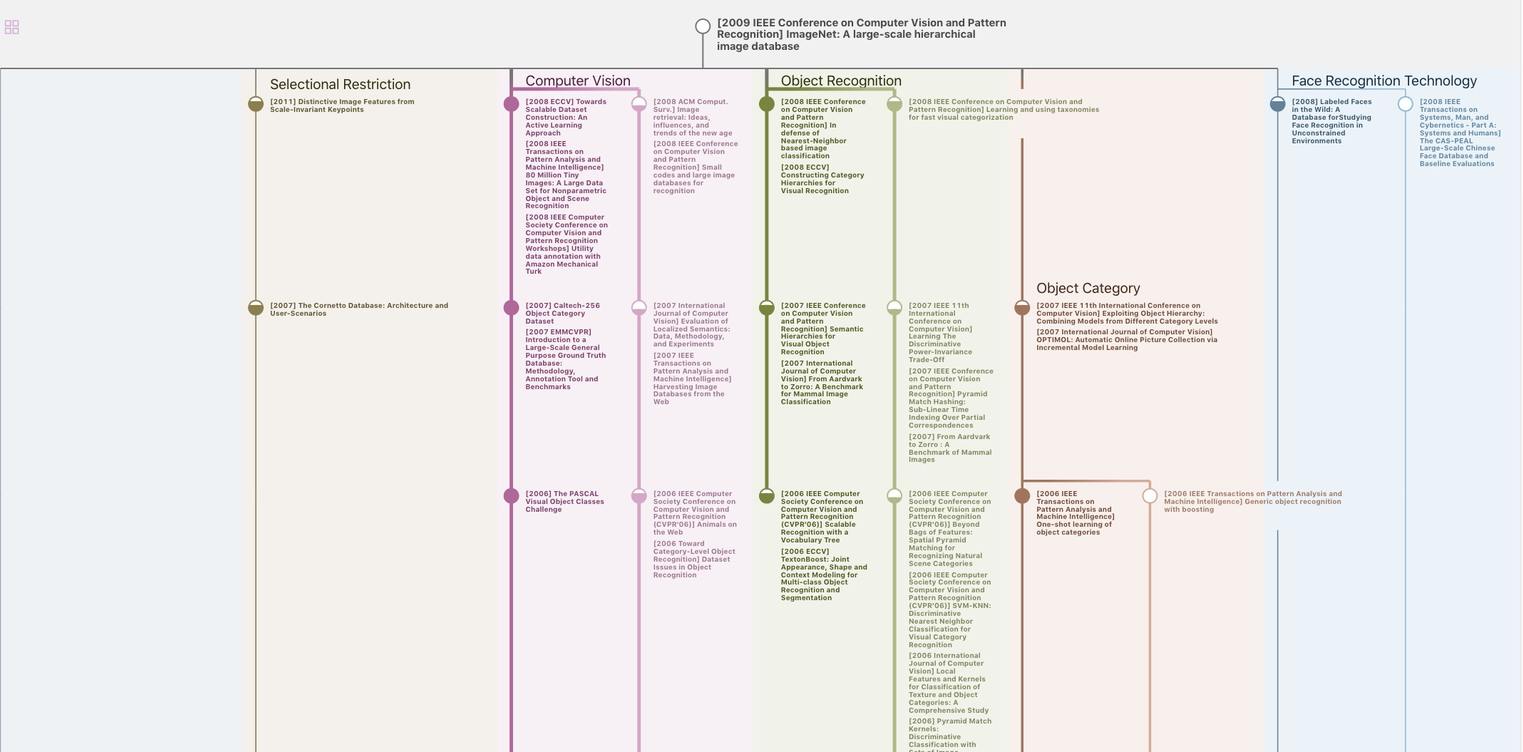
生成溯源树,研究论文发展脉络
Chat Paper
正在生成论文摘要