A Game of Bundle Adjustment -- Learning Efficient Convergence
CoRR(2023)
摘要
Bundle adjustment is the common way to solve localization and mapping. It is an iterative process in which a system of non-linear equations is solved using two optimization methods, weighted by a damping factor. In the classic approach, the latter is chosen heuristically by the Levenberg-Marquardt algorithm on each iteration. This might take many iterations, making the process computationally expensive, which might be harmful to real-time applications. We propose to replace this heuristic by viewing the problem in a holistic manner, as a game, and formulating it as a reinforcement-learning task. We set an environment which solves the non-linear equations and train an agent to choose the damping factor in a learned manner. We demonstrate that our approach considerably reduces the number of iterations required to reach the bundle adjustment's convergence, on both synthetic and real-life scenarios. We show that this reduction benefits the classic approach and can be integrated with other bundle adjustment acceleration methods.
更多查看译文
关键词
bundle adjustment,efficient convergence,learning
AI 理解论文
溯源树
样例
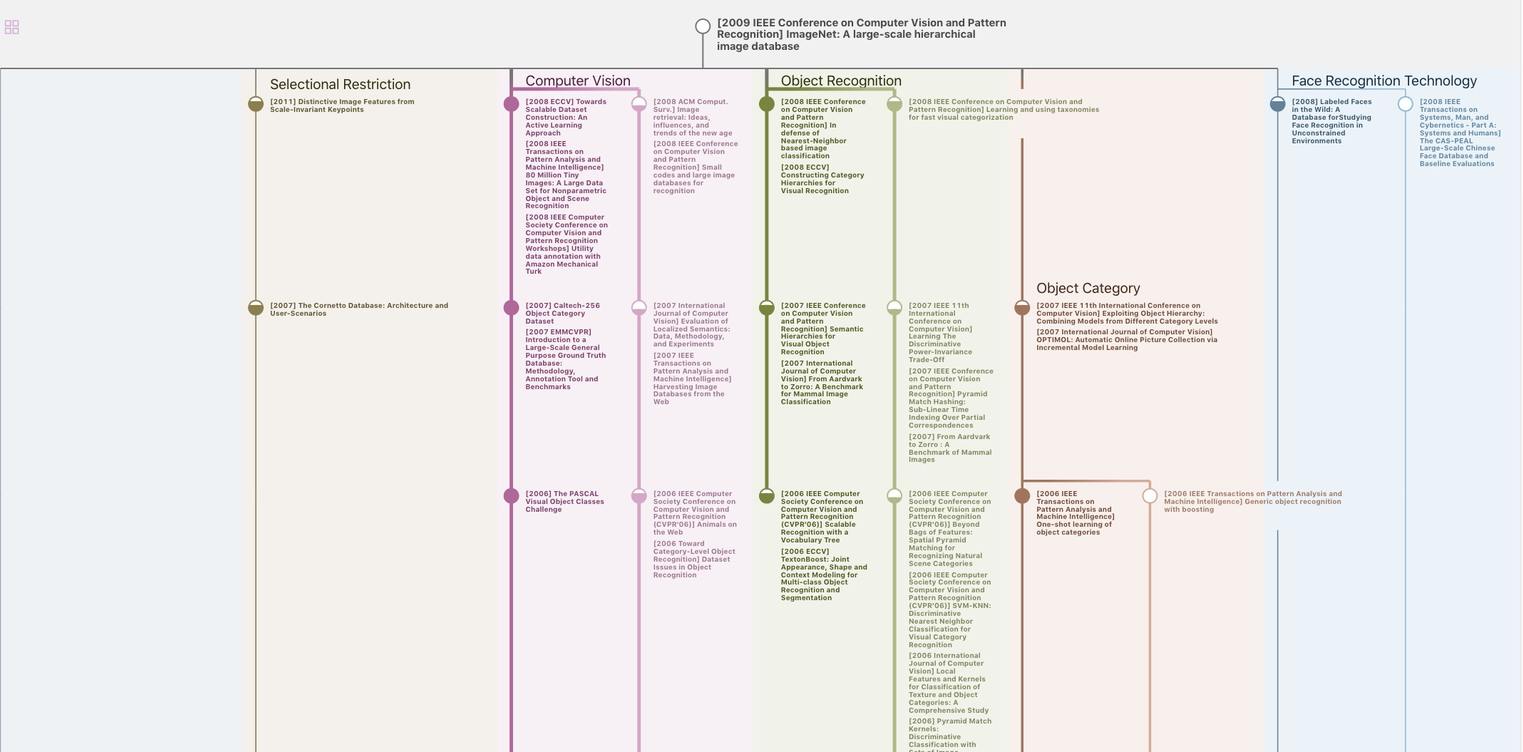
生成溯源树,研究论文发展脉络
Chat Paper
正在生成论文摘要