Dilated Convolution Network for Road Damage Detection
2023 3rd International Symposium on Computer Technology and Information Science (ISCTIS)(2023)
摘要
Road damage resulting from various issues poses a significant risk to traffic safety, which underscores the importance of timely and accurate detection and repair of road defects. Deep learning methods have made significant advancements in road damage detection due to their ability to automatically learn and adapt. The majority of them, however, rely on conventional convolution networks that do not provide precise location information during feature extraction, leading to inaccurate detections. Therefore, we propose a multi-scale convolution network for detecting road damage. After multiple layers of convolution, target location information obtained by depth features becomes sparse. We use the last three stages of multi-scale features for fusion and perform joint up sampling after regular convolution. The fusion of multi-scale features retains rich semantic and location information, while combining depth-separated convolution to reduce model computational complexity. For the attention calculation stage, a combination of channel attention and spatial attention is employed in order to eliminate irrelevant background factors and determine the edge contours of the damaged area accurately. We compare seven mainstream deep learning methods using the CPRID dataset. According to visualization comparisons and quantitative metrics, our network outperforms several current state-of-the-art methods.
更多查看译文
关键词
Deep learning,Semantic segmentation,Road damage detection,Convolutional neural networks,Attention mechanisms
AI 理解论文
溯源树
样例
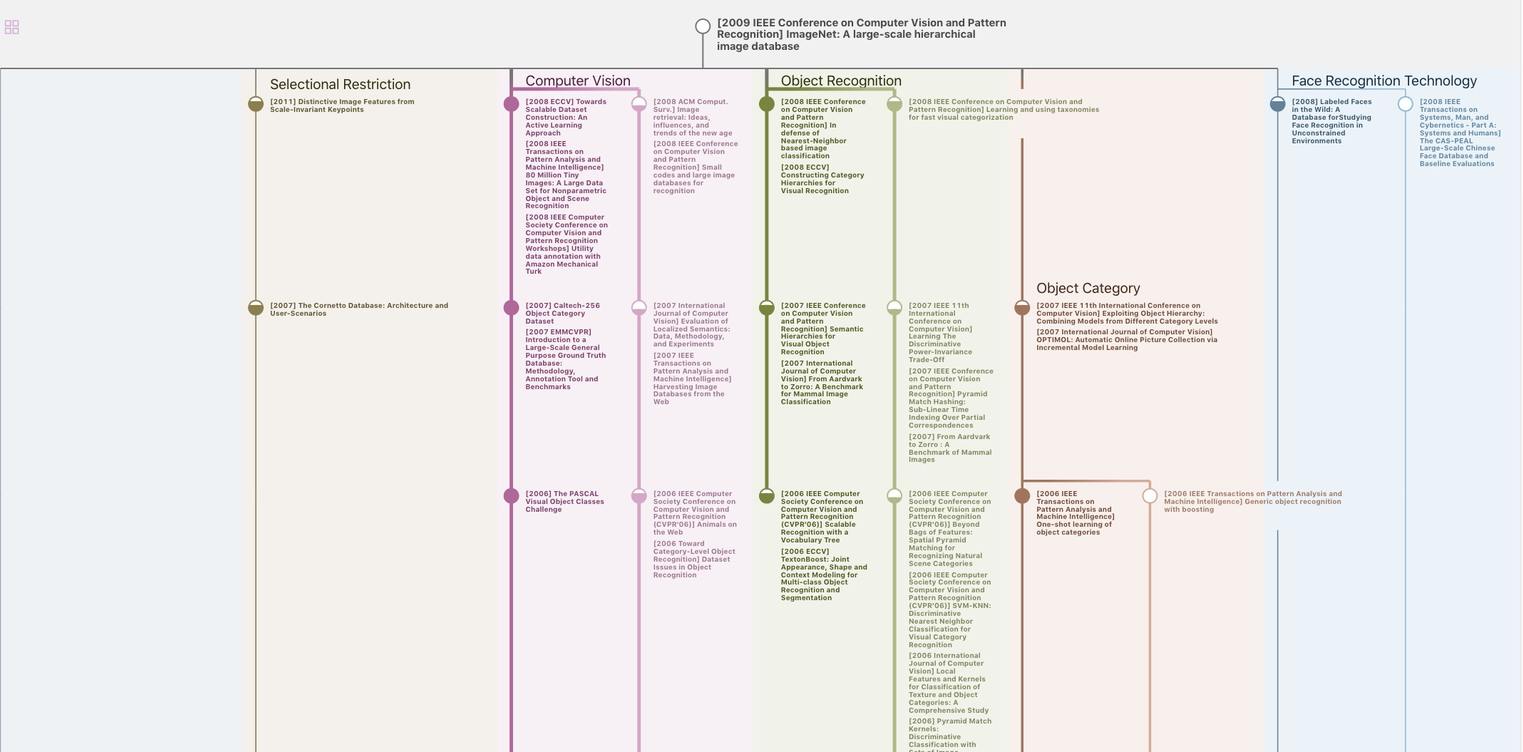
生成溯源树,研究论文发展脉络
Chat Paper
正在生成论文摘要