Connected Hyperbolic Complex Networks
2022 International Conference on Computational Science and Computational Intelligence (CSCI)(2022)
摘要
Generative models play an important role in analyzing and predicting relevant characteristics of real-world complex networks. The predictive power of these models lies in the possibility that large amount of synthetic networks, similar to real ones at some extent, can be produced, and by analyzing them statistically, significant inferences can be performed. Synthetic networks, based on the hyperbolic geometry, turned out to be good generative models of real-world networks, as they reproduce several macroscopic behaviours of real networks and can help in assessing the scalability of new network functions. The original hyperbolic generative model by Krioukov et al. [1] lacks an important property: it can not guarantee with 100% probability that the resulting synthetic network is connected. In other words for some parameter regions, the networks fall into fragments resulting in disconnected regions of subgraphs. Inevitably, from the viewpoint of modeling real networks, the connectedness is an expected property. In this paper we show that, with a slight extension of the original generation rule of the hyperbolic networks, the connectedness can always be ensured. We also present analytical and numerical results which show that there will be no significant changes in the macroscopic properties of networks, like average degree and degree distribution.
更多查看译文
关键词
complex networks,hyperbolic geometry
AI 理解论文
溯源树
样例
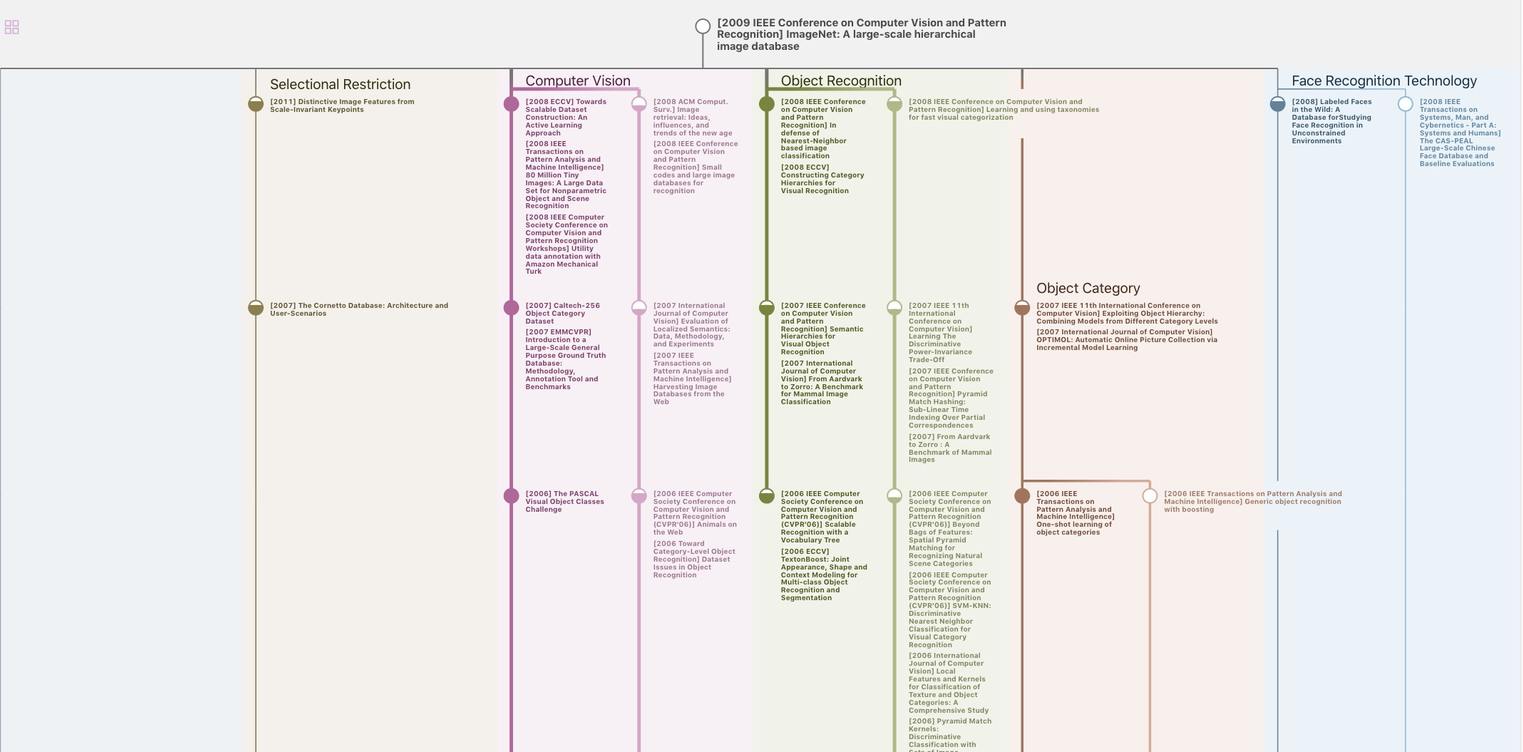
生成溯源树,研究论文发展脉络
Chat Paper
正在生成论文摘要