Causally Sound Priors for Binary Experiments
arXiv (Cornell University)(2023)
摘要
We introduce the BREASE framework for the Bayesian analysis of randomized controlled trials with a binary treatment and a binary outcome. Approaching the problem from a causal inference perspective, we propose parameterizing the likelihood in terms of the baseline risk, efficacy, and side effects of the treatment, along with a flexible, yet intuitive and tractable jointly independent beta prior distribution on these parameters, which we show to be a generalization of the Dirichlet prior for the joint distribution of potential outcomes. Our approach has a number of desirable characteristics when compared to current mainstream alternatives: (i) it naturally induces prior dependence between expected outcomes in the treatment and control groups; (ii) as the baseline risk, efficacy and side effects are quantities inherently familiar to clinicians, the hyperparameters of the prior are directly interpretable, thus facilitating the elicitation of prior knowledge and sensitivity analysis; and (iii) it admits analytical formulae for the marginal likelihood, Bayes factor, and other posterior quantities, as well as exact posterior sampling via simulation, in cases where traditional MCMC fails. Empirical examples demonstrate the utility of our methods for estimation, hypothesis testing, and sensitivity analysis of treatment effects.
更多查看译文
关键词
sound priors,experiments
AI 理解论文
溯源树
样例
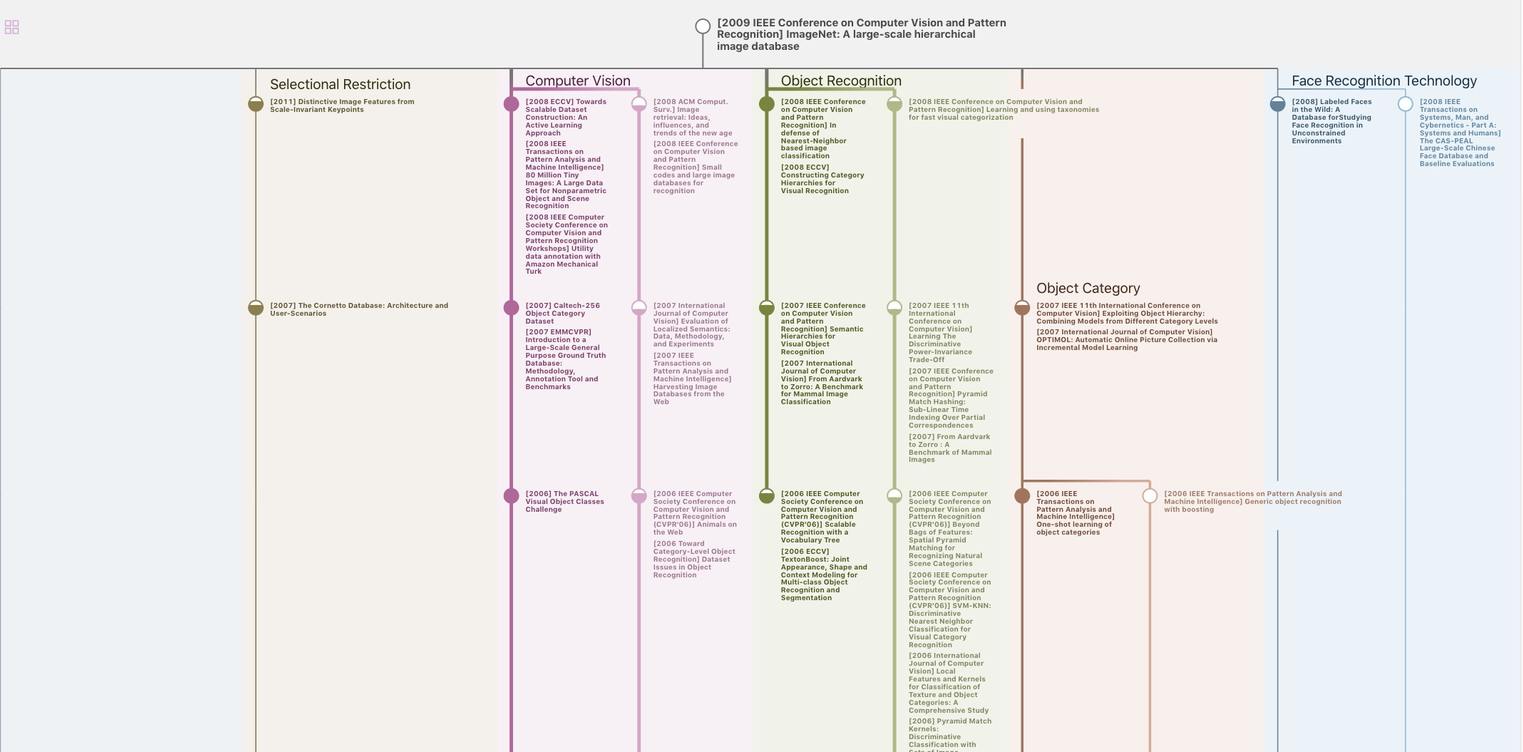
生成溯源树,研究论文发展脉络
Chat Paper
正在生成论文摘要