Coarray Tensor Completion for DOA Estimation
IEEE TRANSACTIONS ON AEROSPACE AND ELECTRONIC SYSTEMS(2023)
摘要
Sparse array direction-of-arrival (DOA) estimation using tensor model has been developed to handle multidimensional sub-Nyquist sampled signals. Furthermore, augmented virtual arrays can be derived for Nyquist-matched coarray tensor processing. However, the partially augmentable sparse array corresponds to a discontinuous virtual array, whereas the existing methods can only utilize its continuous part. Conventional virtual linear array interpolation techniques complete coarray covariance matrices with dispersed missing elements, but fail to complete the coarray tensor with whole missing slices. In this article, we propose a coarray tensor completion algorithm for 2-D DOA estimation, where the coarray tensor statistics can be entirely exploited. In particular, in order to impose an effective low-rank regularization on the slice-missing coarray tensor, we propose shift dimensional augmenting and coarray tensor reshaping approaches to reformulate a structured coarray tensor with sufficiently dispersed missing elements. Furthermore, the shape of the reformulated coarray tensor is optimized by maximizing the dispersion-to-percentage ratio of missing elements. As such, a coarray tensor nuclear norm minimization problem can be designed to optimize the completed coarray tensor corresponding to a filled virtual array, based on which the closed-form DOA estimation is achieved. Meanwhile, the global convergence of the coarray tensor completion is theoretically proved. Simulation results demonstrate the effectiveness of the proposed algorithm compared with other matrix-based and tensor-based methods.
更多查看译文
关键词
Tensors,Estimation,Direction-of-arrival estimation,Array signal processing,Sparse matrices,Transmission line matrix methods,Sensor arrays,Coarray tensor,direction-of-arrival (DOA) estimation,sparse array,tensor completion
AI 理解论文
溯源树
样例
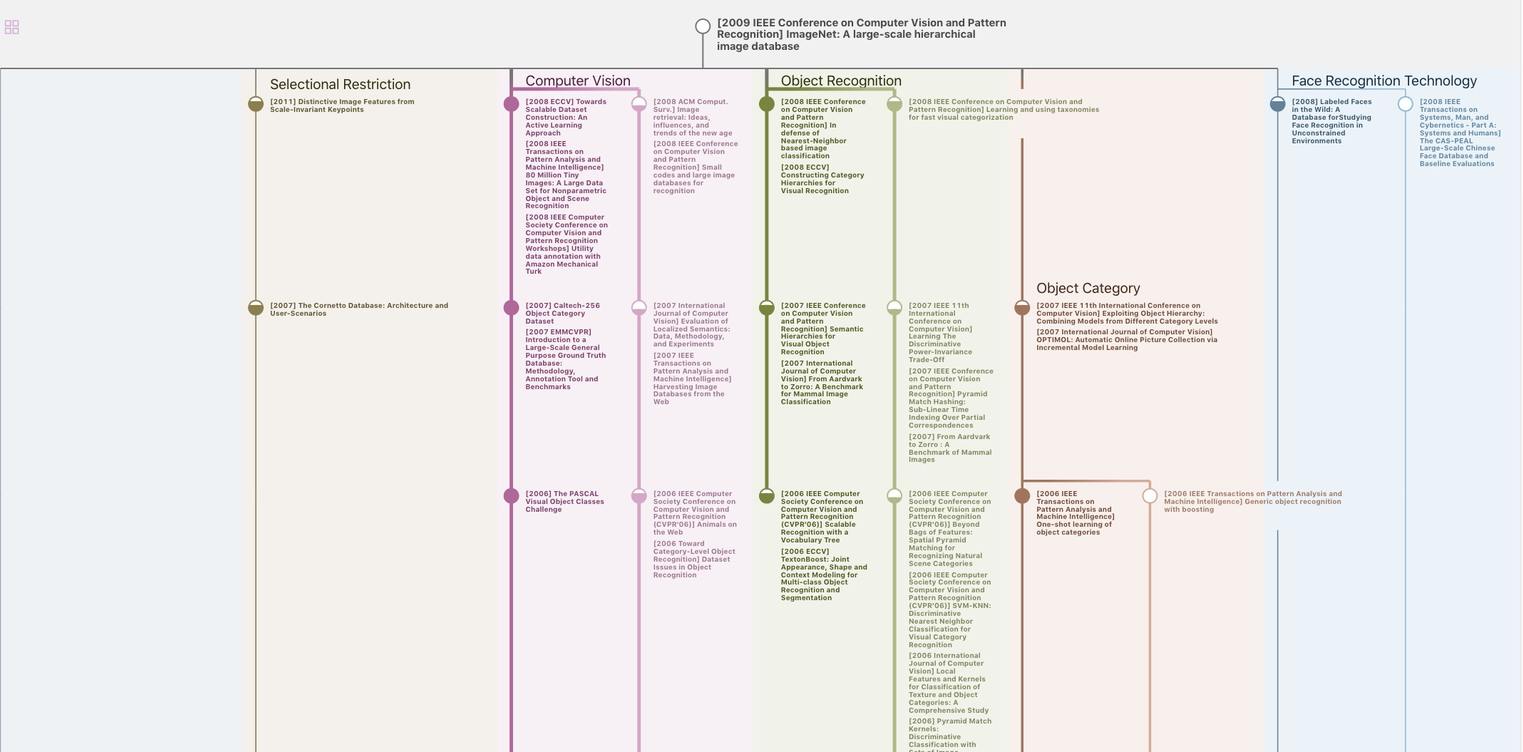
生成溯源树,研究论文发展脉络
Chat Paper
正在生成论文摘要