Causal determinants of postoperative length of stay in cardiac surgery using causal graphical learning
The Journal of Thoracic and Cardiovascular Surgery(2023)
摘要
Objective We aimed to learn the causal determinants of postoperative length of stay in cardiac surgery patients undergoing isolated coronary artery bypass grafting or aortic valve replacement surgery. Methods For patients undergoing isolated coronary artery bypass grafting or isolated aortic valve replacement surgeries between 2011 and 2016, we used causal graphical modeling on electronic health record data. The Fast Causal Inference (FCI) algorithm from the Tetrad software was used on data to estimate a Partial Ancestral Graph (PAG) depicting direct and indirect causes of postoperative length of stay, given background clinical knowledge. Then, we used the latent variable intervention-calculus when the directed acyclic graph is absent (LV-IDA) algorithm to estimate strengths of causal effects of interest. Finally, we ran a linear regression for postoperative length of stay to contrast statistical associations with what was learned by our causal analysis. Results In our cohort of 2610 patients, the mean postoperative length of stay was 219 hours compared with the Society of Thoracic Surgeons 2016 national mean postoperative length of stay of approximately 168 hours. Most variables that clinicians believe to be predictors of postoperative length of stay were found to be causes, but some were direct (eg, age, diabetes, hematocrit, total operating time, and postoperative complications), and others were indirect (including gender, race, and operating surgeon). The strongest average causal effects on postoperative length of stay were exhibited by preoperative dialysis (209 hours); neuro-, pulmonary-, and infection-related postoperative complications (315 hours, 89 hours, and 131 hours, respectively); reintubation (61 hours); extubation in operating room (–47 hours); and total operating room duration (48 hours). Linear regression coefficients diverged from causal effects in magnitude (eg, dialysis) and direction (eg, crossclamp time). Conclusions By using retrospective electronic health record data and background clinical knowledge, causal graphical modeling retrieved direct and indirect causes of postoperative length of stay and their relative strengths. These insights will be useful in designing clinical protocols and targeting improvements in patient management.
更多查看译文
关键词
causal graphical learning,cardiac surgery,postoperative length
AI 理解论文
溯源树
样例
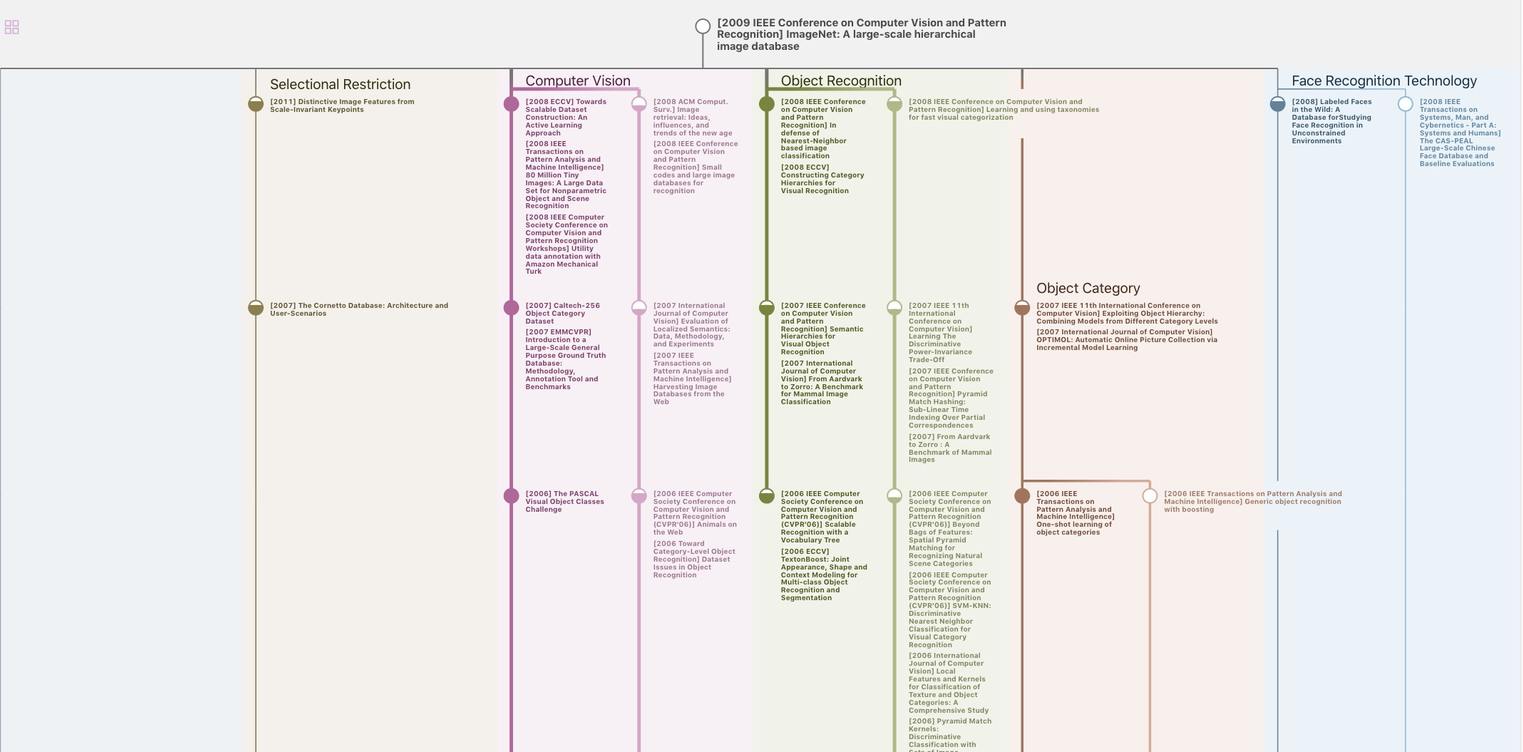
生成溯源树,研究论文发展脉络
Chat Paper
正在生成论文摘要