Deep learning-based detection and classification of multi-leaf collimator modeling errors in volumetric modulated radiation therapy
JOURNAL OF APPLIED CLINICAL MEDICAL PHYSICS(2023)
摘要
Purpose: The purpose of this study was to create and evaluate deep learning-based models to detect and classify errors of multi-leaf collimator (MLC) modeling parameters in volumetric modulated radiation therapy (VMAT), namely the transmission factor (TF) and the dosimetric leaf gap (DLG).Methods: A total of 33 clinical VMAT plans for prostate and head-and-neck cancer were used, assuming a cylindrical and homogeneous phantom, and error plans were created by altering the original value of the TF and the DLG by +/- 10, 20, and 30% in the treatment planning system (TPS). The Gaussian filters of sigma = 0.5 and 1.0 were applied to the planar dose maps of the error-free plan to mimic the measurement dose map, and thus dose difference maps between the error-free and error plans were obtained. We evaluated 3 deep learning-based models, created to perform the following detections/classifications: (1) error-free versus TF error, (2) error-free versus DLG error, and (3) TF versus DLG error. Models to classify the sign of the errors were also created and evaluated. A gamma analysis was performed for comparison.Results: The detection and classification of TF and DLG error were feasible for sigma = 0.5; however, a considerable reduction of accuracy was observed for sigma=1.0 depending on the magnitude of error and treatment site. The sign of errors was detectable by the specifically trained models for sigma = 0.5 and 1.0. The gamma analysis could not detect errors.Conclusions: We demonstrated that the deep learning-based models could feasibly detect and classify TF and DLG errors in VMAT dose distributions, depending on the magnitude of the error, treatment site, and the degree of mimicked measurement doses.
更多查看译文
关键词
deep learning‐based,radiation,volumetric
AI 理解论文
溯源树
样例
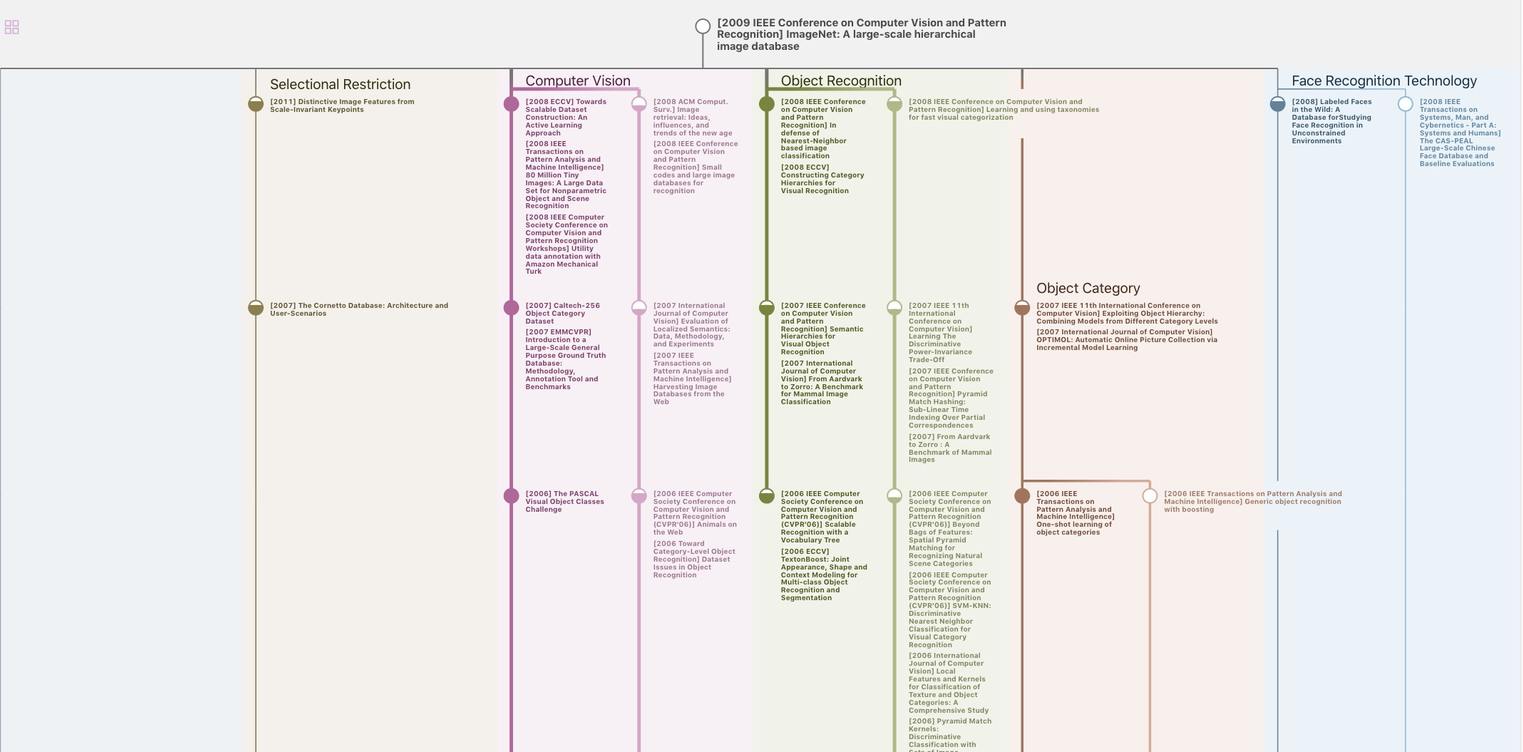
生成溯源树,研究论文发展脉络
Chat Paper
正在生成论文摘要