Using domain adaptation for classification of healthy and disease conditions from mobile-captured images of standard 12-lead electrocardiograms
Scientific Reports(2023)
摘要
12-lead electrocardiogram (ECG) recordings can be collected in any clinic and the interpretation is performed by a clinician. Modern machine learning tools may make them automatable. However, a large fraction of 12-lead ECG data is still available in printed paper or image only and comes in various formats. To digitize the data, smartphone cameras can be used. Nevertheless, this approach may introduce various artifacts and occlusions into the obtained images. Here we overcome the challenges of automating 12-lead ECG analysis using mobile-captured images and a deep neural network that is trained using a domain adversarial approach. The net achieved an average 0.91 receiver operating characteristic curve on tested images captured by a mobile device. Assessment on image from unseen 12-lead ECG formats that the network was not trained on achieved high accuracy. We further show that the network accuracy can be improved by including a small number of unlabeled samples from unknown formats in the training data. Finally, our models also achieve high accuracy using signals as input rather than images. Using a domain adaptation approach, we successfully classified cardiac conditions on images acquired by a mobile device and showed the generalizability of the classification using various unseen image formats.
更多查看译文
关键词
domain adaptation,classification,mobile-captured
AI 理解论文
溯源树
样例
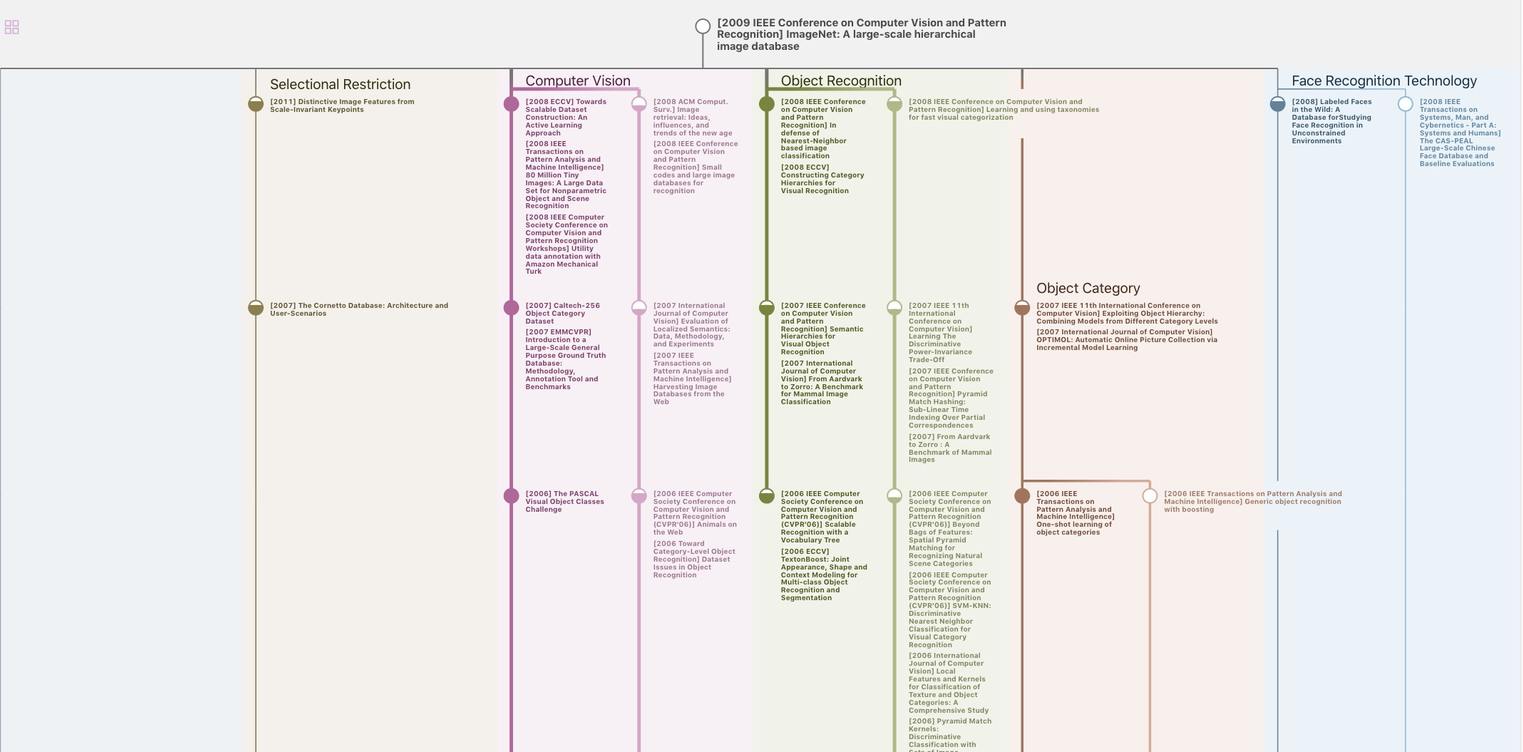
生成溯源树,研究论文发展脉络
Chat Paper
正在生成论文摘要