Lightweight Distributed Gaussian Process Regression for Online Machine Learning
arXiv (Cornell University)(2024)
摘要
In this paper, we study the problem where a group of agents aim to collaboratively learn a common static latent function through streaming data. We propose a lightweight distributed Gaussian process regression (GPR) algorithm that is cognizant of agents' limited capabilities in communication, computation and memory. Each agent independently runs agent-based GPR using local streaming data to predict test points of interest; then the agents collaboratively execute distributed GPR to obtain global predictions over a common sparse set of test points; finally, each agent fuses results from distributed GPR with agent-based GPR to refine its predictions. By quantifying the transient and steady-state performances in predictive variance and error, we show that limited inter-agent communication improves learning performances in the sense of Pareto. Monte Carlo simulation is conducted to evaluate the developed algorithm.
更多查看译文
关键词
gaussian process regression,machine
AI 理解论文
溯源树
样例
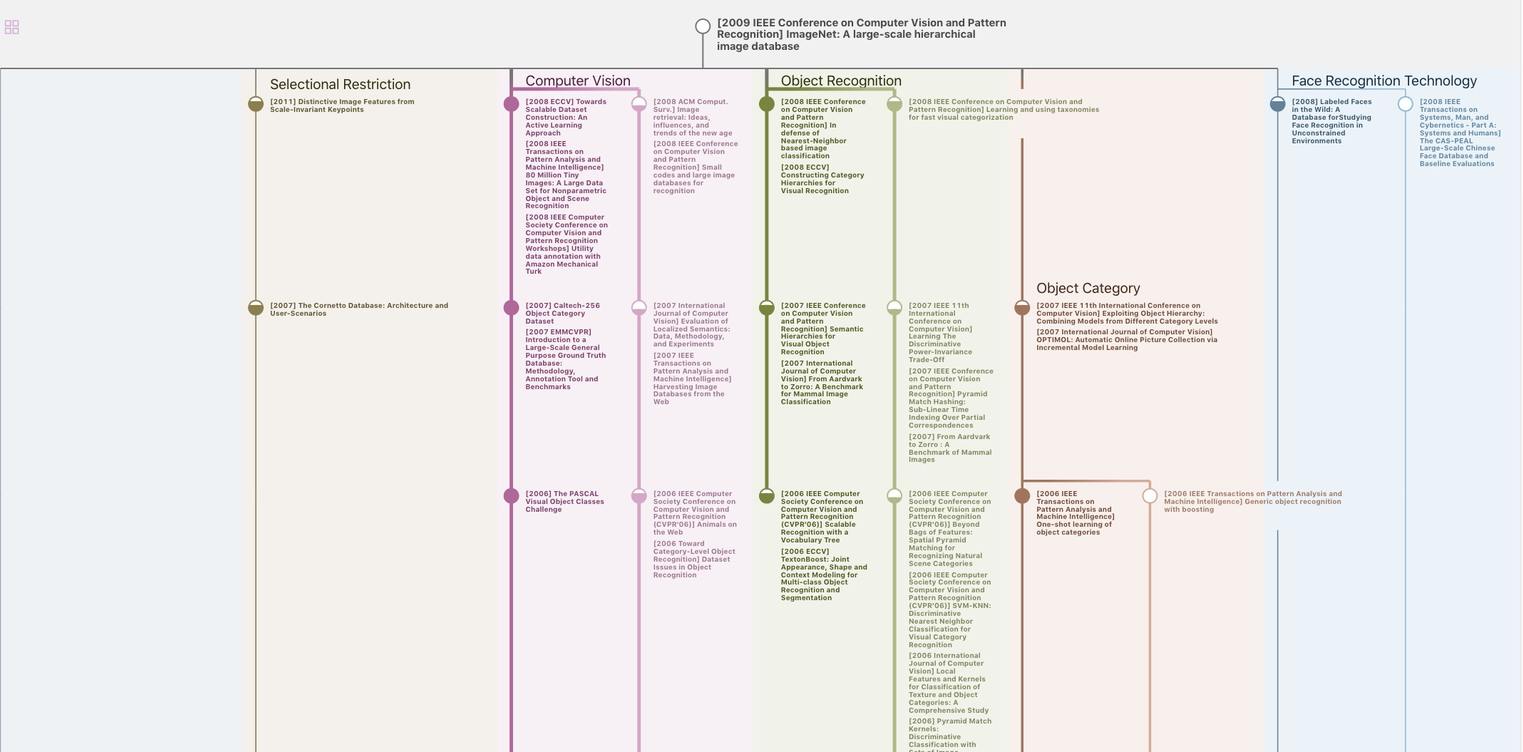
生成溯源树,研究论文发展脉络
Chat Paper
正在生成论文摘要