Predicting Huntington’s disease state with ensemble learning & sMRI: more than just the striatum
medRxiv (Cold Spring Harbor Laboratory)(2023)
摘要
Developing effective treatments for Huntington’s disease (HD) requires reliable markers of disease progression. Striatal atrophy has been the hallmark of HD progression, but volumetric anomalies are also found in other brain regions. Little is known about the potential increase in predictive biomarking accuracy when volumetric scores from multiple brain regions are combined to predict the HD status of individual participants. We used cross-sectional structural MRI data from 184 HD gene-positive participants to a) test a novel ensemble machine learning model in classifying participants in one of four HD progression states (PreHD A; PreHD B; HD1; HD2), and (b) identify the brain regions that carry HD biomarking signal from 15 regions. We used 5-fold cross validation and backward feature elimination to find the optimal predictors and investigated the stability of the findings through repeated analyses. The ensemble predictive model systematically matched or outperformed the accuracy of nine standard machine learning models, reaching 55.3%±6.1 balanced accuracy in 4-group classification. The accuracy was higher for binary classifications (PreHD vs HD: 83.3%±6.3; PreHD A vs PreHD B: 76.7%±8.0; PreHD B vs HD1: 75.9%±8.5; HD1 vs HD2: 70.9%±9.4). Striatal structures (caudate and putamen) were systematically found to be top predictors. However, the accuracy increased substantially when we included other regions in the model (e.g., occipital cortex, lateral ventricles, cingulate, temporal lobe). Optimal models frequently included 2-7 brain regions from different areas. Overall, the accuracy of classifications remained stable across repetitions but the list of selected brain regions could vary, likely due to collinearities in volumetric scores. This is the first study to demonstrate the improvement of classification accuracy when predicting HD progression with a stacked ensemble model. Our findings indicate that HD progression is marked not only by striatal atrophy but also by volumetric changes outside the striatum, without which biomarking models cannot achieve optimal results. The robust methods applied here exposed instability in the selection of brain regions despite the sizeable sample size (n=184); such instabilities could lead to different conclusions in different studies when single analyses are applied on smaller sample sizes. From a translational perspective, our study informs on the selection of candidate endpoints or target regions for therapeutic intervention in future clinical trials.
### Competing Interest Statement
The authors have declared no competing interest.
### Funding Statement
MK was supported by a grant from CHDI Foundation (A-15920). DCA was supported by funding from the European Unions Horizon 2020 research & innovation programme under grant agreement number 666992 and from NIHR UCLH Biomedical Research Centre. RIS and SJT were supported by funding from the Wellcome Trust (200181/Z/15/Z). SJT is also partly supported by the UK Dementia Research Institute that receives its funding from DRI Ltd., the UK Medical Research Council, Alzheimer's Society, and Alzheimer's Research UK. PAW was supported by the MRC Skills Development Fellowship (MR/T027770/1). TRACK-HD was funded by CHDI foundation.
### Author Declarations
I confirm all relevant ethical guidelines have been followed, and any necessary IRB and/or ethics committee approvals have been obtained.
Yes
The details of the IRB/oversight body that provided approval or exemption for the research described are given below:
The Commissie Medische Ethiek H1-Q of Leids Universitair Medisch Centrum, the National Hospital for Neurology and Neurosurgery and Institute of Neurology Joint Research Ethics Committee for University College London, the Groupe Hospitalier Pitie-Salpetriere Ethics Committee for Hospitalier Pitie-Salpetriere Paris and the UBC Clinical Research Ethics Board of the University of British Columbia all gave ethical approval for this study to be conducted at their respective sites.
I confirm that all necessary patient/participant consent has been obtained and the appropriate institutional forms have been archived, and that any patient/participant/sample identifiers included were not known to anyone (e.g., hospital staff, patients or participants themselves) outside the research group so cannot be used to identify individuals.
Yes
I understand that all clinical trials and any other prospective interventional studies must be registered with an ICMJE-approved registry, such as ClinicalTrials.gov. I confirm that any such study reported in the manuscript has been registered and the trial registration ID is provided (note: if posting a prospective study registered retrospectively, please provide a statement in the trial ID field explaining why the study was not registered in advance).
