Multi-Tenant Deep Learning Acceleration with Competitive GPU Resource Sharing
2023 IEEE Cloud Summit(2023)
摘要
As Deep Learning (DL) continues to drive a variety of applications in edge and cloud data centers, co-locating multiple DL models onto the same GPU become widely deployed to improve resource utilization, and achieve acceleration. For example, a self-driving system hosts multiple tasks simultaneously (e.g., detection, classification, segmentation, etc.) and expects concurrent computing on one single device. However, our analysis demonstrates that, when deploying compound DNN models for multiple tenants on a GPU, certain issues arise: As different models' structure heterogeneities and skewed data distributions, corresponding models cause highly imbalanced computing workloads. However, current GPU scheduling methods lack effective resource allocations. To address these issues, we propose a novel resource allocation method - competitive resource sharing, which is beneficial for parallel model executions, and the proposed concept of "virtual resource" could effectively characterize and guide the practical per-task resource utilization and allocation. Our experiments demonstrate that the DNN computing throughput could be significantly escalated by 2.16x similar to 2.80x in various multitenant scenarios.
更多查看译文
关键词
Resource-Allocation,GPU-Computing,Multi-tenant-DNN
AI 理解论文
溯源树
样例
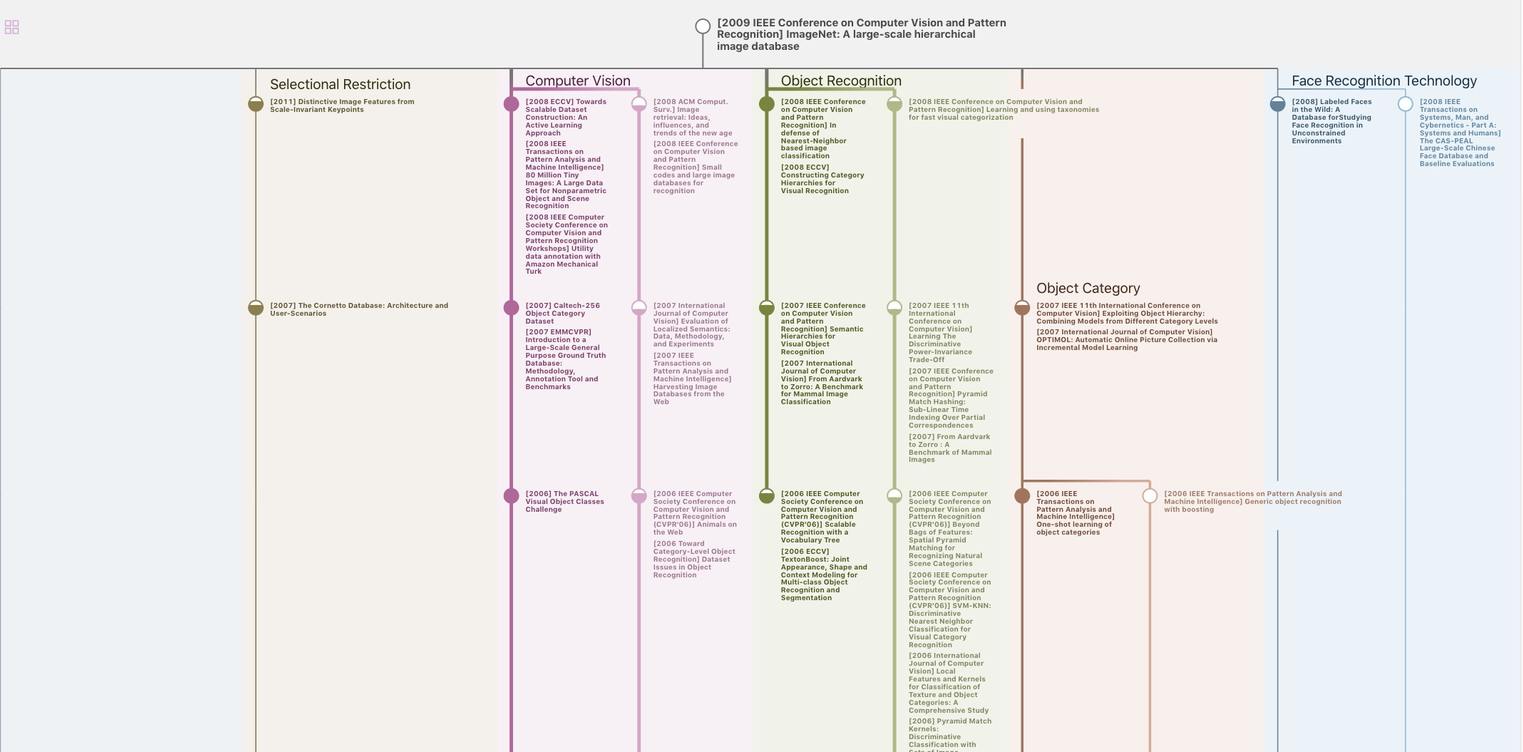
生成溯源树,研究论文发展脉络
Chat Paper
正在生成论文摘要