Training Neural Networks with Universal Adiabatic Quantum Computing
CoRR(2023)
摘要
The training of neural networks (NNs) is a computationally intensive task requiring significant time and resources. This paper presents a novel approach to NN training using Adiabatic Quantum Computing (AQC), a paradigm that leverages the principles of adiabatic evolution to solve optimisation problems. We propose a universal AQC method that can be implemented on gate quantum computers, allowing for a broad range of Hamiltonians and thus enabling the training of expressive neural networks. We apply this approach to various neural networks with continuous, discrete, and binary weights. Our results indicate that AQC can very efficiently find the global minimum of the loss function, offering a promising alternative to classical training methods.
更多查看译文
关键词
quantum,neural networks,computing
AI 理解论文
溯源树
样例
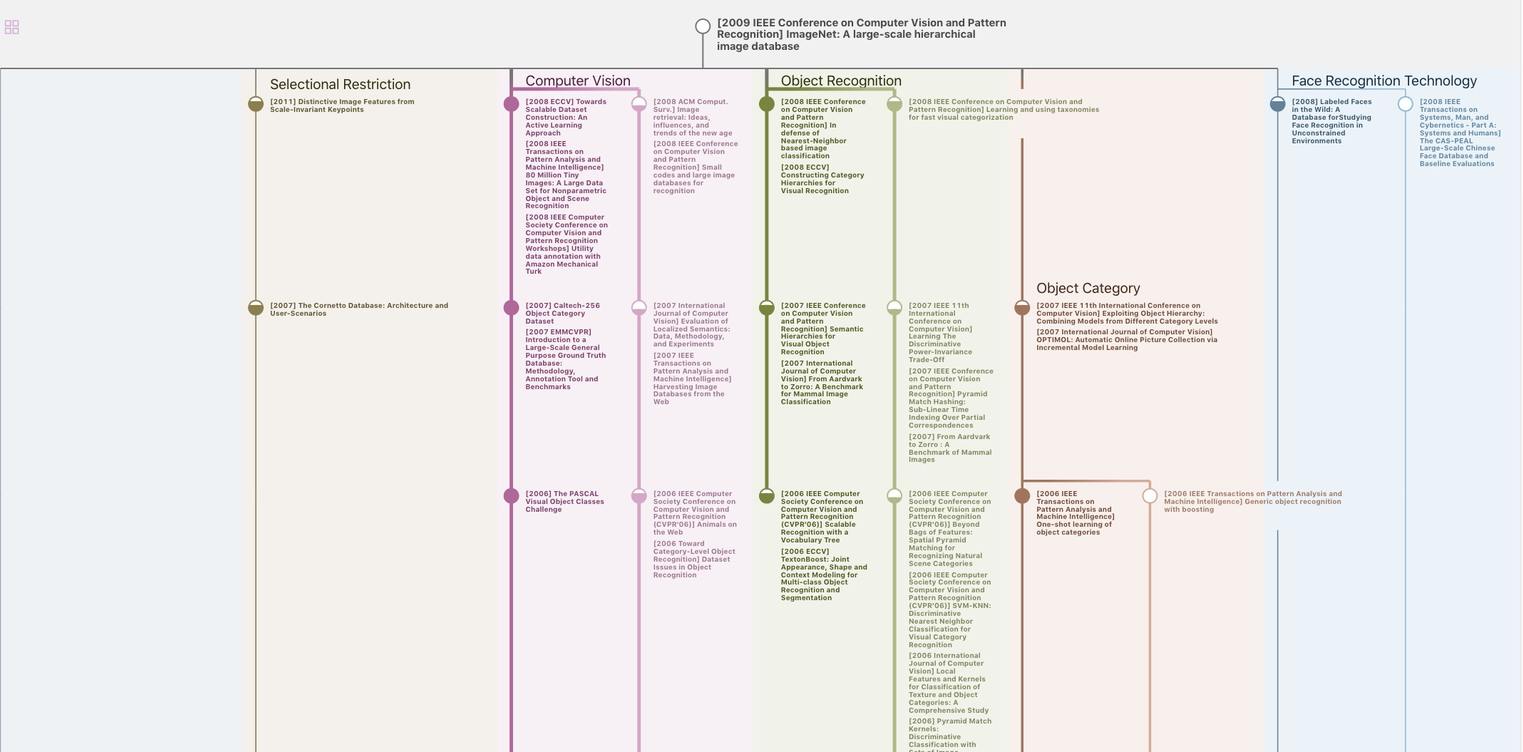
生成溯源树,研究论文发展脉络
Chat Paper
正在生成论文摘要