Towards Efficient Federated Learning: Layer-Wise Pruning-Quantization Scheme and Coding Design
Entropy (Basel, Switzerland)(2023)
摘要
As a promising distributed learning paradigm, federated learning (FL) faces the challenge of communication-computation bottlenecks in practical deployments. In this work, we mainly focus on the pruning, quantization, and coding of FL. By adopting a layer-wise operation, we propose an explicit and universal scheme: FedLP-Q (federated learning with layer-wise pruning-quantization). Pruning strategies for homogeneity/heterogeneity scenarios, the stochastic quantization rule, and the corresponding coding scheme were developed. Both theoretical and experimental evaluations suggest that FedLP-Q improves the system efficiency of communication and computation with controllable performance degradation. The key novelty of FedLP-Q is that it serves as a joint pruning-quantization FL framework with layer-wise processing and can easily be applied in practical FL systems.
更多查看译文
关键词
federated learning,model pruning,parameter quantization,code design,layer-wise aggregation,communication-computation efficiency
AI 理解论文
溯源树
样例
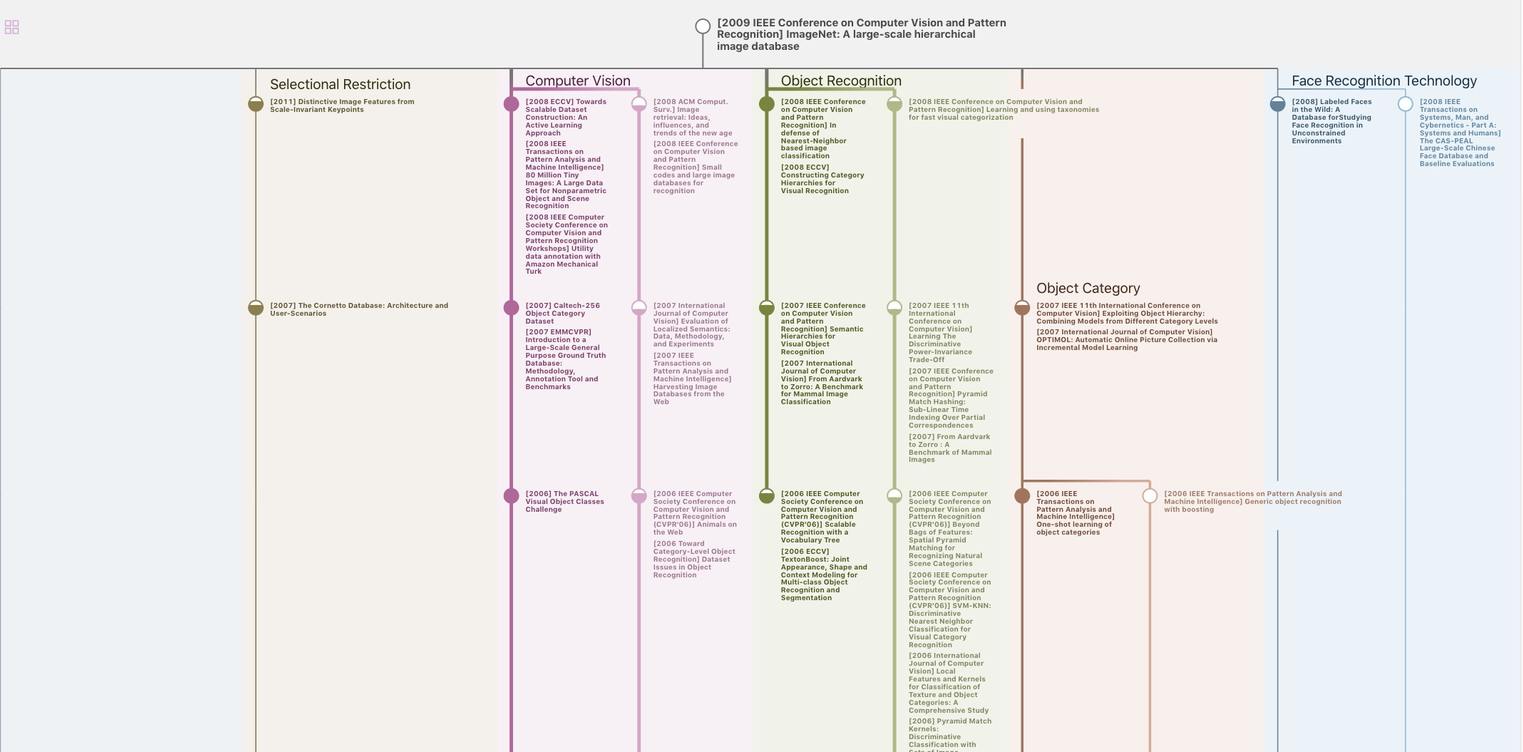
生成溯源树,研究论文发展脉络
Chat Paper
正在生成论文摘要