Deep learning model for personalized prediction of positive MRSA culture using time-series electronic health records
Nature Communications(2024)
摘要
Methicillin-resistant Staphylococcus aureus (MRSA) poses significant morbidity and mortality in hospitals. Rapid, accurate risk stratification of MRSA is crucial for optimizing antibiotic therapy. Our study introduced a deep learning model, PyTorch_EHR, which leverages electronic health record (EHR) time-series data, including wide-variety patient specific data, to predict MRSA culture positivity within two weeks. 8,164 MRSA and 22,393 non-MRSA patient events from Memorial Hermann Hospital System, Houston, Texas are used for model development. PyTorch_EHR outperforms logistic regression (LR) and light gradient boost machine (LGBM) models in accuracy (AUROC PyTorch_EHR = 0.911, AUROC LR = 0.857, AUROC LGBM = 0.892). External validation with 393,713 patient events from the Medical Information Mart for Intensive Care (MIMIC)-IV dataset in Boston confirms its superior accuracy (AUROC PyTorch_EHR = 0.859, AUROC LR = 0.816, AUROC LGBM = 0.838). Our model effectively stratifies patients into high-, medium-, and low-risk categories, potentially optimizing antimicrobial therapy and reducing unnecessary MRSA-specific antimicrobials. This highlights the advantage of deep learning models in predicting MRSA positive cultures, surpassing traditional machine learning models and supporting clinicians’ judgments.
更多查看译文
AI 理解论文
溯源树
样例
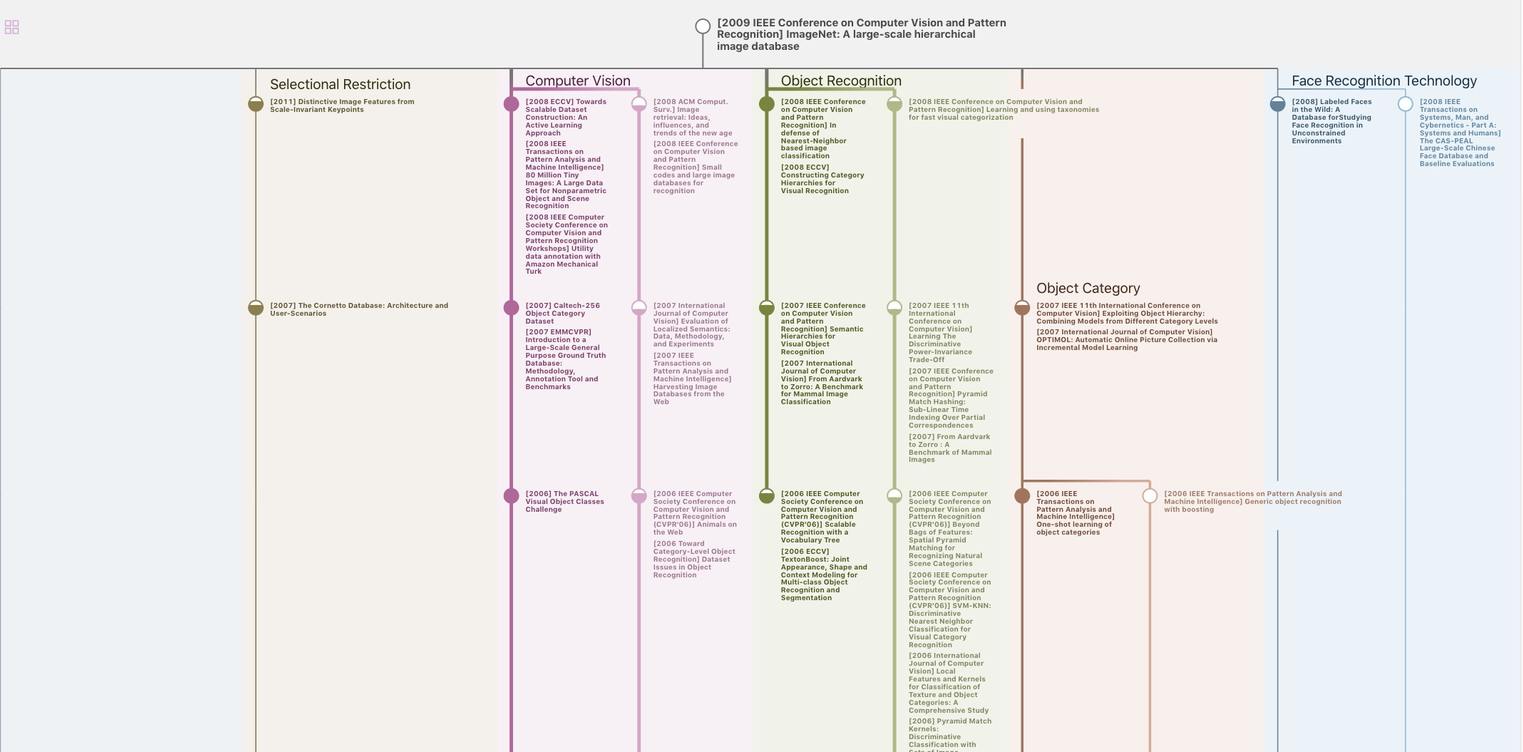
生成溯源树,研究论文发展脉络
Chat Paper
正在生成论文摘要