Effects of Selection Bias on Online Adversarial Aware SVM When Facing an Evasion Attack
2022 International Conference on Computational Science and Computational Intelligence (CSCI)(2022)
摘要
In evasion attacks, the success of machine learning (ML) depends on its ability to detect an attack in an adversarial setting. It is important that these ML models are trained regularly to keep abreast with evolving evasion attacks. We present an investigation of the effects of selection bias and class imbalance in training an Adversary-Aware Online SVM (AAOSVM). We show that incorrect samples can compromise the model's ability to detect attacks. Comparison results showed better True Positive Rates (TPR) performance than the Online Support Vector Machine (OSVM), and that AAOSVM was sensitive to selection bias but not to class imbalance.
更多查看译文
关键词
Adversary-Aware,Machine Learning,Support Vector Machine,Bias,Phishing
AI 理解论文
溯源树
样例
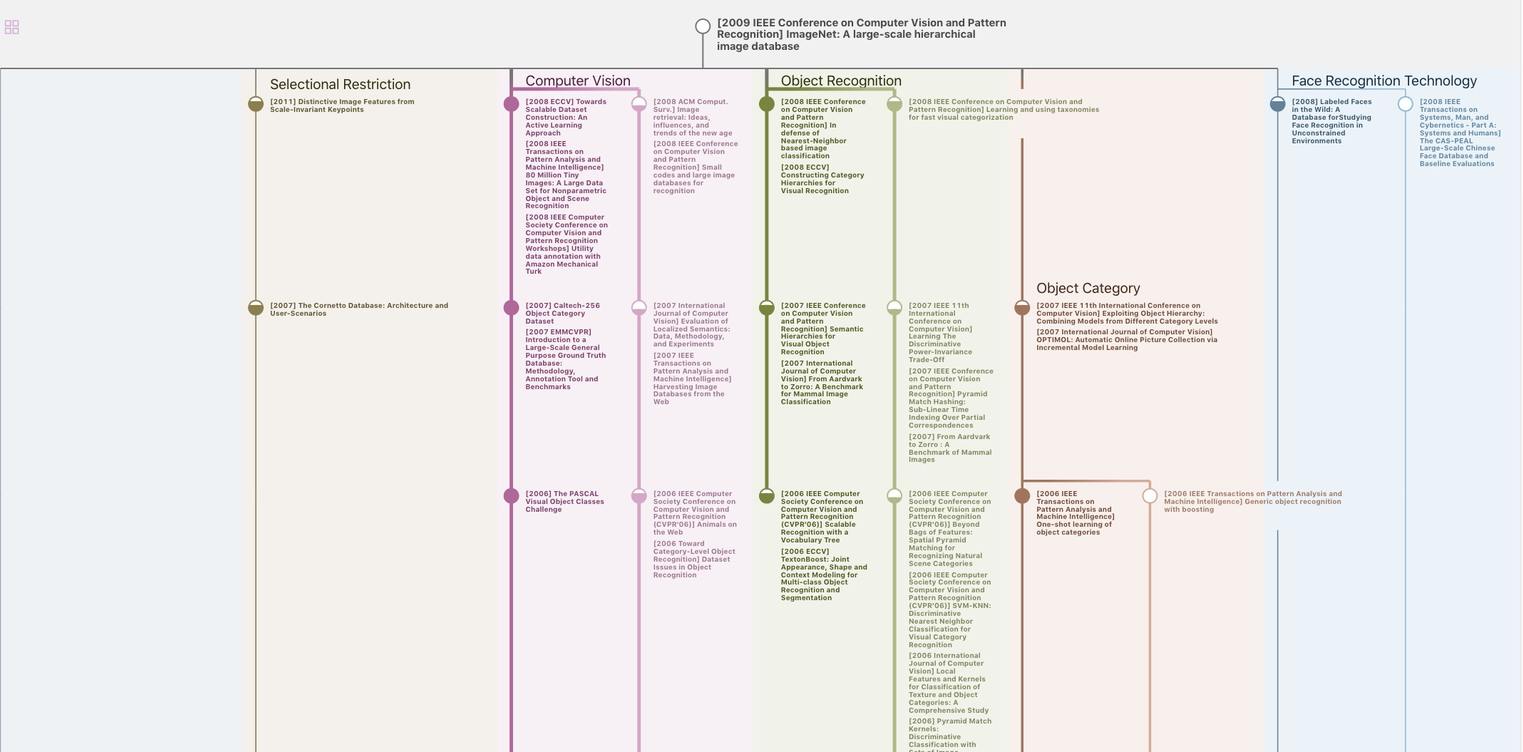
生成溯源树,研究论文发展脉络
Chat Paper
正在生成论文摘要