AI Segmentation of Vestibular Schwannomas with Radiomic Analysis and Clinical Correlates
medrxiv(2023)
摘要
Vestibular schwannoma (VS) is a benign, slow growing tumor that may affect hearing and balance. It accounts for 7-8% of all primary brain tumors. Gamma knife radiosurgery (GKRS) is a common treatment option for VS. Magnetic resonance imaging (MRI) is employed for diagnosis, surgery planning, and follow-up of VS. Long-term follow-up determines efficacy of VS treatment. Identifying MRI-derived markers to improve management of VS is challenging. This study describes MRI processing pipeline that automatically segments VS and investigates stability and outcome predictive power of radiomic MRI features.
We first preprocessed and segmented available pre-GKRS T1-weighted post-contrast MRI images in VS patients, using a Convolutional Neural Network (CNN) developed on DeepMedic framework. Then, we compared CNN and manual segmentations, extracted radiomic features from both manual and CNN segmentations of VS, and, finally, evaluated robustness of extracted features and clinical outcome analyses based thereof.
We found that homogeneity, robust maximum intensity and sphericity were the most robust across segmentations. We also found that maximum and minimum intensities were most predictive of tumor growth across all segmentation methods and subject cohorts. We used retrospective post-GK SRS data collected in our institution to build the processing pipeline for unsupervised segmenting of VS. This pipeline is released as a Docker image integrated with XNAT (extensible neuroimaging archive toolkit), an established open research imaging database platform[15][1]. Generated segmentations can be viewed and edited in the XNAT-based online OHIF (Open Health Imaging Foundation) viewer[16][2] in real time.
### Competing Interest Statement
Albert Kim is a consultant for Monteris Medical.
### Funding Statement
This work was partially funded by NCI grant U24CA204854 aimed at developing data and knowledge management platforms to support the collection, analysis, and sharing of imaging and related data for use in cancer research.
### Author Declarations
I confirm all relevant ethical guidelines have been followed, and any necessary IRB and/or ethics committee approvals have been obtained.
Yes
The details of the IRB/oversight body that provided approval or exemption for the research described are given below:
IRB of Washington University in Saint Louis gave ethical approval for this work.
I confirm that all necessary patient/participant consent has been obtained and the appropriate institutional forms have been archived, and that any patient/participant/sample identifiers included were not known to anyone (e.g., hospital staff, patients or participants themselves) outside the research group so cannot be used to identify individuals.
Yes
I understand that all clinical trials and any other prospective interventional studies must be registered with an ICMJE-approved registry, such as ClinicalTrials.gov. I confirm that any such study reported in the manuscript has been registered and the trial registration ID is provided (note: if posting a prospective study registered retrospectively, please provide a statement in the trial ID field explaining why the study was not registered in advance).
Yes
I have followed all appropriate research reporting guidelines, such as any relevant EQUATOR Network research reporting checklist(s) and other pertinent material, if applicable.
Yes
All data produced in the present study are available upon reasonable request to the authors
* VS
: Vestibular Schwannoma
MGA
: Multimodal Glioma Analysis
GK
: Gamma Knife
QC
: Quality Control
NCR
: necrosis
ENH
: enhancement
ROI
: region of interest
FOV
: field of view
nogt-63
: no gamma knife contour
CNN
: convolutional neural network
gt
: gamma knife
DM
: DeepMedic
[1]: #ref-15
[2]: #ref-16
更多查看译文
关键词
vestibular schwannomas
AI 理解论文
溯源树
样例
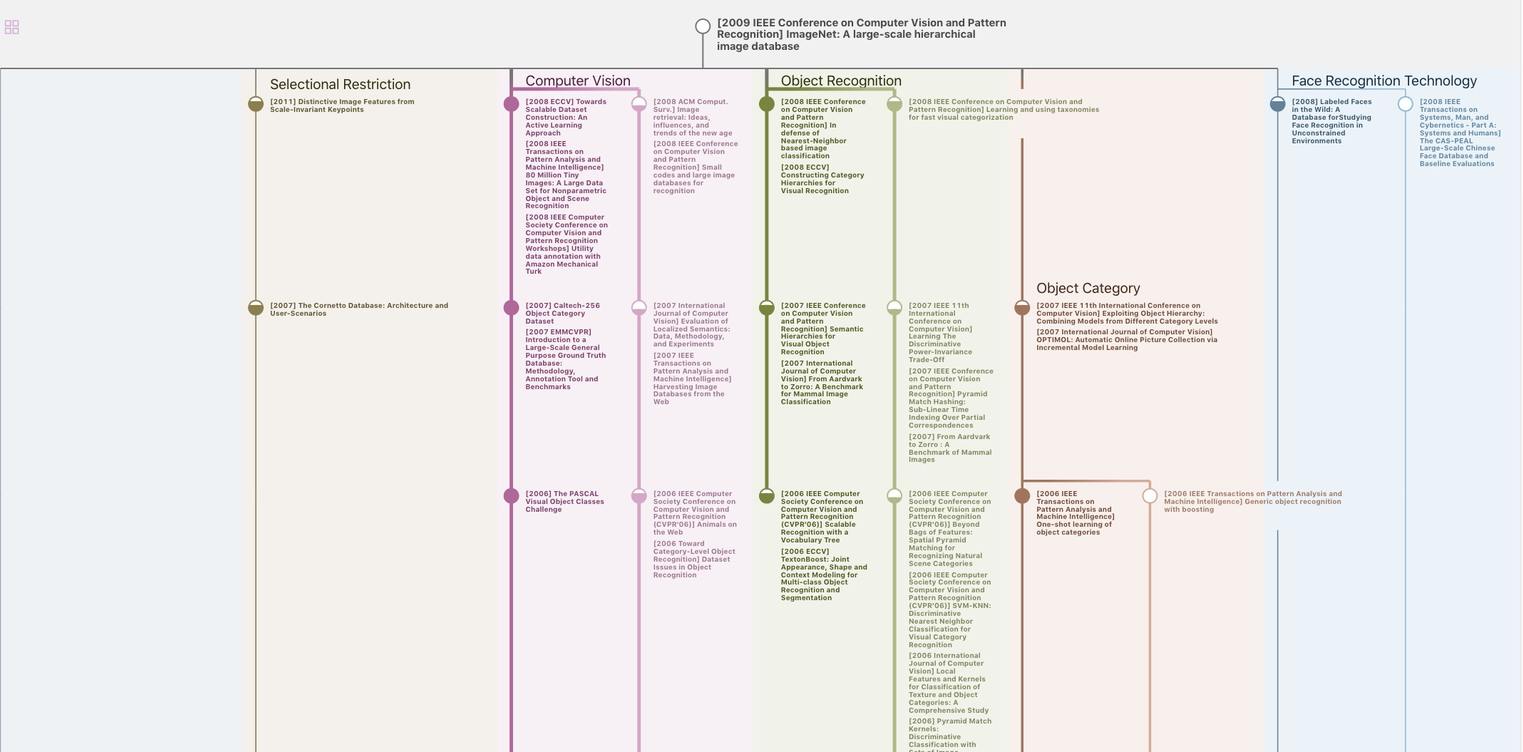
生成溯源树,研究论文发展脉络
Chat Paper
正在生成论文摘要