Yes
I have followed all appropriate research reporting guidelines, such as any relevant EQUATOR Network research reporting checklist(s) and other pertinent material, if applicable.
Yes
更多查看译文
关键词
huntingtons,ensemble learning,predicting,disease state
AI 理解论文
溯源树
样例
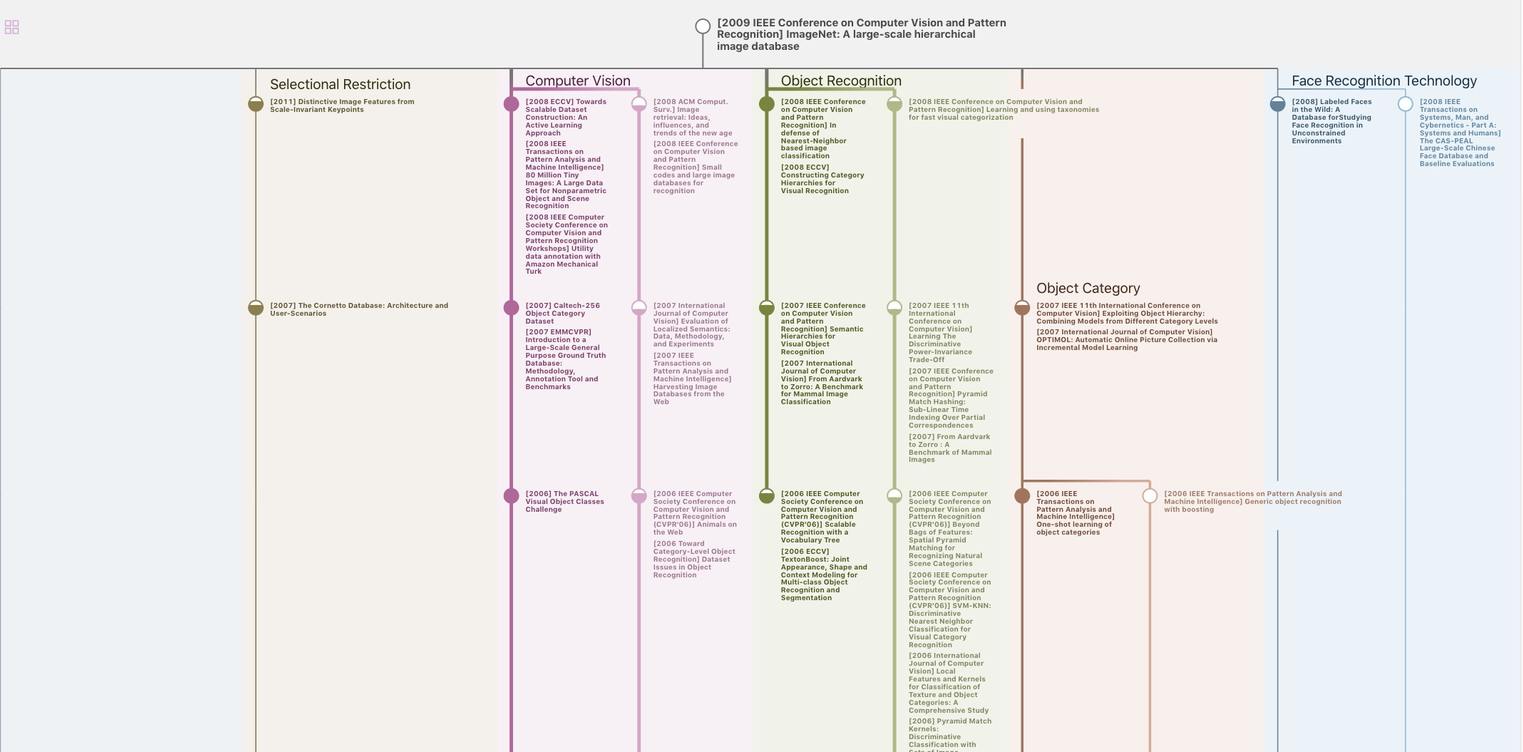
生成溯源树,研究论文发展脉络
Chat Paper
正在生成论文摘要