Bayesian Low-rank Adaptation for Large Language Models
ICLR 2024(2023)
摘要
Low-rank adaptation (LoRA) has emerged as a new paradigm for cost-efficient
fine-tuning of large language models (LLMs). However, fine-tuned LLMs often
become overconfident especially when fine-tuned on small datasets. Bayesian
methods, with their inherent ability to estimate uncertainty, serve as potent
tools to mitigate overconfidence and enhance calibration. In this work, we
introduce Laplace-LoRA, which applies a Bayesian approach to the LoRA
parameters. Specifically, Laplace-LoRA applies a Laplace approximation to the
posterior over the LoRA parameters, considerably improving the calibration of
fine-tuned LLMs.
更多查看译文
关键词
Large language models,Bayesian deep learning,Laplace approximation,uncertainty calibration
AI 理解论文
溯源树
样例
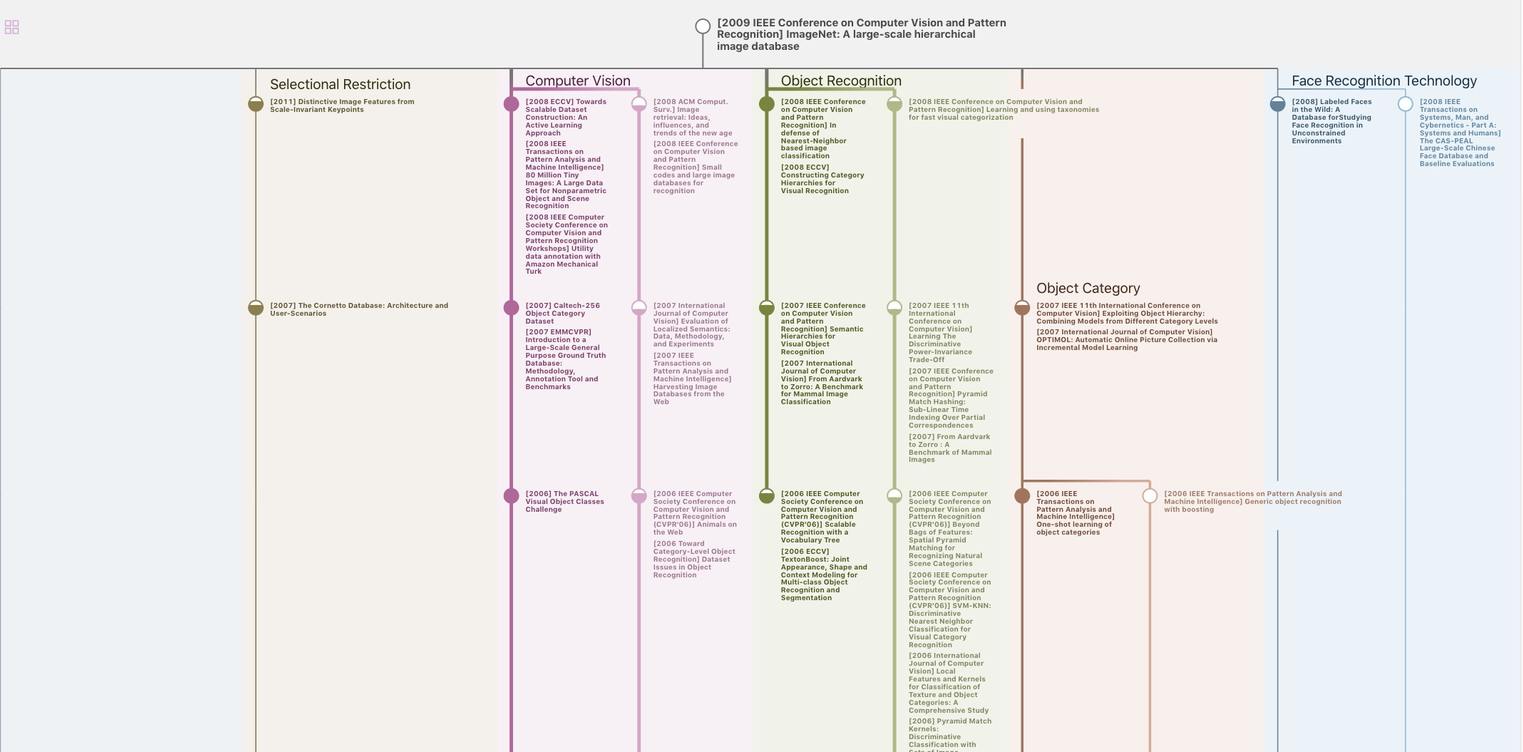
生成溯源树,研究论文发展脉络
Chat Paper
正在生成论文摘要