HAPPY: A deep learning pipeline for mapping cell-to-tissue graphs across placenta histology whole slide images
bioRxiv (Cold Spring Harbor Laboratory)(2022)
摘要
Abstract Accurate placenta pathology assessment is essential for managing maternal and newborn health, but the placenta’s heterogeneity and temporal variability pose challenges for histology analysis. To address this issue, we developed the ‘Histology Analysis Pipeline.PY’ (HAPPY), a deep learning hierarchical method for quantifying the variability of cells and micro-anatomical tissue structures across placenta histology whole slide images. HAPPY differs from patch-based features or segmentation approaches by following an interpretable biological hierarchy, representing cells and cellular communities within tissues at a single-cell resolution across whole slide images. We present a set of quantitative metrics from healthy term placentas as a baseline for future assessments of placenta health and we show how these metrics deviate in placentas with clinically significant placental infarction. HAPPY’s cell and tissue predictions closely replicate those from independent clinical experts and placental biology literature.
更多查看译文
关键词
placenta histology,deep learning pipeline,cell-to-tissue
AI 理解论文
溯源树
样例
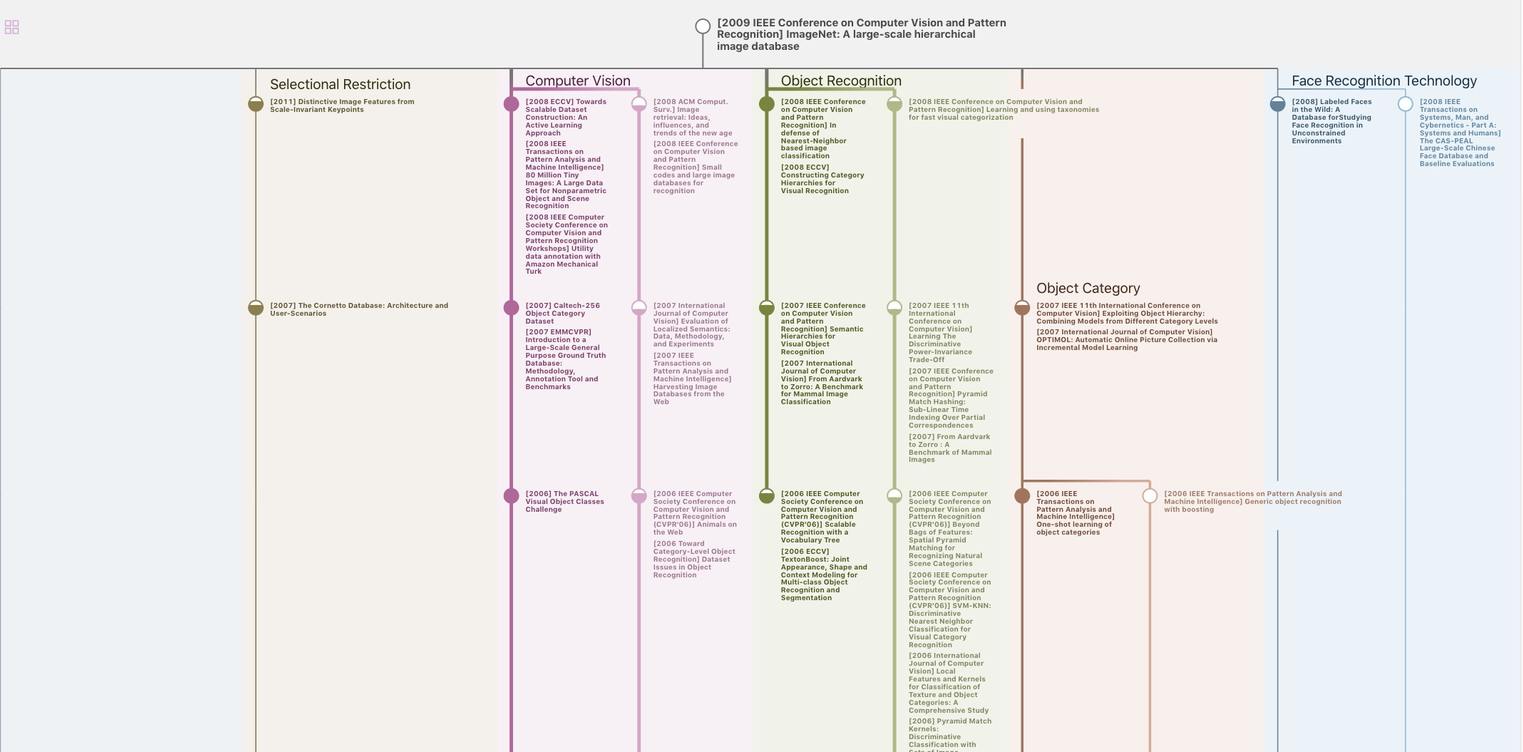
生成溯源树,研究论文发展脉络
Chat Paper
正在生成论文摘要