LLPNet: Graph Autoencoder for Triggering Light Long-Lived Particles at HL-LHC
arXiv (Cornell University)(2023)
摘要
In the search for exotic events involving displaced particles at HL-LHC, the triggering at the level-1 (L1) system will pose a significant challenge. This is particularly relevant in scenarios where low mass long-lived particles (LLPs) are coupled to a Standard Model (SM)-like 125 GeV Higgs boson and they decay into jets. The complexity arises from the low hadronic activity resulting from LLP decay, and the existing triggers' inability to efficiently select displaced events. This study introduces a novel machine learning approach to address this challenge, utilizing a lightweight autoencoder architecture designed for low latency requirements at L1. Focusing on light LLPs with decay lengths ranging from 1 to 100 cm, this approach employs "Edge convolution" on L1 reconstructed tracks. The results show notable signal acceptance at the permissible background rate, primarily originating from minimum bias and QCD di-jet events. For LLPs of mass 10, 30, and 50 GeV at decay length of 5 cm, the signal efficiencies are 33%, 70%, and 80%, respectively. At a 50 cm decay length, these efficiencies are 20%, 39%, and 45% for the same respective masses.
更多查看译文
关键词
graph autoencoder,particles,long-lived,hl-lhc
AI 理解论文
溯源树
样例
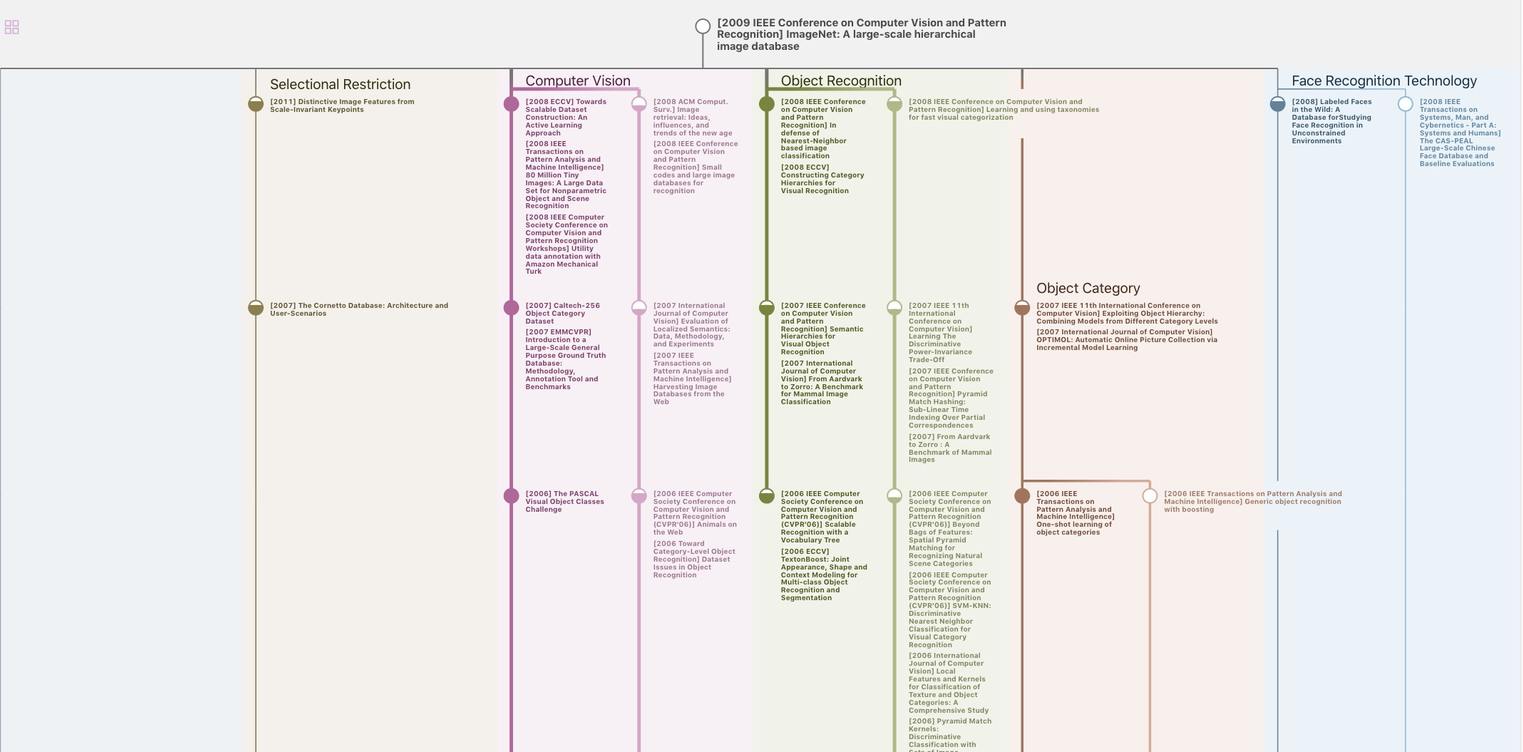
生成溯源树,研究论文发展脉络
Chat Paper
正在生成论文摘要