Comparative analysis of predictive machine learning algorithms for diabetes mellitus
Bulletin of Electrical Engineering and Informatics(2023)
摘要
Diabetes mellitus (DM) is a serious worldwide health issue, and its prevalence is rapidly growing. It is a spectrum of metabolic illnesses defined by perpetually increased blood glucose levels. Undiagnosed diabetes can lead to a variety of problems, including retinopathy, nephropathy, neuropathy, and other vascular abnormalities. In this context, machine learning (ML) technologies may be particularly useful for early disease identification, diagnosis, and therapy monitoring. The core idea of this study is to identify the strong ML algorithm to predict it. For this several ML algorithms were chosen i.e., support vector machine (SVM), Naïve Bayes (NB), K nearest neighbor (KNN), random forest (RF), logistic regression (LR), and decision tree (DT), according to studied work. Two, Pima Indian diabetic (PID) and Germany diabetes datasets were used and the experiment was performed using Waikato environment for knowledge analysis (WEKA) 3.8.6 tool. This article discussed about performance matrices and error rates of classifiers for both datasets. The results showed that for PID database (PIDD), SVM works better with an accuracy of 74% whereas for Germany KNN and RF work better with 98.7% accuracy. This study can aid healthcare facilities and researchers in comprehending the value and application of ML algorithms in predicting diabetes at an early stage.
更多查看译文
关键词
predictive machine,diabetes mellitus,machine learning,algorithms
AI 理解论文
溯源树
样例
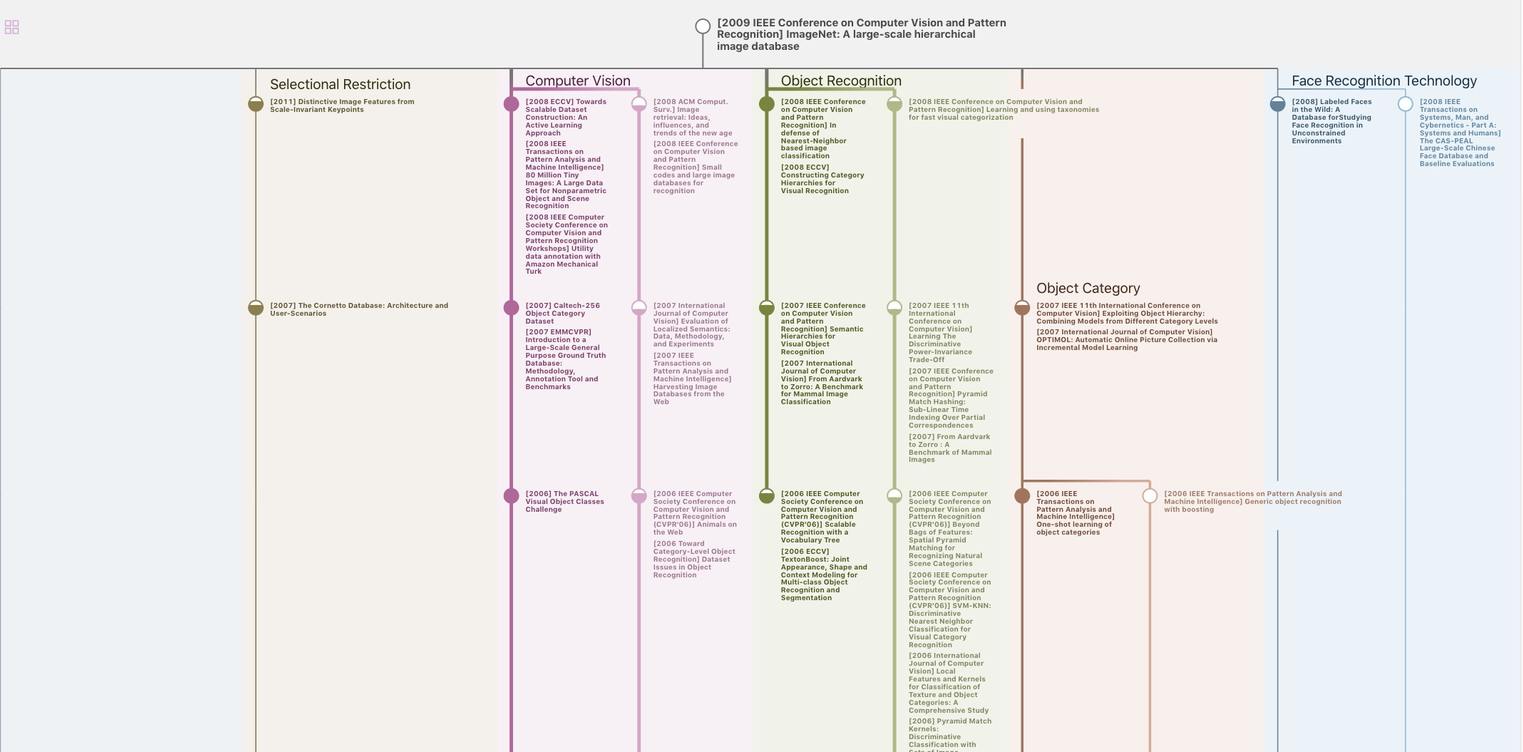
生成溯源树,研究论文发展脉络
Chat Paper
正在生成论文摘要