Deep Reinforcement Learning for Energy Efficiency Maximization in Cache-Enabled Cell-Free Massive MIMO Networks: Single- and Multi-Agent Approaches
IEEE TRANSACTIONS ON VEHICULAR TECHNOLOGY(2023)
摘要
Cell-free massive multiple-input multiple-output (CF-mMIMO) is an emerging beyond fifth-generation (5G) technology that improves energy efficiency (EE) and removes cell structure limitation by using multiple access points (APs). This study investigates the EE maximization problem. Forming proper cooperation clusters is crucial when optimizing EE, and it is often done by selecting AP-user pairs with good channel quality or aligning AP cache contents with user requests. However, the result can be suboptimal if we determine the clusters based solely on either aspect. This motivates our joint design of user association and content caching. Without knowing the user content preferences in advance, two deep reinforcement learning (DRL) approaches, i.e., single-agent reinforcement learning (SARL) and multi-agent reinforcement learning (MARL), are proposed for different scenarios. The SARL approach operates in a centralized manner which has lower computational requirements on edge devices. The MARL approach requires more computation resources at the edge devices but enables parallel computing to reduce the computation time and therefore scales better than the SARL approach. The numerical analysis shows that the proposed approaches outperformed benchmark algorithms in terms of network EE in a small network. In a large network, the MARL yielded the best EE performance and its computation time was reduced significantly by parallel computing.
更多查看译文
关键词
Cell-free massive multiple-input multiple-output,user association,edge caching,deep reinforcement learning,multi-agent reinforcement learning
AI 理解论文
溯源树
样例
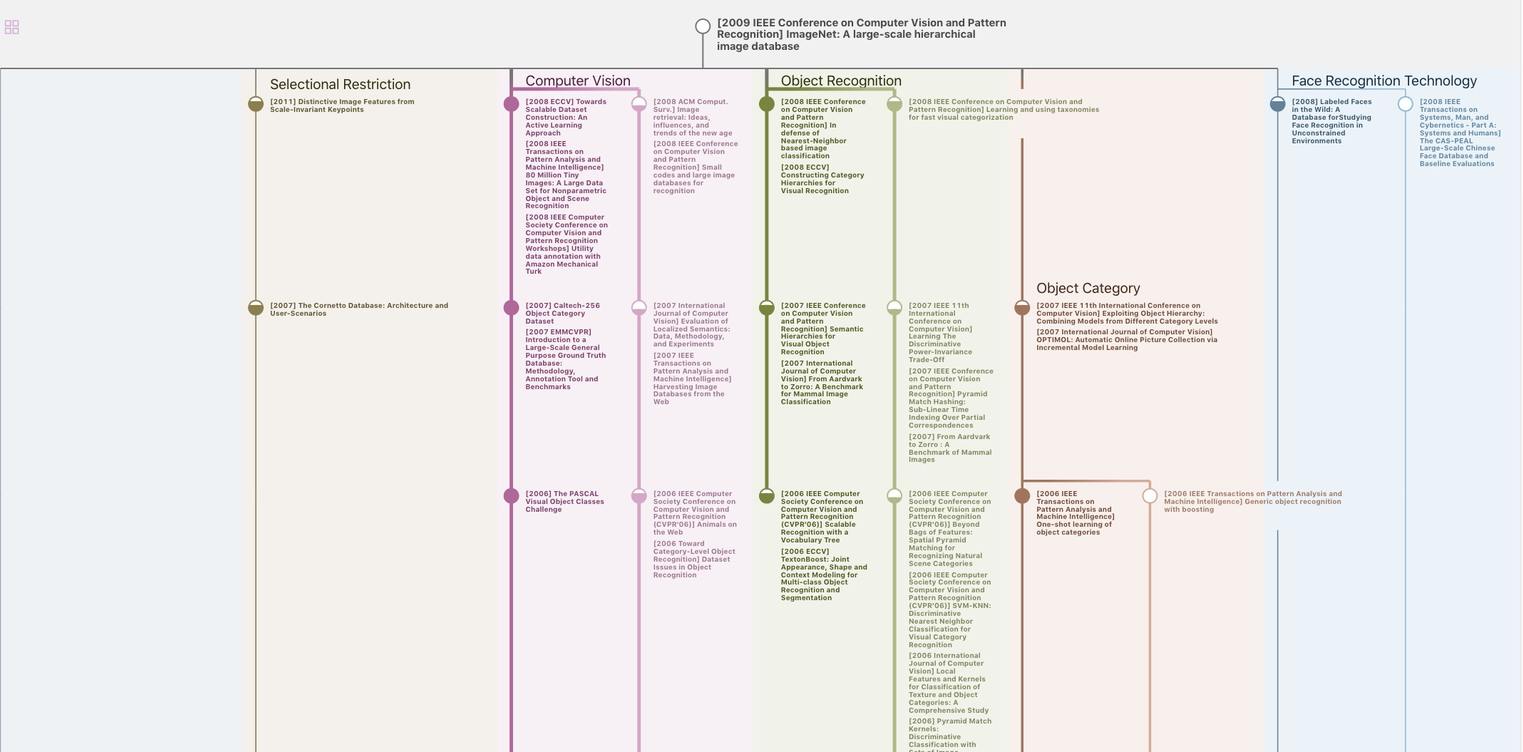
生成溯源树,研究论文发展脉络
Chat Paper
正在生成论文摘要